SPOTHOT: Scalable Detection of Geo-spatial Events in Large Textual Streams.
SSDBM(2016)
摘要
The analysis of social media data poses several challenges: first of all, the data sets are very large, secondly they change constantly, and third they are heterogeneous, consisting of text, images, geographic locations and social connections. In this article, we focus on detecting events consisting of text and location information, and introduce an analysis method that is scalable both with respect to volume and velocity. We also address the problems arising from differences in adoption of social media across cultures, languages, and countries in our event detection by efficient normalization. We introduce an algorithm capable of processing vast amounts of data using a scalable online approach based on the SigniTrend event detection system, which is able to identify unusual geo-textual patterns in the data stream without requiring the user to specify any constraints in advance, such as hashtags to track: In contrast to earlier work, we are able to monitor every word at every location with just a fixed amount of memory, compare the values to statistics from earlier data and immediately report significant deviations with minimal delay. Thus, this algorithm is capable of reporting Breaking News in real-time. Location is modeled using unsupervised geometric discretization and supervised administrative hierarchies, which permits detecting events at city, regional, and global levels at the same time. The usefulness of the approach is demonstrated using several real-world example use cases using Twitter data.
更多查看译文
AI 理解论文
溯源树
样例
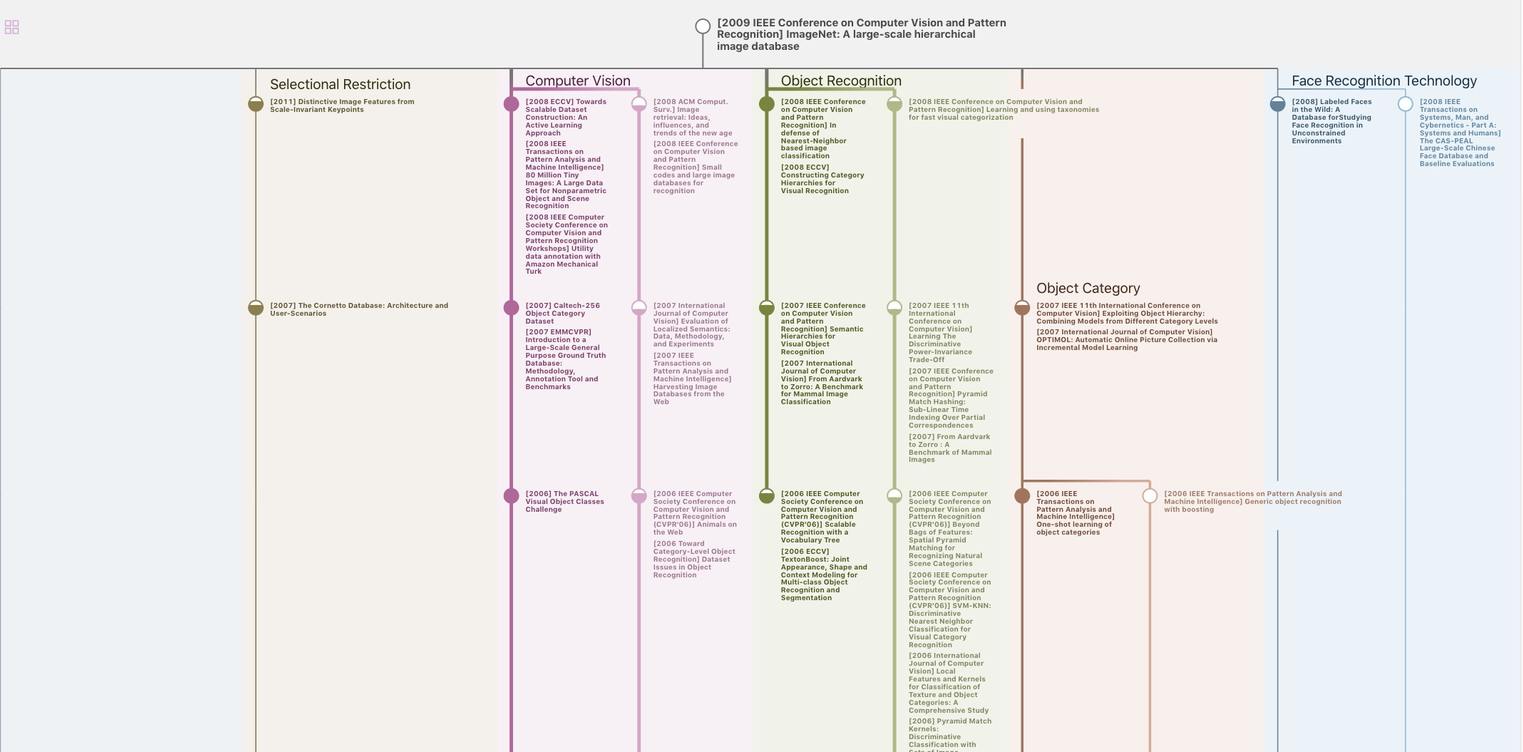
生成溯源树,研究论文发展脉络
Chat Paper
正在生成论文摘要