Early Detection Of Fraud Storms In The Cloud
ECMLPKDD'15: Proceedings of the 2015th European Conference on Machine Learning and Knowledge Discovery in Databases - Volume Part III(2015)
摘要
Cloud computing resources are sometimes hijacked for fraudulent use. While some fraudulent use manifests as a small-scale resource consumption, a more serious type of fraud is that of fraud storms, which are events of large-scale fraudulent use. These events begin when fraudulent users discover new vulnerabilities in the sign up process, which they then exploit in mass. The ability to perform early detection of these storms is a critical component of any cloud-based public computing system.In this work we analyze telemetry data from Microsoft Azure to detect fraud storms and raise early alerts on sudden increases in fraudulent use. The use of machine learning approaches to identify such anomalous events involves two inherent challenges: the scarcity of these events, and at the same time, the high frequency of anomalous events in cloud systems.We compare the performance of a supervised approach to the one achieved by an unsupervised, multivariate anomaly detection framework. We further evaluate the system performance taking into account practical considerations of robustness in the presence of missing values, and minimization of the model's data collection period.This paper describes the system, as well as the underlying machine learning algorithms applied. A beta version of the system is deployed and used to continuously control fraud levels in Azure.
更多查看译文
AI 理解论文
溯源树
样例
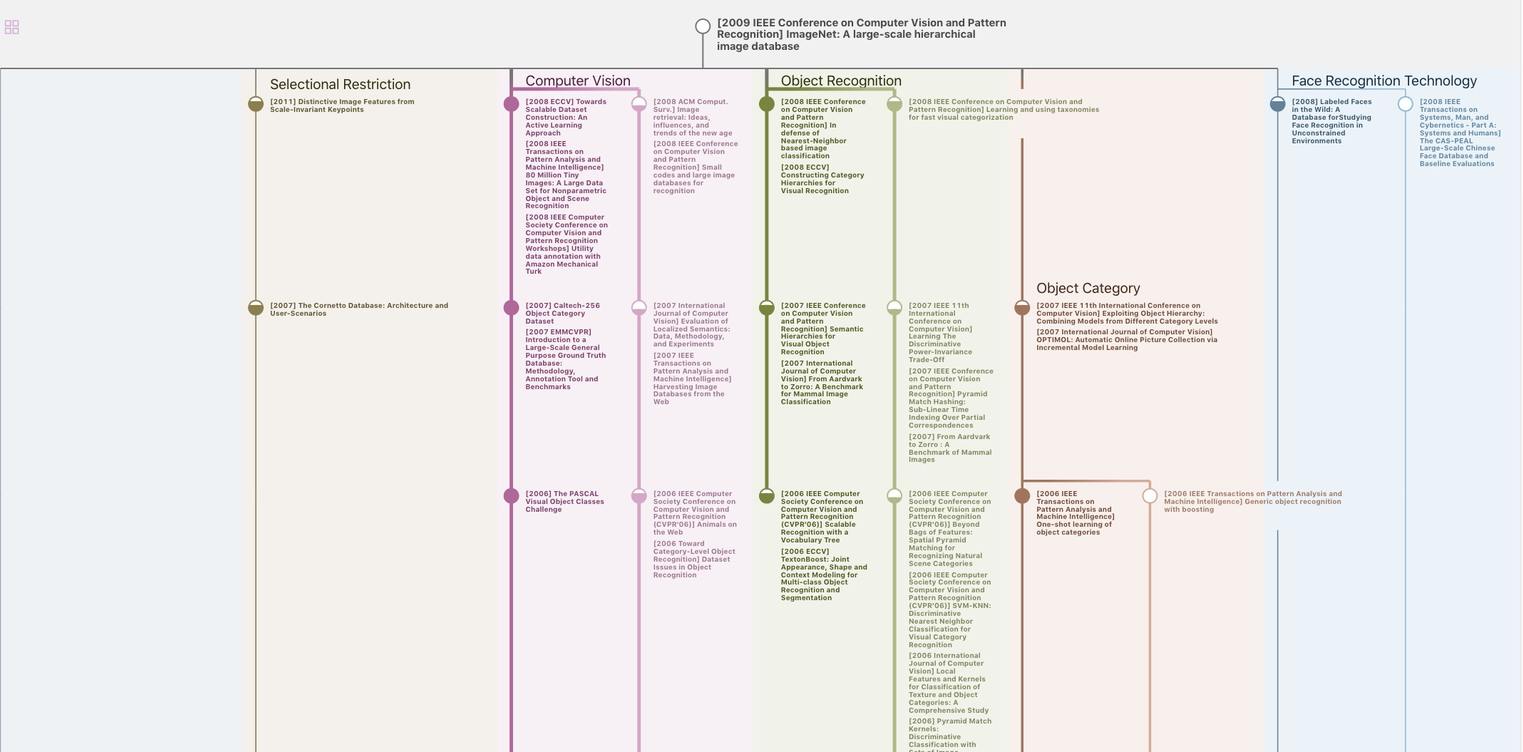
生成溯源树,研究论文发展脉络
Chat Paper
正在生成论文摘要