A differential privacy framework for matrix factorization recommender systems
User Modeling and User-Adapted Interaction(2016)
摘要
Recommender systems rely on personal information about user behavior for the recommendation generation purposes. Thus, they inherently have the potential to hamper user privacy and disclose sensitive information. Several works studied how neighborhood-based recommendation methods can incorporate user privacy protection. However, privacy preserving latent factor models, in particular, those represented by matrix factorization techniques, the state-of-the-art in recommender systems, have received little attention. In this paper, we address the problem of privacy preserving matrix factorization by utilizing differential privacy, a rigorous and provable approach to privacy in statistical databases. We propose a generic framework and evaluate several ways, in which differential privacy can be applied to matrix factorization. By doing so, we specifically address the privacy-accuracy trade-off offered by each of the algorithms. We show that, of all the algorithms considered, input perturbation results in the best recommendation accuracy, while guaranteeing a solid level of privacy protection against attacks that aim to gain knowledge about either specific user ratings or even the existence of these ratings. Our analysis additionally highlights the system aspects that should be addressed when applying differential privacy in practice, and when considering potential privacy preserving solutions.
更多查看译文
关键词
Recommender System,Matrix Factorization,Stochastic Gradient Descent,Alternate Little Square,Differential Privacy
AI 理解论文
溯源树
样例
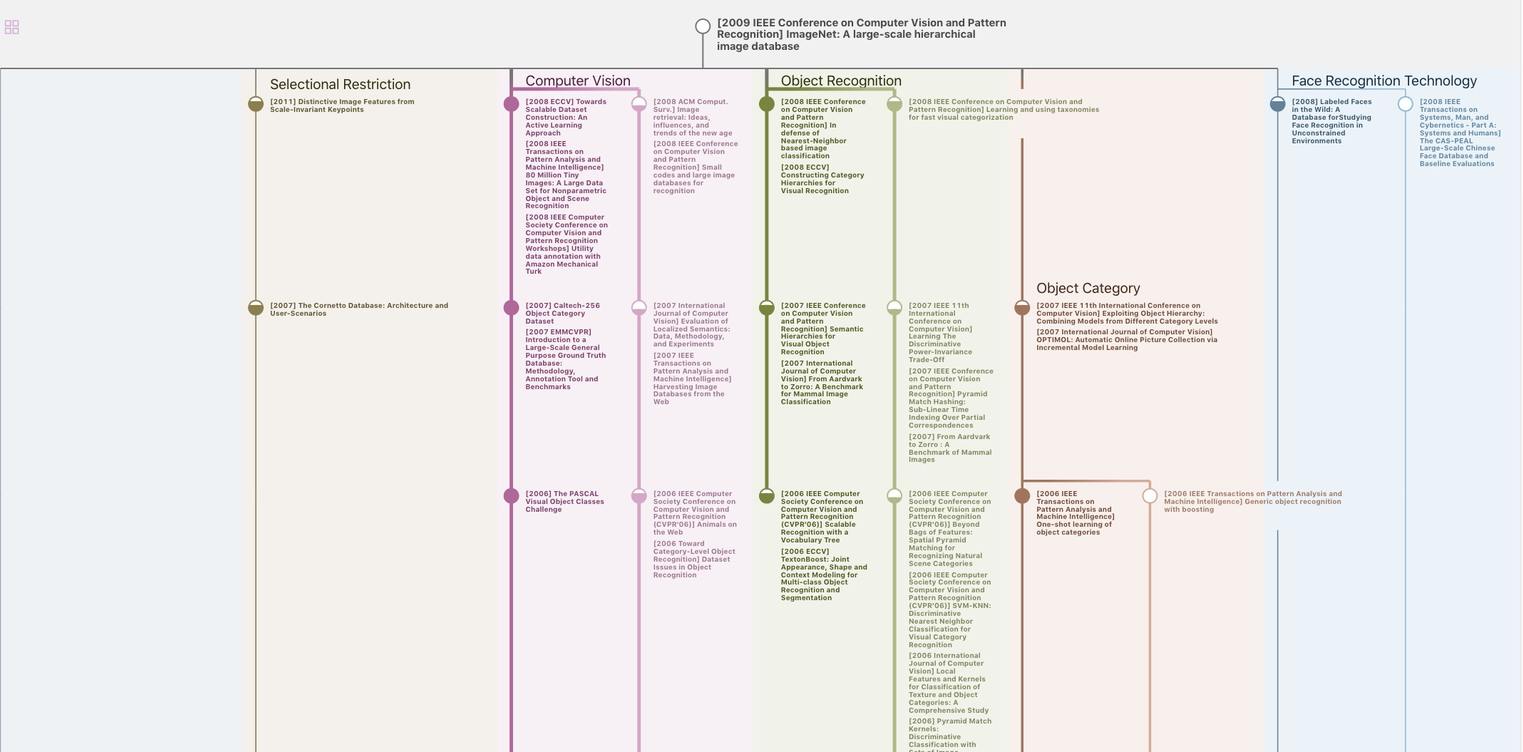
生成溯源树,研究论文发展脉络
Chat Paper
正在生成论文摘要