Extended local binary patterns for face recognition.
Inf. Sci.(2016)
摘要
A set of six LBP-like features derived from local intensities and differences.A labeled dominant pattern scheme is proposed to learn salient information.Utilizing whitened PCA to produce more compact, robust and discriminative features.Fused WPCA features improves the accuracy and robustness of face recognition.Proposed face recognition system is highly robust to illumination variations. This paper presents a simple and novel, yet highly effective approach for robust face recognition. Using LBP-like descriptors based on local accumulated pixel differences - Angular Differences and Radial Differences, the local differences were decomposed into complementary components of signs and magnitudes. Based on these descriptors we developed labeled dominant patterns where the most frequently occurring patterns and their labels were learned to capture discriminative textural information. Six histogram features were obtained from each given face image by concatenating spatial histograms extracted from non-overlapping subregions. A whitened PCA technique was used for dimensionality reduction to produce more compact, robust and discriminative features, which were then fused using the nearest neighbor classifier, with Euclidean distance as the similarity measure.We evaluated the effectiveness of the proposed method on the Extended Yale B, the large-scale FERET, and CAS-PEAL-R1 databases, and found that that the proposed method impressively outperforms other well-known systems with a recognition rate of 74.6% on the CAS-PEAL-R1 lighting probe set.
更多查看译文
关键词
Face recognition,Feature extraction,Local binary pattern,Local descriptors
AI 理解论文
溯源树
样例
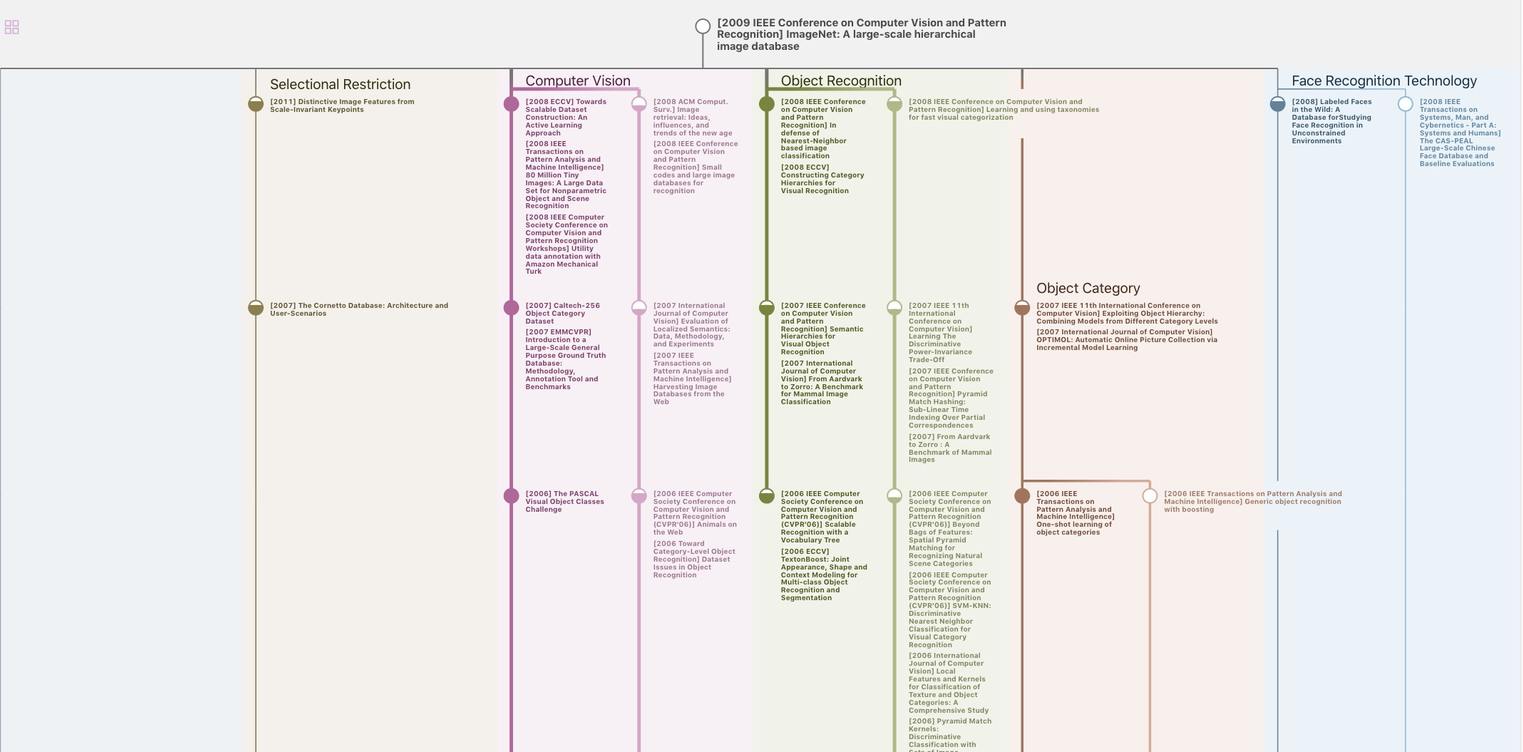
生成溯源树,研究论文发展脉络
Chat Paper
正在生成论文摘要