Parallelism and distribution for very large scale content-based image retrieval. (Parallélisme et distribution pour des bases d'images à très grande échelle).
semanticscholar(2013)
摘要
The scale of multimedia collections has grown very fast over the last few years. Facebook stores more than 100 billion images, 200 million are added every day. In order to cope with this growth, methods for content-based image retrieval must adapt gracefully. The work presented in this thesis goes in this direction. Two observations drove the design of the high-dimensional indexing technique presented here. Firstly, the collections are so huge, typically several terabytes, that they must be kept on secondary storage. Addressing disk related issues is thus central to our work. Secondly, all CPUs are now multi-core and clusters of machines are a commonplace. Parallelism and distribution are both key for fast indexing and high-throughput batch-oriented searching. We describe in this manuscript a high-dimensional indexing technique called eCP. Its design includes the constraints associated to using disks, parallelism and distribution. At its core is an non-iterative unstructured vectorial quantization scheme. eCP builds on an existing indexing scheme that is main memory oriented. Our rst contribution is a set of extensions for processing very large data collections, reducing indexing costs and best using disks. The second contribution proposes multi-threaded algorithms for both building and searching, harnessing the power of multi-core processors. Datasets for evaluation contain about 25 million images or over 8 billion SIFT descriptors. The third contribution addresses distributed computing. We adapt eCP to the MapReduce programming model and use the Hadoop framework and HDFS for our experiments. This time we evaluate eCP's ability to scale-up with a collection of 100 million images, more than 30 billion SIFT descriptors, and its ability to scale-out by running experiments on more than 100 machines.
更多查看译文
AI 理解论文
溯源树
样例
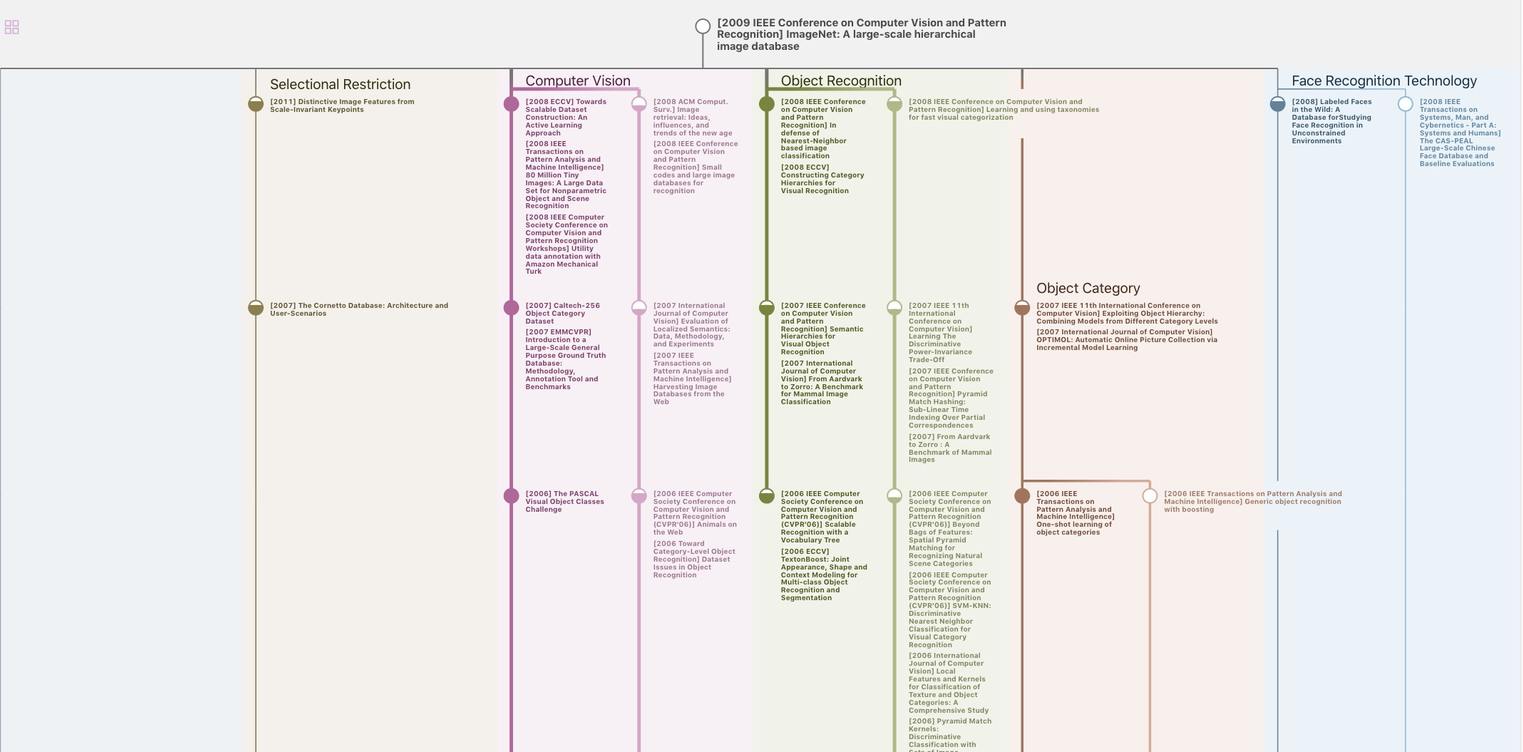
生成溯源树,研究论文发展脉络
Chat Paper
正在生成论文摘要