Hyperspectral Local Intrinsic Dimensionality.
IEEE Trans. Geoscience and Remote Sensing(2016)
摘要
The intrinsic dimensionality (ID) of multivariate data is a very important concept in spectral unmixing of hyperspectral images. A good estimation of the ID is crucial for a correct retrieval of the number of endmembers (the spectral signatures of macroscopic materials) in the image, for dimensionality reduction or for subspace learning, among others. Recently, some approaches to perform spectral unmixing and superresolution locally have been proposed, which require a local estimation of the number of endmembers to use. However, the role of ID in local regions of hyperspectral images has not been properly addressed. Some important issues when dealing with small regions of hyperspectral data can seriously affect the performance of conventional hyperspectral ID estimators. We show that three factors mainly affect local ID estimation: the number of pixels in the local regions, which has to be high enough for the estimations to be relevant, the number of hyperspectral bands which complicates the estimations if the ambient space has a high dimensionality, and the noise, which can be misinterpreted as a signal when its power is important. Here, we review the hyperspectral ID estimators on the literature for local ID estimation, we show how they behave in a local setting on synthetic and real data sets, and we provide some guidelines to make proper use of these estimators in local approaches.
更多查看译文
关键词
Hyperspectral imagery,intrinsic dimensionality (ID),local spectral unmixing,virtual dimensionality
AI 理解论文
溯源树
样例
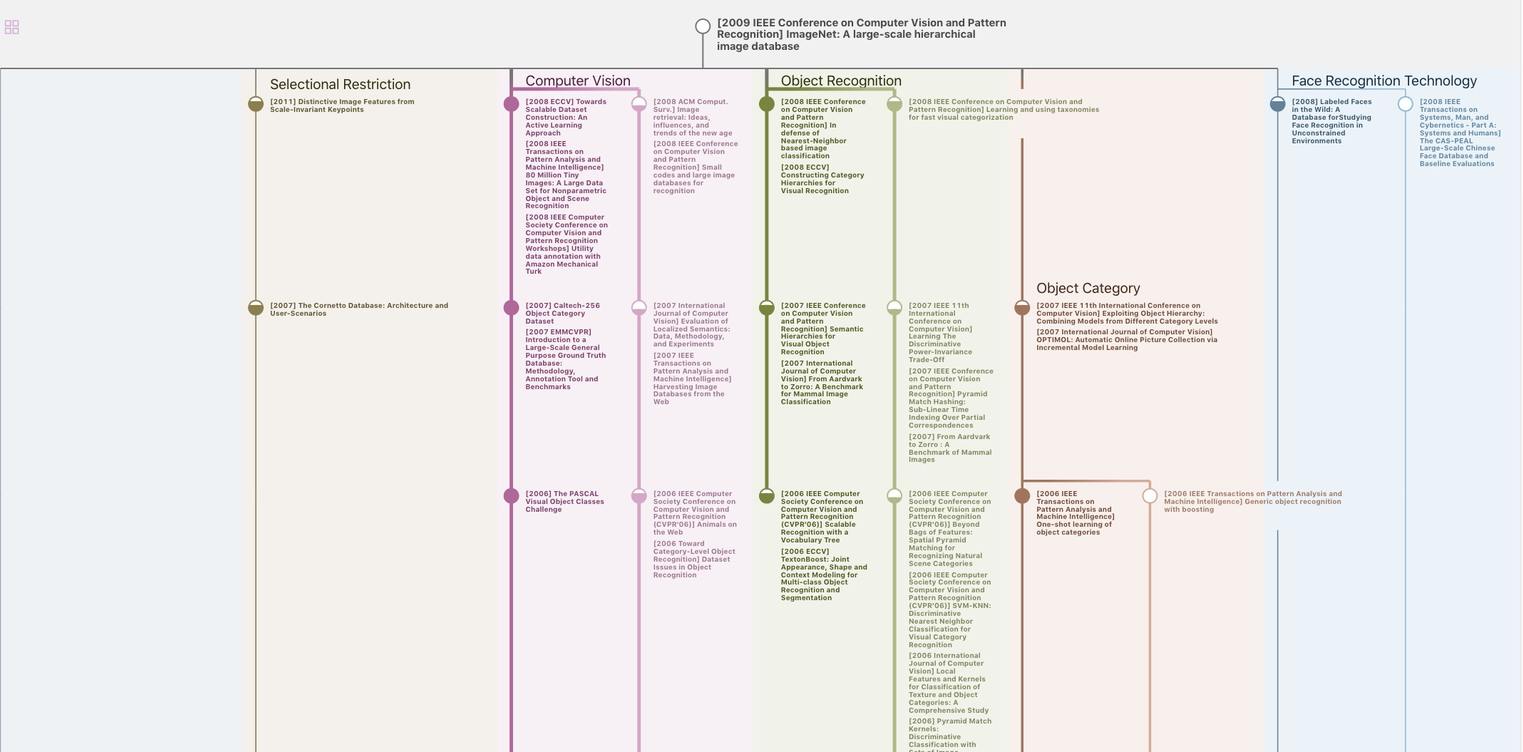
生成溯源树,研究论文发展脉络
Chat Paper
正在生成论文摘要