Robust Constrained Learning-based NMPC enabling reliable mobile robot path tracking.
I. J. Robotics Res.(2016)
摘要
This paper presents a Robust Constrained Learning-based Nonlinear Model Predictive Control RC-LB-NMPC algorithm for path-tracking in off-road terrain. For mobile robots, constraints may represent solid obstacles or localization limits. As a result, constraint satisfaction is required for safety. Constraint satisfaction is typically guaranteed through the use of accurate, a priori models or robust control. However, accurate models are generally not available for off-road operation. Furthermore, robust controllers are often conservative, since model uncertainty is not updated online. In this work our goal is to use learning to generate low-uncertainty, non-parametric models in situ. Based on these models, the predictive controller computes both linear and angular velocities in real-time, such that the robot drives at or near its capabilities while respecting path and localization constraints. Localization for the controller is provided by an on-board, vision-based mapping and navigation system enabling operation in large-scale, off-road environments. The paper presents experimental results, including over 5 km of travel by a 900 kg skid-steered robot at speeds of up to 2.0 m/s. The result is a robust, learning controller that provides safe, conservative control during initial trials when model uncertainty is high and converges to high-performance, optimal control during later trials when model uncertainty is reduced with experience.
更多查看译文
关键词
Model predictive control,Gaussian process,robust control
AI 理解论文
溯源树
样例
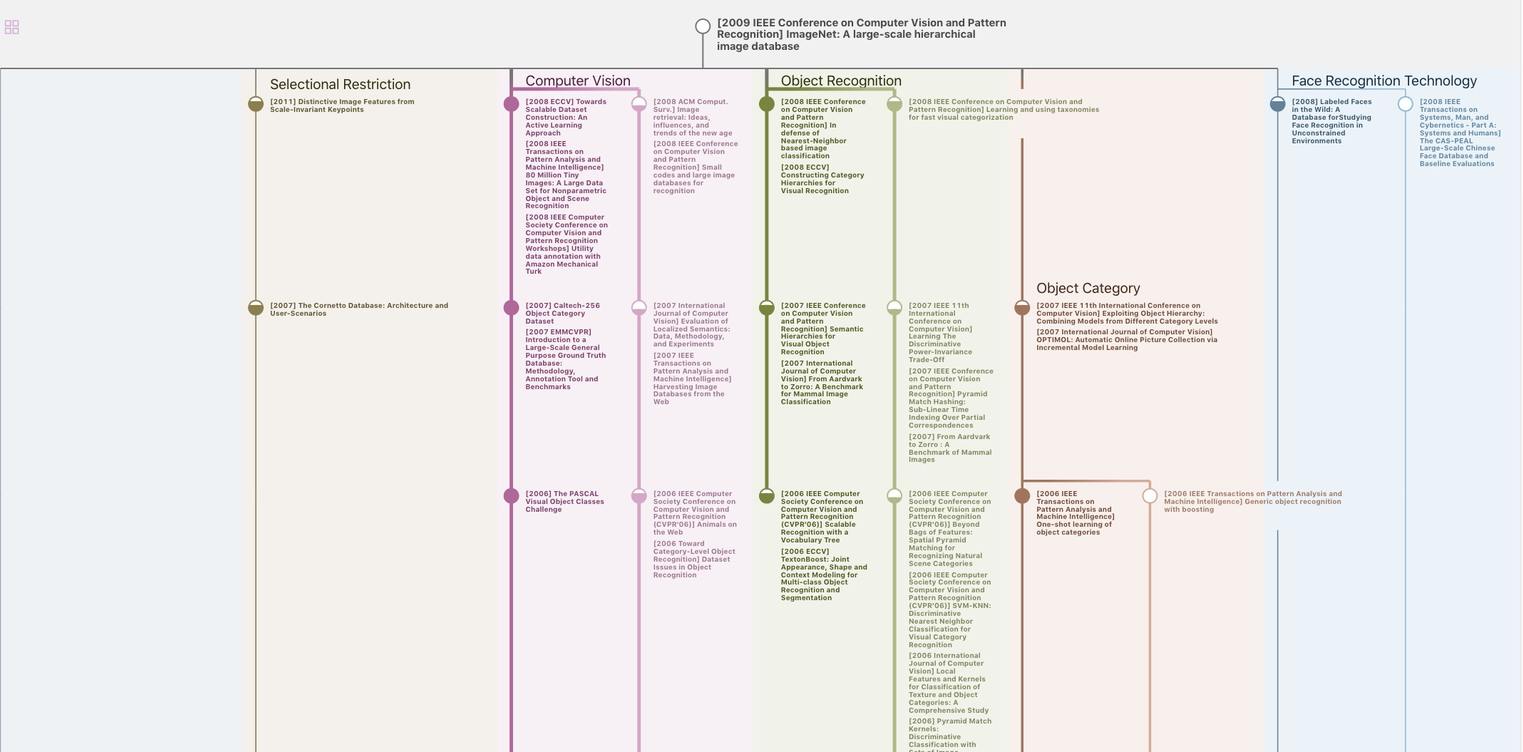
生成溯源树,研究论文发展脉络
Chat Paper
正在生成论文摘要