Sequential Bayesian Optimisation For Spatial-Temporal Monitoring
UAI'14: Proceedings of the Thirtieth Conference on Uncertainty in Artificial Intelligence(2014)
摘要
Bayesian Optinisationhas received considerable attention in recent years as a general methodology to find the maximum of costly-to-evaluate objective functions. Most existing BO work focuses on where to gather a set of samples without giving special consideration to the sampling sequence, or the costs or constraints associated with that sequence. However, in real-world sequential decision problems such as robotics, the order in which samples are gathered is paramount, especially when the robot needs to optimise a temporally non-stationary objective function. Additionally, the state of the environment and sensing platform determine the type and cost of samples that can be gathered. To address these issues, we formulate Sequential Bayesian Optinisation(SBO) with side-state information within a Partially Observed Markov Decision Process (POMDP) framework that can accommodate discrete and continuous observation spaces. We build on previous work using Monte-Carlo Tree Search (MCTS) and Upper Confidence boundfor Trees (UCT) for POMDPs and extend it to work with continuous state and observation spaces. Through a series of experiments on monitoring a spatial-temporal process with a mobile robot, we show that our UCT-based SBO POMDP optimisation outperforms myopic and non-myopic alternatives.
更多查看译文
AI 理解论文
溯源树
样例
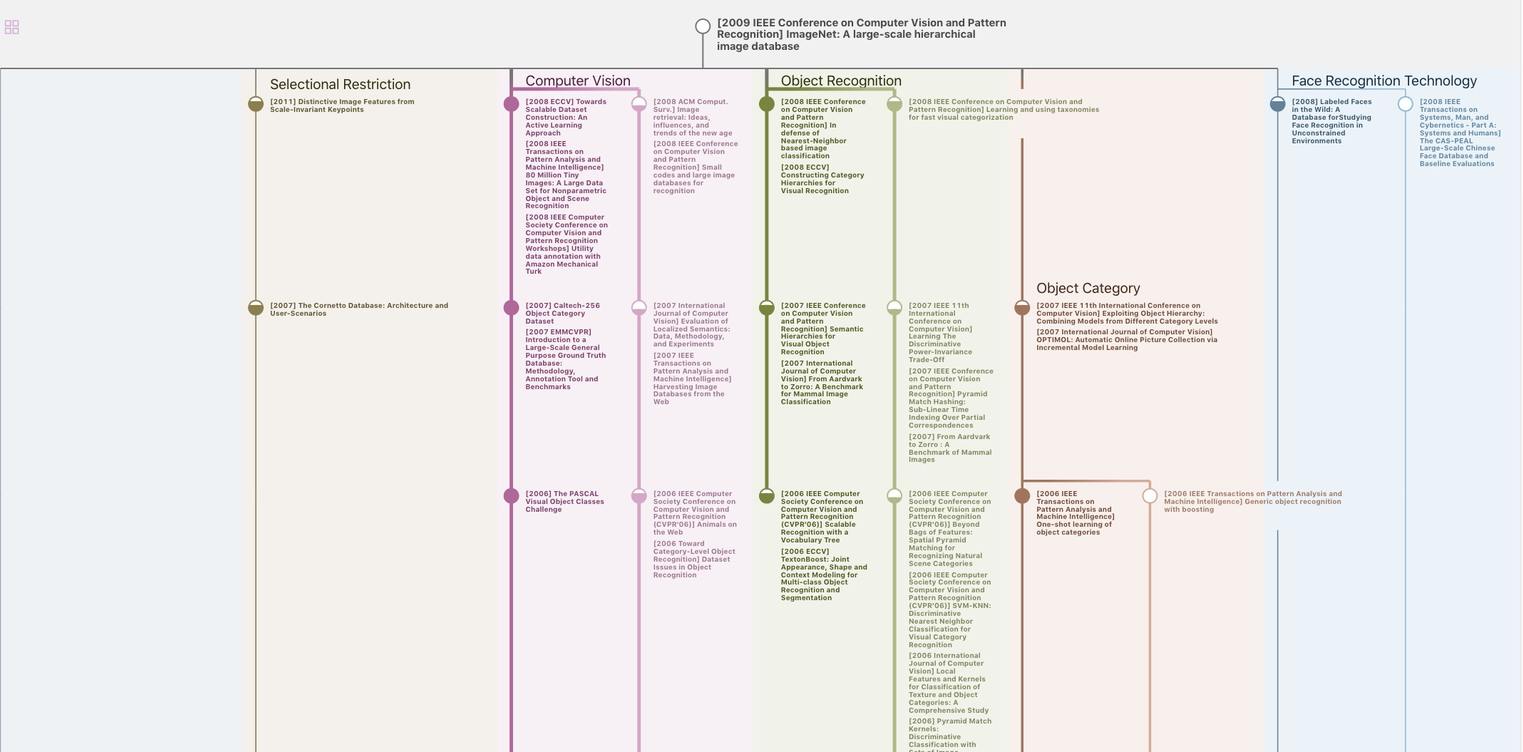
生成溯源树,研究论文发展脉络
Chat Paper
正在生成论文摘要