Automated Session-Quality Assessment for Human Tutoring Based on Expert Ratings of Tutoring Success.
EDM(2015)
摘要
Archived transcripts from tens of millions of online human tutoring sessions potentially contain important knowledge about how online tutors help, or fail to help, students learn. However, without ways of automatically analyzing these large corpora, any knowledge in this data will remain buried. One way to approach this issue is to train an estimator for the learning effectiveness of an online tutoring interaction. While significant work has been done on automated assessment of student responses and artifacts (e.g., essays), automated assessment has not traditionally automated assessments of human-to-human tutoring sessions. In this work, we trained a model for estimating tutoring session quality based on a corpus of 1438 online tutoring sessions rated by expert tutors. Each session was rated for evidence of learning (outcomes) and educational soundness (process). Session features for this model included dialog act classifications, mode classifications (e.g., Scaffolding), statistically distinctive subsequences of such classifications, dialog initiative (e.g., statements by tutor vs. student), and session length. The model correlated more highly with evidence of learning than educational soundness ratings, in part due to the greater difficulty of classifying tutoring modes. This model was then applied to a corpus of 242k online tutoring sessions, to examine the relationships between automated assessments and other available metadata (e.g., the tutor’s self-assessment). On this large corpus, the automated assessments followed similar patterns as the expert rater’s assessments, but with lower overall correlation strength. Based on the analyses presented, the assessment model for online tutoring sessions emulates the ratings of expert human tutors for session quality ratings with a reasonable degree of accuracy.
更多查看译文
AI 理解论文
溯源树
样例
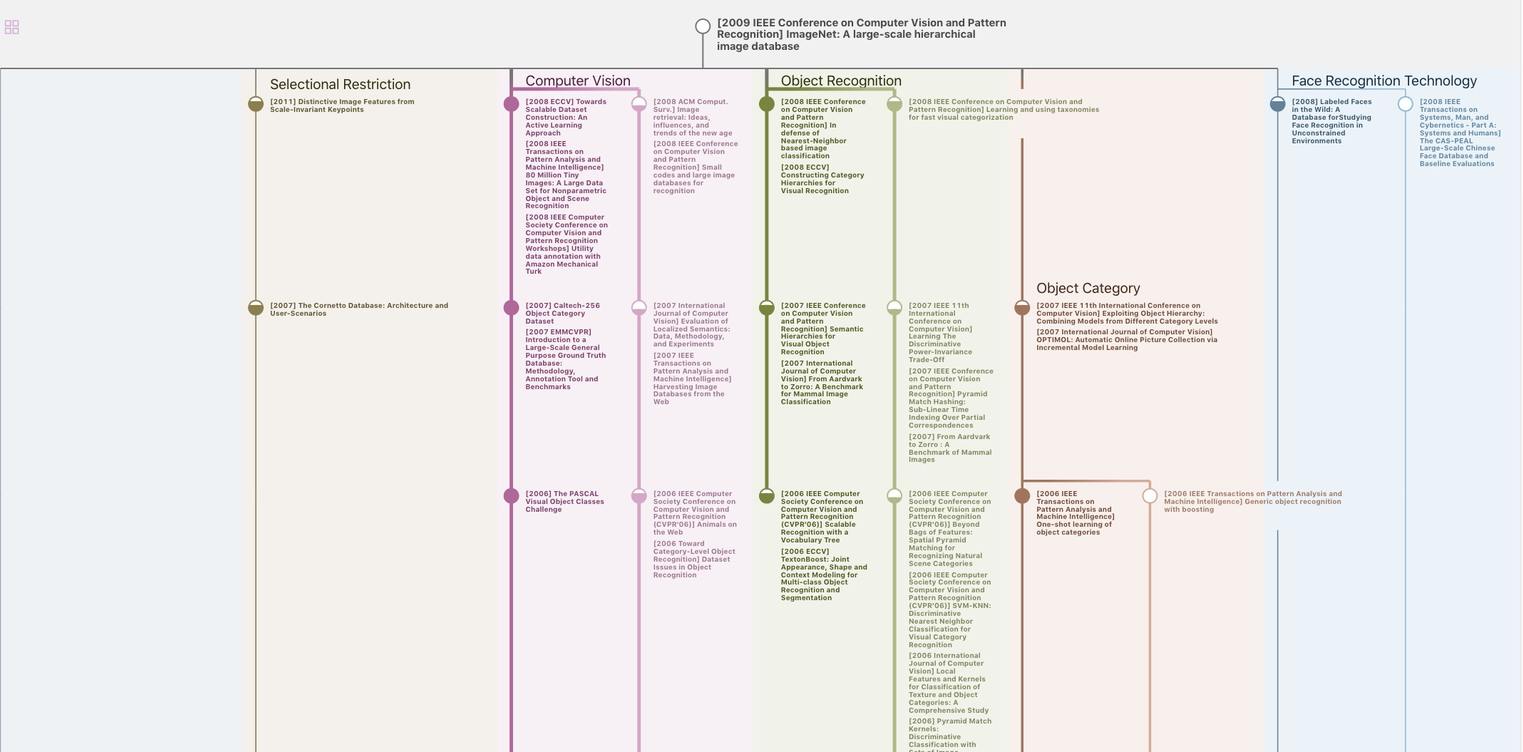
生成溯源树,研究论文发展脉络
Chat Paper
正在生成论文摘要