Nearly Tight Oblivious Subspace Embeddings by Trace Inequalities.
SODA '16: Symposium on Discrete Algorithms Arlington Virginia January, 2016(2016)
摘要
We present a new analysis of sparse oblivious subspace embeddings, based on the "matrix Chernoff" technique. These are probability distributions over (relatively) sparse matrices such that for any d-dimensional subspace of Rn, the norms of all vectors in the subspace are simultaneously approximately preserved by the embedding with high probability--typically with parameters depending on d but not on n. The families of embedding matrices considered here are essentially the same as those in [NN13], but with better parameters (sparsity and embedding dimension). Because of this, this analysis essentially serves as a "drop-in replacement" for Nelson-Nguyen's, improving bounds on its many applications to problems such as as least squares regression and low-rank approximation.
This new method is based on elementary tail bounds combined with matrix trace inequalities (Golden-Thompson or Lieb's theorem), and does not require combinatorics, unlike the Nelson-Nguyen approach. There are also variants of this method that are even simpler, at the cost of worse parameters. Furthermore, the bounds obtained are much tighter than previous ones, matching known lower bounds up to a single log(d) factor in embedding dimension (previous results had more log factors and also had suboptimal tradeoffs with sparsity).
更多查看译文
关键词
tight oblivious subspace embeddings,trace inequalities
AI 理解论文
溯源树
样例
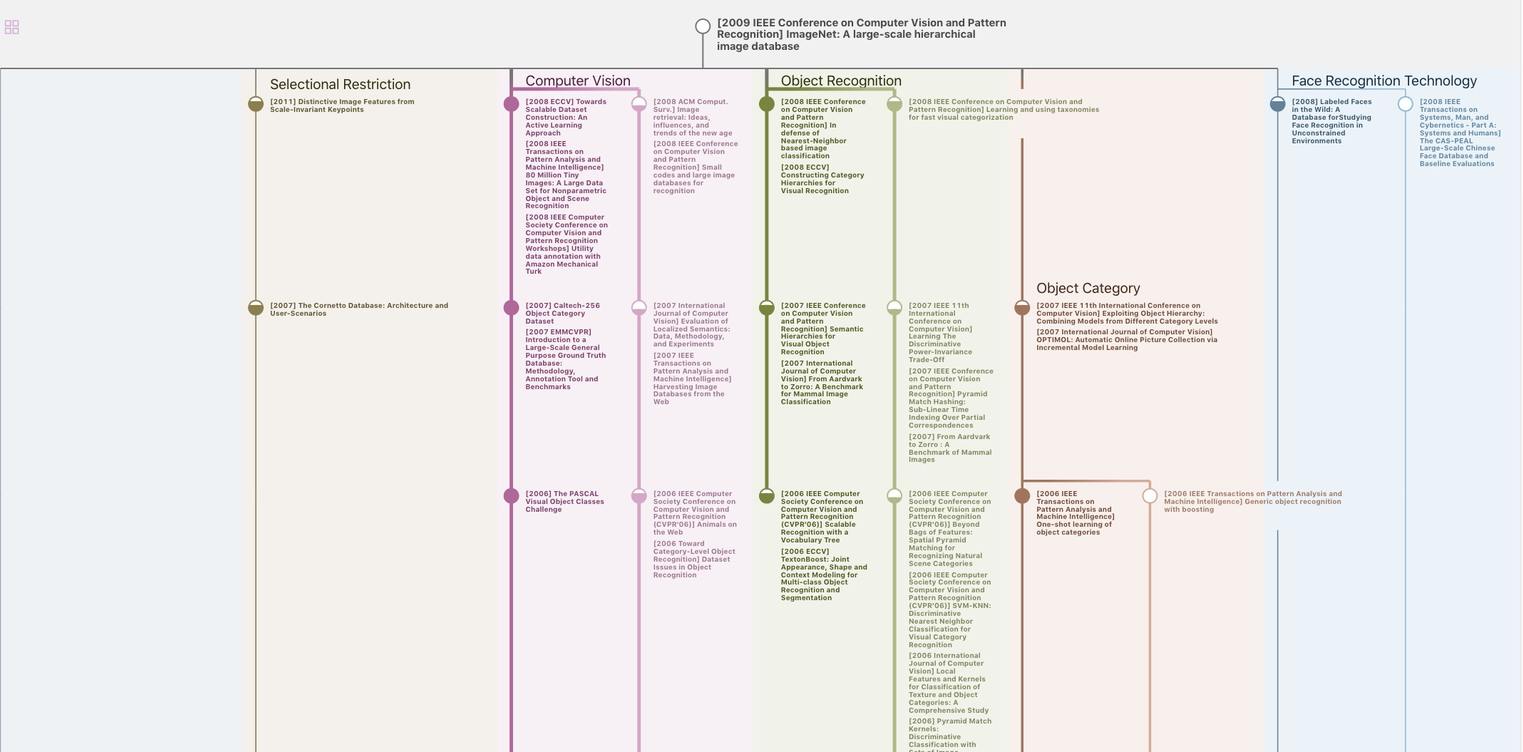
生成溯源树,研究论文发展脉络
Chat Paper
正在生成论文摘要