Bounding and Minimizing Counterfactual Error.
arXiv: Machine Learning(2016)
摘要
There is intense interest in applying machine learning methods to problems of causal inference which arise in applications such as healthcare, economic policy, and education. In this paper we use the counterfactual inference approach to causal inference, and propose new theoretical results and new algorithms for performing counterfactual inference. Building on an idea recently proposed by Johansson et al., our results and methods rely on learning so-called balanced representations: representations that are similar between the factual and counterfactual distributions. We give a novel, simple and intuitive bound, showing that the expected counterfactual error of a representation is bounded by a sum of the factual error of that representation and the distance between the factual and counterfactual distributions induced by the representation. We use Integral Probability Metrics to measure distances between distributions, and focus on two special cases: the Wasserstein distance and the Maximum Mean Discrepancy (MMD) distance. Our bound leads directly to new algorithms, which are simpler and easier to employ compared to those suggested in Johansson et al.. Experiments on real and simulated data show the new algorithms match or outperform state-of-the-art methods.
更多查看译文
AI 理解论文
溯源树
样例
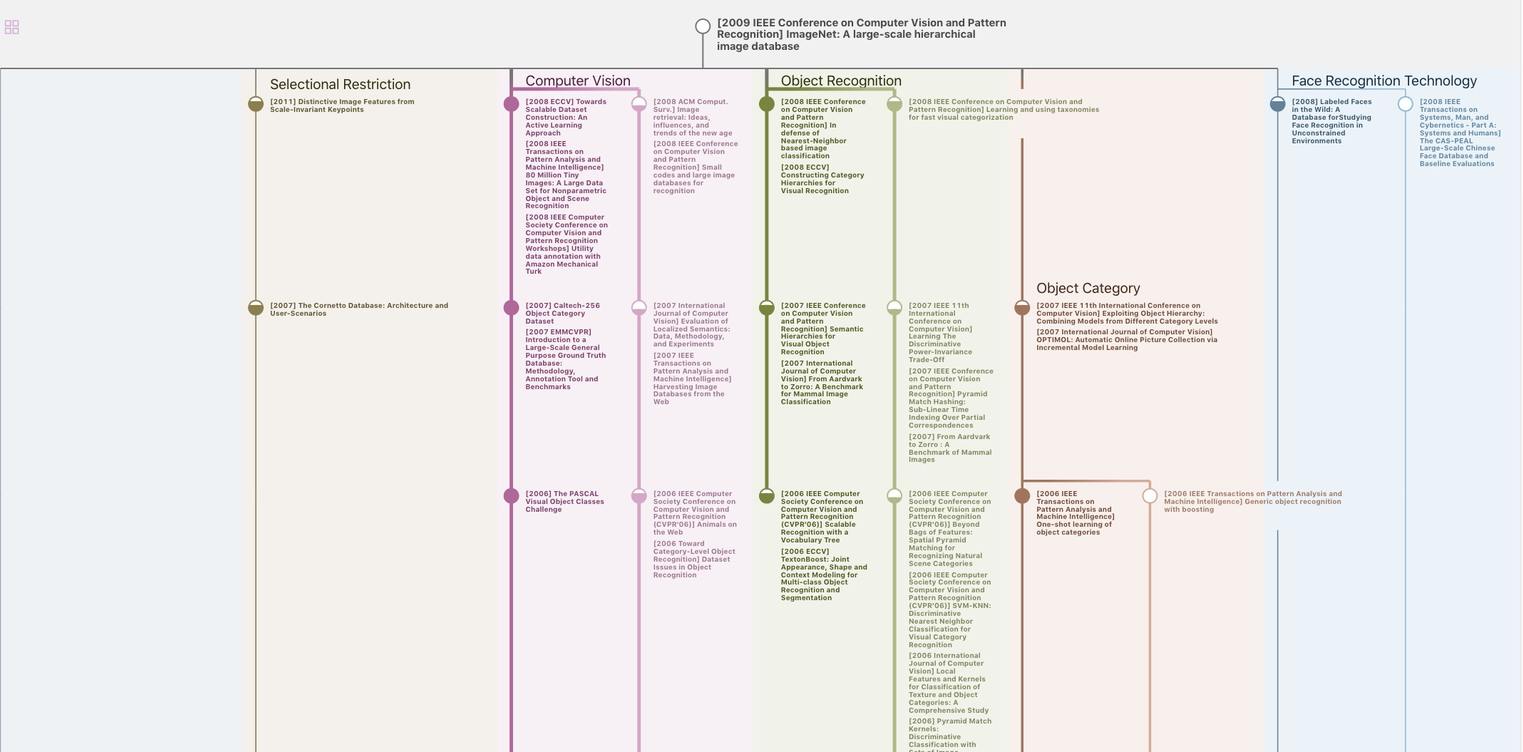
生成溯源树,研究论文发展脉络
Chat Paper
正在生成论文摘要