Quantiles over data streams: experimental comparisons, new analyses, and further improvements
The VLDB Journal(2016)
摘要
A fundamental problem in data management and analysis is to generate descriptions of the distribution of data. It is most common to give such descriptions in terms of the cumulative distribution, which is characterized by the quantiles of the data. The design and engineering of efficient methods to find these quantiles has attracted much study, especially in the case where the data are given incrementally, and we must compute the quantiles in an online, streaming fashion. While such algorithms have proved to be extremely useful in practice, there has been limited formal comparison of the competing methods, and no comprehensive study of their performance. In this paper, we remedy this deficit by providing a taxonomy of different methods and describe efficient implementations. In doing so, we propose new variants that have not been studied before, yet which outperform existing methods. To illustrate this, we provide detailed experimental comparisons demonstrating the trade-offs between space, time, and accuracy for quantile computation.
更多查看译文
关键词
Data stream algorithms,Quantiles,Ordinary least squares
AI 理解论文
溯源树
样例
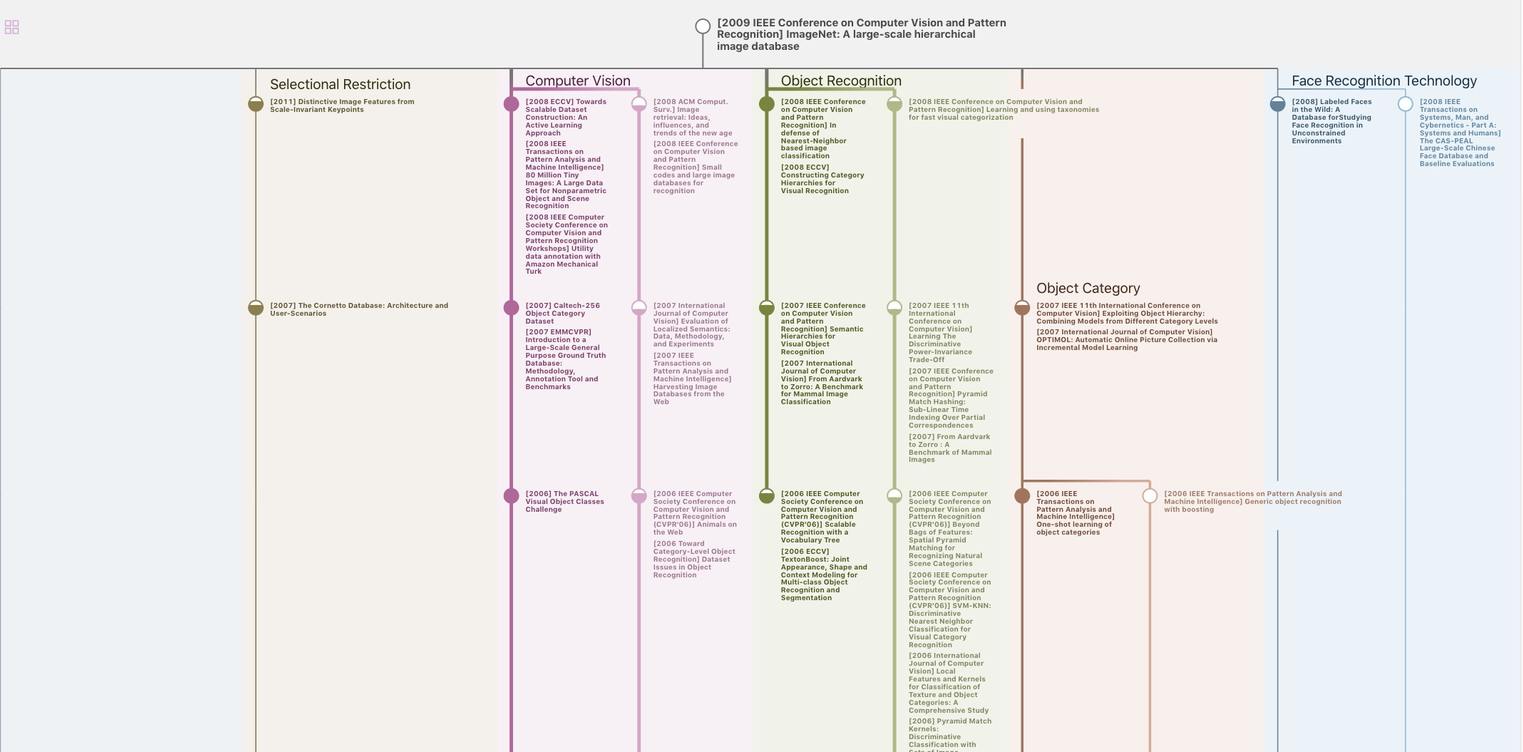
生成溯源树,研究论文发展脉络
Chat Paper
正在生成论文摘要