Scan $B$-Statistic for Kernel Change-Point Detection
Annual Conference on Neural Information Processing Systems(2019)
摘要
Detecting the emergence of an abrupt change-point is a classic problem in statistics and machine learning. Kernel-based nonparametric statistics have been used for this task, which enjoys fewer assumptions on the distributions than the parametric approach and can handle high-dimensional data. In this article, we focus on the scenario when the amount of background data is large and propose a computationally efficient kernel-based statistics for change-point detection, inspired by the recently developed B-statistics. A novel theoretical result of the article is the characterization of the tail probability of these statistics using the change-of-measure technique, which focuses on characterizing the tail of the detection statistics rather than obtaining its asymptotic distribution under the null distribution. Such approximations are crucial to controlling the false alarm rate, which corresponds to the average run length in online change-point detection. Our approximations are shown to be highly accurate. Thus, they provide a convenient way to find detection thresholds for online cases without the need to resort to the more expensive simulations. We show that our methods perform well on both synthetic data and real data.
更多查看译文
关键词
Change-point detection,false-alarm control,kernel-based statistics,online algorithm
AI 理解论文
溯源树
样例
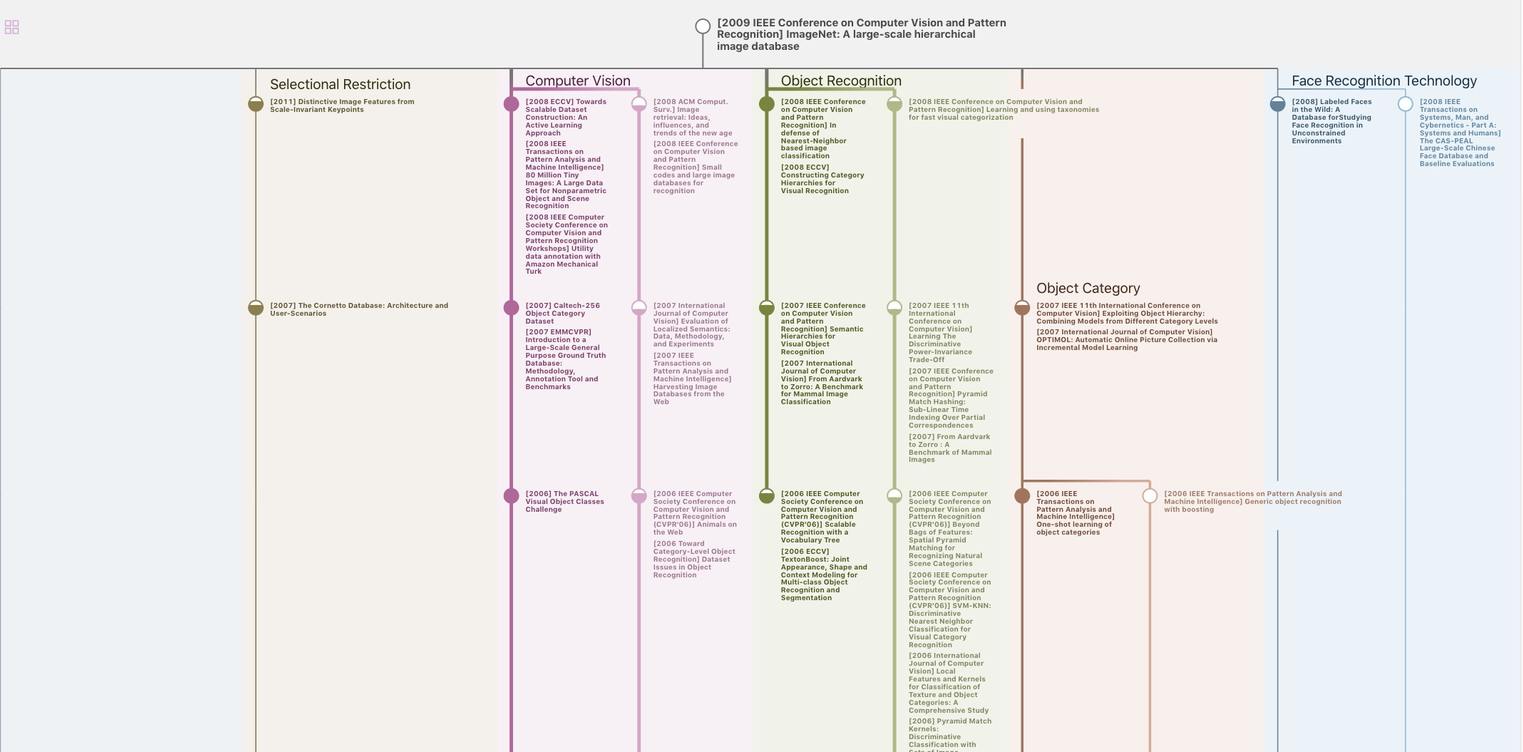
生成溯源树,研究论文发展脉络
Chat Paper
正在生成论文摘要