Wide Dynamic Range Weights And Biologically Realistic Synaptic Dynamics For Spike-Based Learning Circuits
2016 IEEE International Symposium on Circuits and Systems (ISCAS)(2016)
摘要
Spike-based neuromorphic learning circuits typically represent their synaptic weights as voltages, and convert them into post-synaptic currents so that they can be integrated by their afferent silicon neuron. This voltage-to-current conversion is often done using a single transistor. This results in an exponential (for weak-inversion) or quadratic (for strong inversion) non-linear transformation which severely restricts the type of learning algorithms that can be implemented. To overcome this problem we propose a range of solutions that perform a linear transformation from weight voltage to synaptic current, simplifying the implementation of a spike-based learning rules. We demonstrate the application of these conversion circuits using current-mode integrators that produce alpha-functions with biologically realistic temporal dynamics and amplitudes that are linearly proportional to the synaptic weights. The circuits proposed are low-power, and can be integrated in a wide range of spike-based learning frameworks that have been recently proposed. We describe the advantages and disadvantages of the various solutions proposed and validate them with circuit simulation results.
更多查看译文
关键词
wide dynamic range,biologically realistic synaptic dynamics,spike based learning circuits,postsynaptic currents,silicon neuron,voltage-to-current conversion,nonlinear transformation,learning algorithms,biologically realistic temporal dynamics,low power circuits,spike based learning framework
AI 理解论文
溯源树
样例
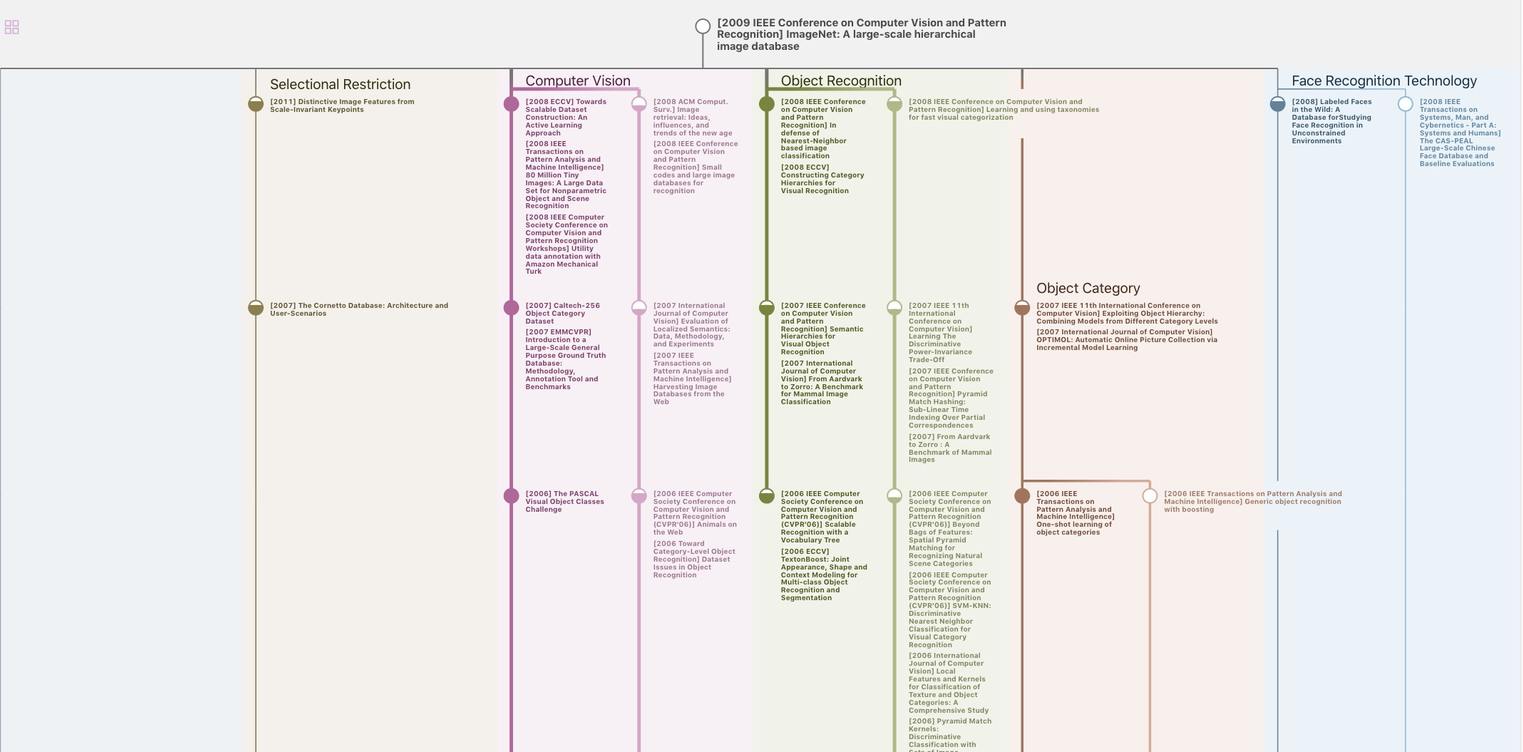
生成溯源树,研究论文发展脉络
Chat Paper
正在生成论文摘要