Detecting Data Semantic: A Data Leakage Prevention Approach
TrustCom/BigDataSE/ISPA(2015)
摘要
Data leakage prevention systems (DLPSs) are increasingly being implemented by organizations. Unlike standard security mechanisms such as firewalls and intrusion detection systems, DLPSs are designated systems used to protect in use, at rest and in transit data. DLPSs analytically use the content and surrounding context of confidential data to detect and prevent unauthorized access to confidential data. DLPSs that use content analysis techniques are largely dependent upon data fingerprinting, regular expressions, and statistical analysis to detect data leaks. Given that data is susceptible to change, data fingerprinting and regular expressions suffer from shortcomings in detecting the semantics of evolved confidential data. However, statistical analysis can manage any data that appears fuzzy in nature or has other variations. Thus, DLPSs with statistical analysis capabilities can approximate the presence of data semantics. In this paper, a statistical data leakage prevention (DLP) model is presented to classify data on the basis of semantics. This study contributes to the data leakage prevention field by using data statistical analysis to detect evolved confidential data. The approach was based on using the well-known information retrieval function Term Frequency-Inverse Document Frequency (TF-IDF) to classify documents under certain topics. A Singular Value Decomposition (SVD) matrix was also used to visualize the classification results. The results showed that the proposed statistical DLP approach could correctly classify documents even in cases of extreme modification. It also had a high level of precision and recall scores.
更多查看译文
关键词
Data leakage prevention, Data semantics, Statistical analysis, Singular Value Decomposition
AI 理解论文
溯源树
样例
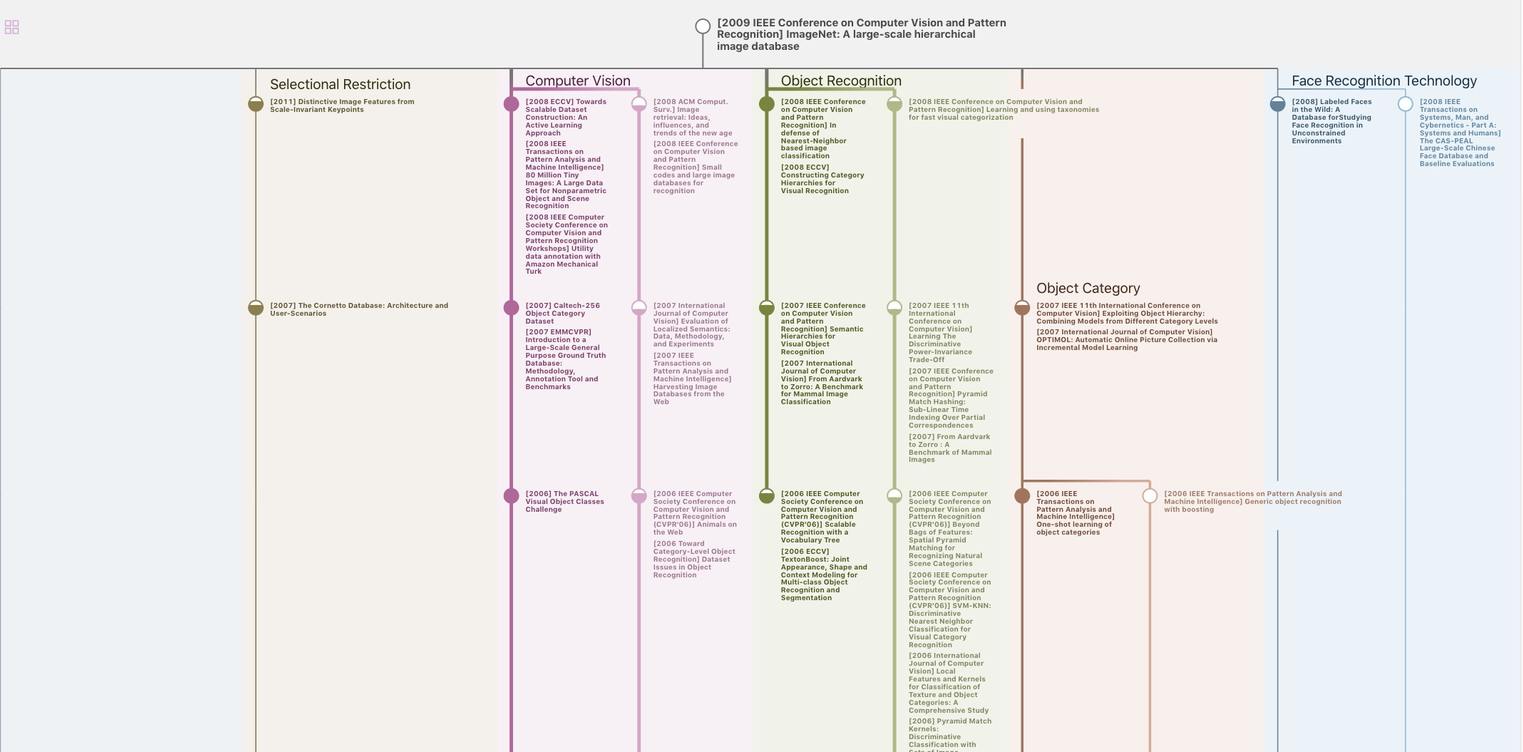
生成溯源树,研究论文发展脉络
Chat Paper
正在生成论文摘要