Towards a Synthetic Data Generator for Matching Decision Trees.
ICEIS(2016)
摘要
It is popular to use real-world data to evaluate or teach data mining techniques. However, there are some disadvantages to use real-world data for such purposes. Firstly, real-world data in most domains is difficult to obtain for several reasons, such as budget, technical or ethical. Secondly, the use of many of the real-world data is restricted or in the case of data mining, those data sets do either not contain specific patterns that are easy to mine for teaching purposes or the data needs special preparation and the algorithm needs very specific settings in order to find patterns in it. The solution to this could be the generation of synthetic, âmeaningful dataâ (data with intrinsic patterns). This paper presents a framework for such a data generator, which is able to generate datasets with intrinsic patterns, such as decision trees. A preliminary run of the prototype proves that the generation of such âmeaningful dataâ is possible. Also the proposed approach could be extended to a further development for generating synthetic data with other intrinsic patterns.
更多查看译文
关键词
Synthetic, Data Generator, Data Mining, Decision Trees, Classification, Pattern
AI 理解论文
溯源树
样例
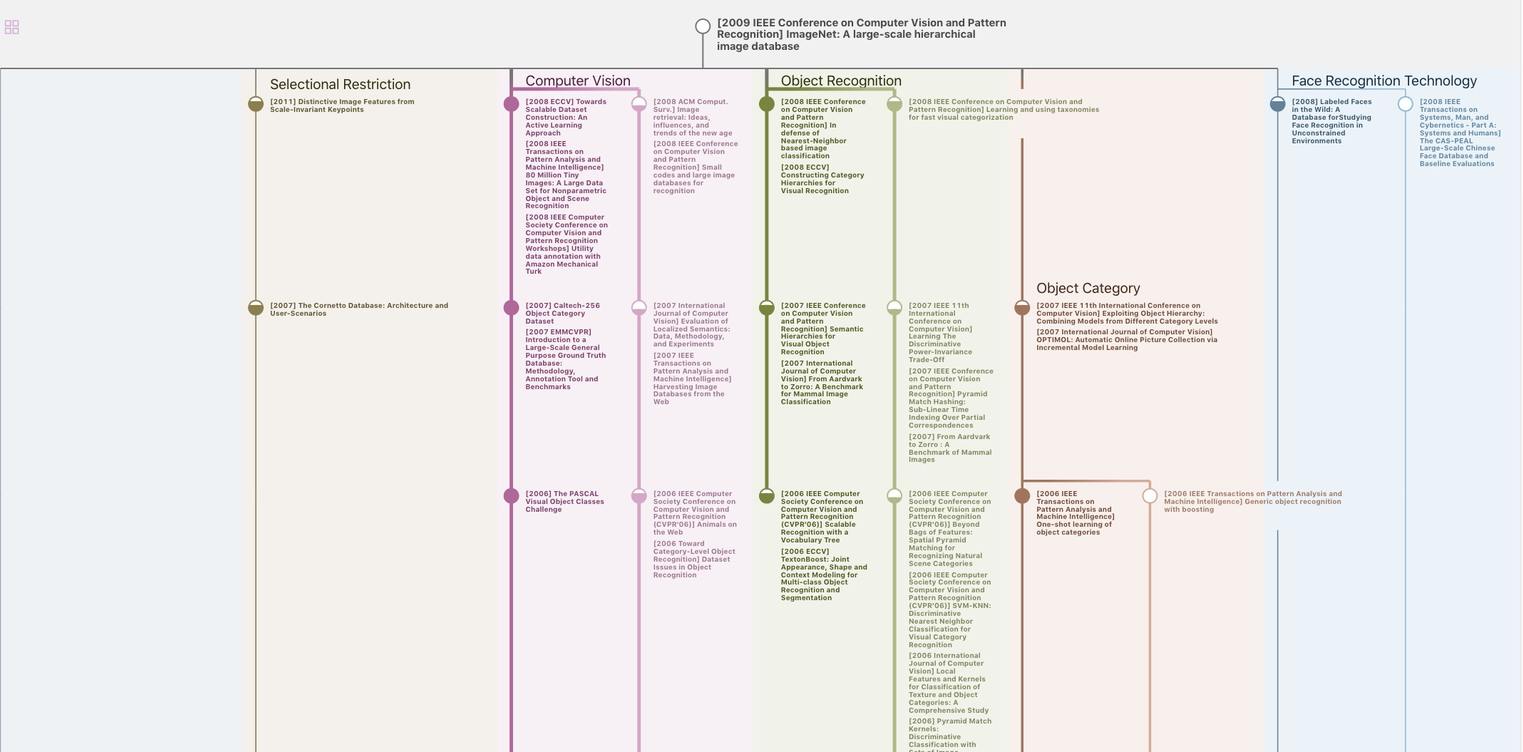
生成溯源树,研究论文发展脉络
Chat Paper
正在生成论文摘要