Harnessing Big Personal Data, With Scrutable User Modelling For Privacy And Control
MM '15: ACM Multimedia Conference Brisbane Australia October, 2015(2015)
摘要
My work aims to enable people to harness, control and manage their big personal data. This is challenging because people are generating vast, and growing, collections of personal data. That data is captured by a rich personal digital ecosystems of devices, some worn or carried, and others are fixed or embedded in the environment. Users explicitly store some data but systems also capture the user's digital footprints, ranging from simple clicks and touches, to images, audio and video. This personal data resides in a quite bewildering range of places, from personal devices to cloud stores, in multitudes of silos.Big personal data differs from the scientific big data in important ways. Because it is personal, it should be handled in ways that enable people to ensure it is managed and used as they wish. It may be of modest size compared with scientific big data, but people consider their data stores as big, because they are complex and hard to manage.A driving goal for my research has been to tackle the challenges of big personal data by creating infrastructures, representations and interfaces that enable a user to scrutinize and control their personal data in a scrutable user model.One important role for users models is personalisation, where the user model is a dynamic set of evidence-based beliefs about the user. This is the foundation for personalization, ranging from recommenders to teaching systems. User models may represent anything from the user's attributes to their knowledge, beliefs, goals, plans and preferences. Some user modeling evidence comes directly from the user; for example, people typically provide dating sites with rich (if not entirely accurate) descriptions of themselves and about the people they believe that would like. Much commercial user modeling evidence comes from observing the user; for example, a personalized teaching system observes the learner's interactions, as they use learning resources and tackle problem and from this data the system infers what the learner knows. Typically, a user model refines raw data about the user and combines it with other knowledge. For example, teaching systems make use of a knowledge model of the domain and recommenders use collaborative filters based on user models of many people. A scrutable user model is designed to enable people to scrutinize their own user model, the processes used to form it and the ways that it is used.A second important role for the user model is as an independent first class citizen; user models can be valuable in their own right, independently of whether they are used by any personalised system. Such user models have the potential to support diverse forms of learning. This is because they can mirror salient aspects of the user over the long term, and when there is a suitable interface to these models, they can provide a valuable resource. In education, this is described in terms of important self-regulated learning processes, such as reflection, self-monitoring and planning. Some broader uses are central to the Quantified Self movement.The talk presents a series of four linked case studies of my work:1. Infrastructures for scrutable user modeling that are designed to people to determine what it in the user model and how it is collected, used, managed and forgotten [3, 1];2. Open Learner Models [2] that can be independent or within personalized teaching systems and MOOCs [4];3. Harnessing personal data to tackle the Sisyphean challenge of lifelong self -regulated activity needed for health and wellness;4. Harnessing rich streams of depth images, video, audio, touch using surface computing to create collaboration technologies that support collaborative learning [5].
更多查看译文
AI 理解论文
溯源树
样例
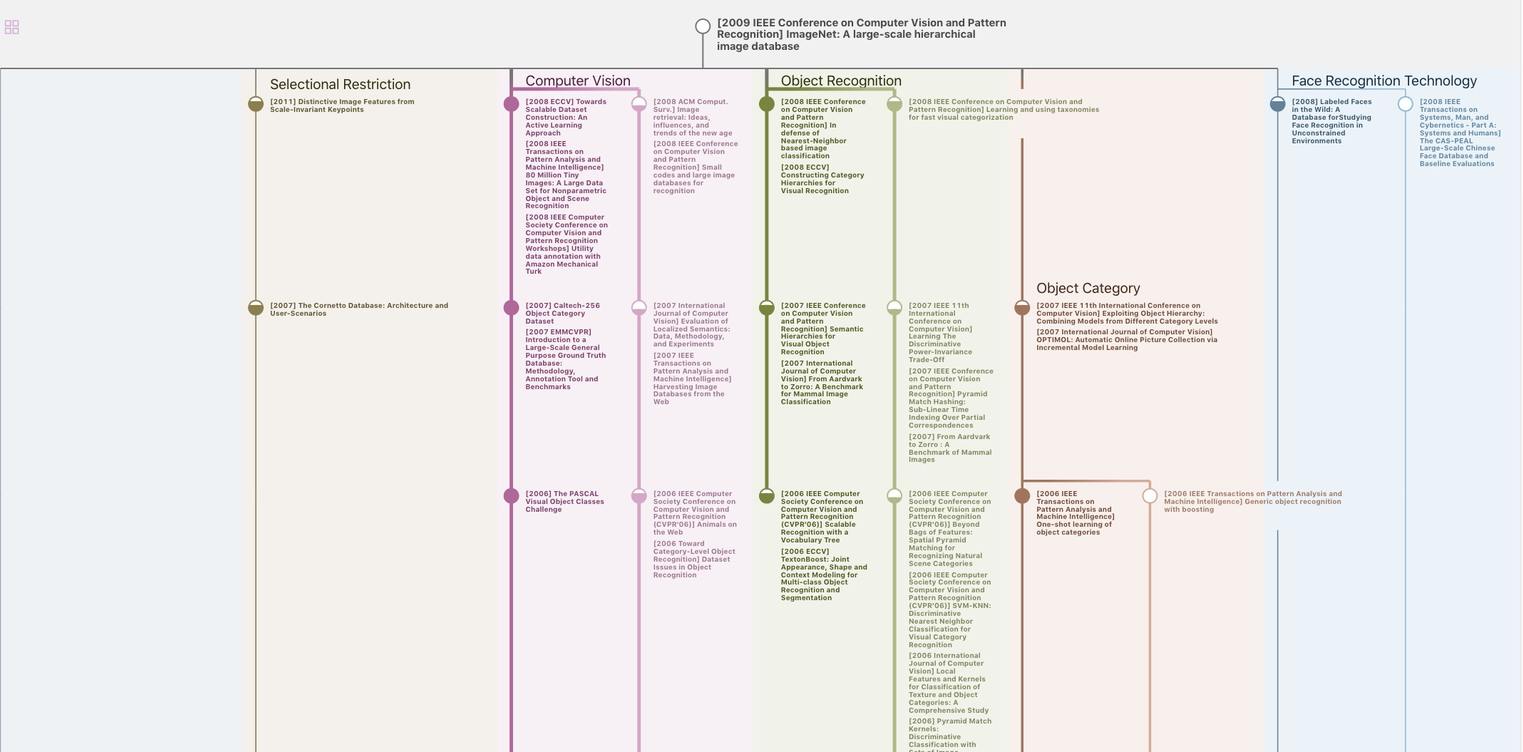
生成溯源树,研究论文发展脉络
Chat Paper
正在生成论文摘要