Learning Deep Neural Network Using Max-Margin Minimum Classification Error
2016 IEEE International Conference on Acoustics, Speech and Signal Processing (ICASSP)(2016)
摘要
Deep neural networks (DNNs) have recently achieved state-of-the-art performance on various tasks, such as image classification, handwriting recognition, text spotting, and speech recognition. Most of these DNNs use softmax regression and cross-entropy loss to calculate the loss function for optimization. However, the loss function is merely expected to raise the output of the true class and reduce others without distinction. In this paper, we propose a new max-margin minimum classification error ((MCE)-C-3) training method, which is inspired by the traditional minimum classification error, but is more appropriate for training DNNs. The proposed M3CE aims not only to increase the posteriori of the true class but also to decrease the output of the most confused class, which can cover any shortage of the cross-entropy loss. We evaluate the M3CE on two popular datasets, MNIST and CIFAR-10. Experimental results show that the (MCE)-C-3 complements cross-entropy efficiently and achieves better performance.
更多查看译文
关键词
Deep neural network,softmax regression,minimum classification error,max-margin
AI 理解论文
溯源树
样例
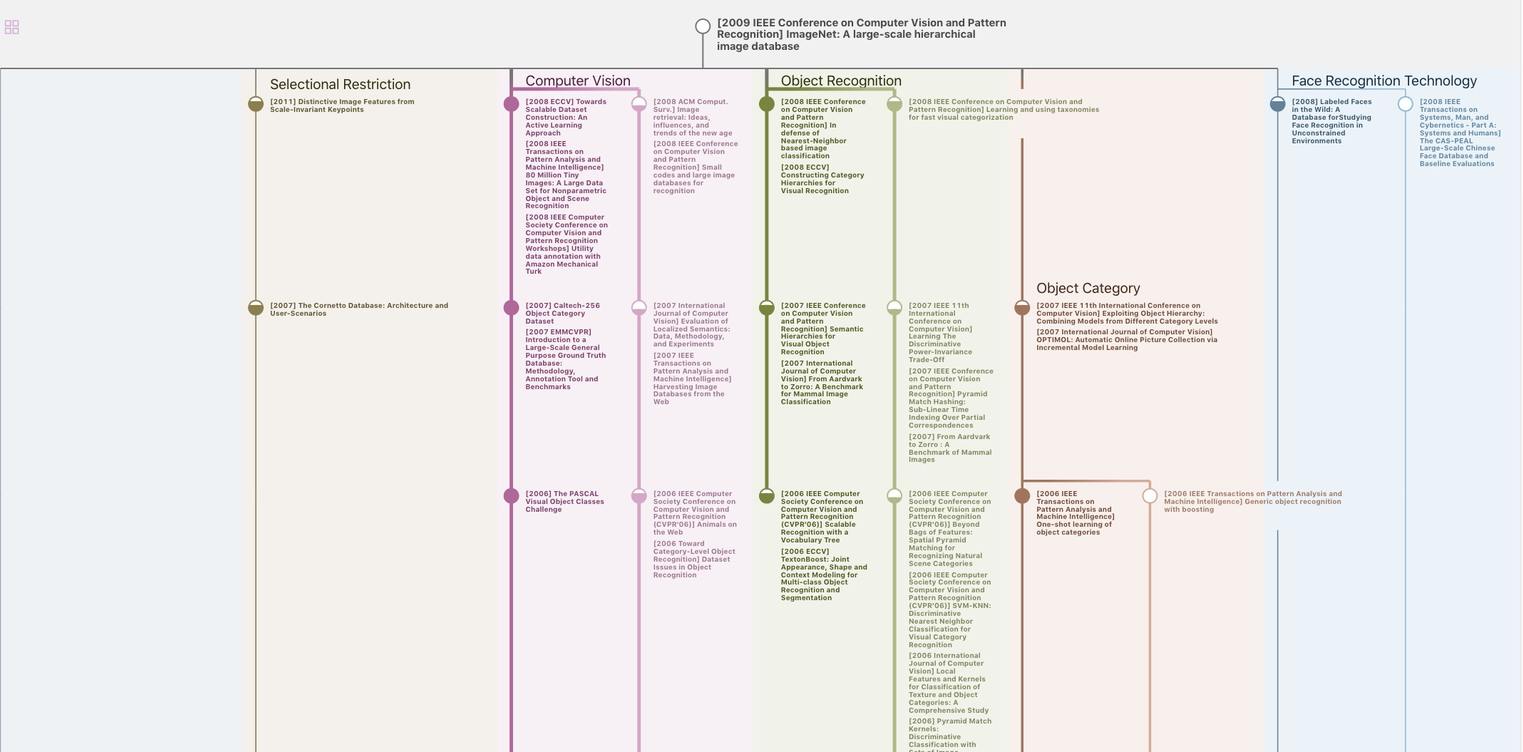
生成溯源树,研究论文发展脉络
Chat Paper
正在生成论文摘要