Exploriometer: Leveraging Personality Traits For Coverage And Diversity Aware Recommendations
WWW '15: 24th International World Wide Web Conference Florence Italy May, 2015(2015)
摘要
Since the first introduced Collaborative Filtering Recommenders (CFR), there have been many attempts to improve their performance by enhancing the prediction accuracy. Even though rating prediction is the prevailing paradigm in CFR, there are other issues which have gained significant attention with respect to the content and its variety. Coverage, which constitutes the degree to which recommendations cover the set of available items, is an important factor along with diversity of the items proposed to an individual, often measured by an average dissimilarity between all pairs of recommended items.In this paper, we argue that coverage and diversity cannot be effectively addressed by conventional CFR with pure similarity-based neighborhood creation processes, especially in sparse datasets. Motivated by the need for including wider content characteristics, we propose a novel neighbor selection technique which emphasizes on variety in preferences (to cover polyphony in selection). Our approach consists of a new metric, named "Exploriometer", which acts as a personality trait for users based on their rating behavior. We favor users who are explorers in order to increase polyphony, and subsequently coverage and diversity; but we still select similar users when we create neighborhoods as a solid basis in order to keep accuracy levels high. The proposed approach has been experimented by two real-world datasets (MovieLens(1) and Yahoo! Music(2)) with coverage, diversity and accuracy aware recommendations extracted by both traditional CFR and CFR enhanced with our neighborhood creation process. We also introduce a new metric, inspired by the Pearson Correlation Coefficient, to estimate the diversity of recommended items. The derived results demonstrate that our neighbor selection technique can enhance coverage and diversity of the recommendations, especially on sparse datasets.
更多查看译文
关键词
Collaborative Filtering Systems,Diversity,Coverage,Neighbor Selection
AI 理解论文
溯源树
样例
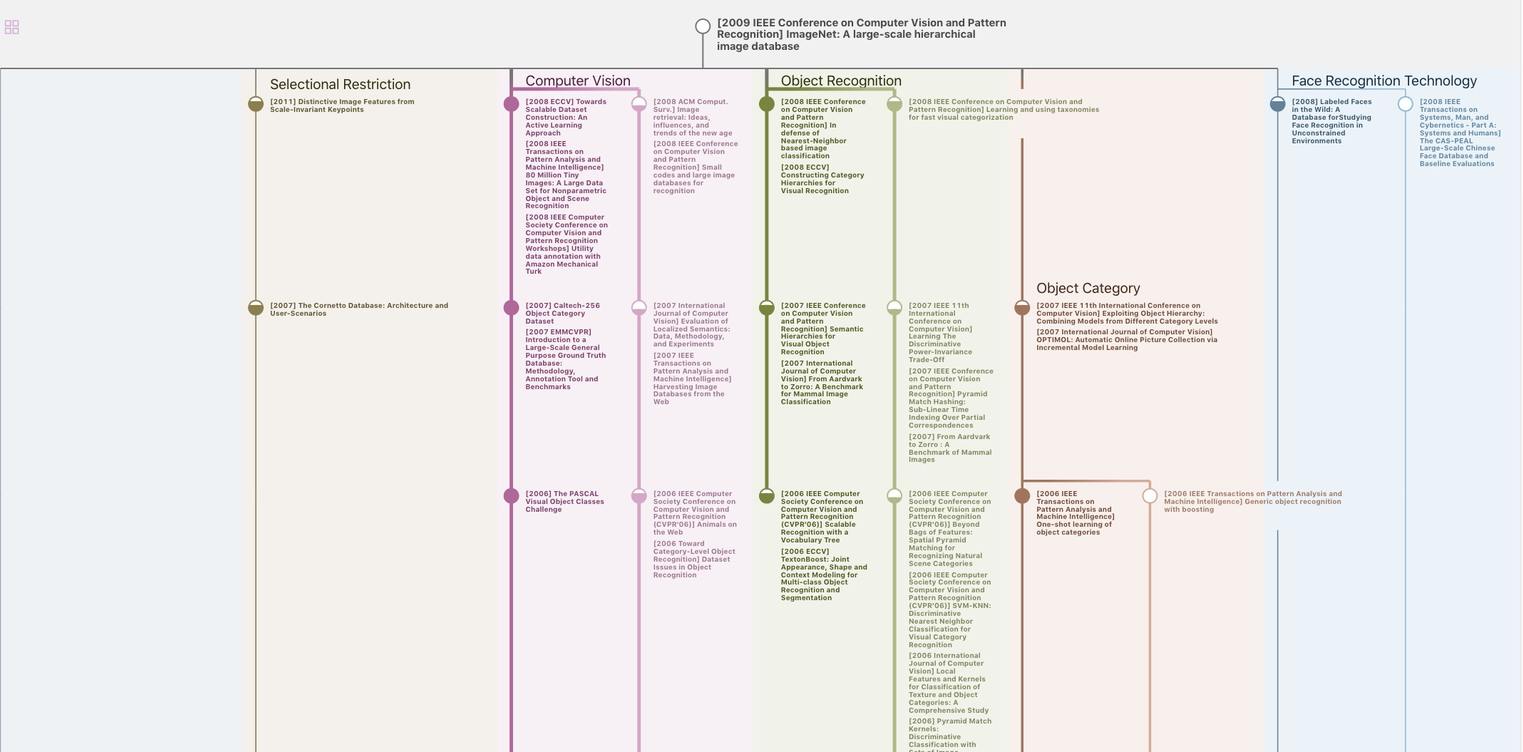
生成溯源树,研究论文发展脉络
Chat Paper
正在生成论文摘要