Scalable Large Near-Clique Detection In Large-Scale Networks Via Sampling
KDD '15: The 21th ACM SIGKDD International Conference on Knowledge Discovery and Data Mining Sydney NSW Australia August, 2015(2015)
摘要
Extracting dense subgraphs from large graphs is a key primitive in a variety of graph mining applications, ranging from mining social networks and the Web graph to bioinformatics [41]. In this paper we focus on a family of poly-time solvable formulations, known as the k-clique densest sob graph problem (K-CLIQUE-DSP) [57]. When k = 2, the problem becomes the well-known densest sabgraph, problem (DSP) [22, :11, :191. Our main contribution is a sampling scheme that gives densest subgraph sparsifier, yielding a randomized algorithm that produces high-quality approximations while providing significant speedups and improved space complexity. We also extend this family of formulations to bipartite graphs by introducing the (p, q)-biclique densest subgraph problem ((P,Q)-BickiQuE-DSP), and devise an exact algorithm that can treat both clique and biclique densities in a unified way.As an example of performance, our sparsifying algorithm extracts the 5-clique densest subgraph which is a large-near clique on 62 vertices from a large collaboration network. Our algorithm achieves 100% accuracy over five runs, while achieving arm average speedup factor of over 10000. Specifically, we reduce the running time from similar to 2 107 seconds to an average running time of 0.15 seconds. We also use our methods to study how the k-clique densest subgraphs change as a function of time in time-evolving networks for various small values of k. We observe significant deviations between the experimental findings on real-world networks and stochastic Kronecker graphs, a random graph model that mimics real-world networks in certain aspects.We believe that our work is a significant advance in routines with rigorous theoretical guarantees for scalable extraction of large near-cliques from networks.
更多查看译文
关键词
Dense subgraphs,Near-clique extraction,Graph Mining
AI 理解论文
溯源树
样例
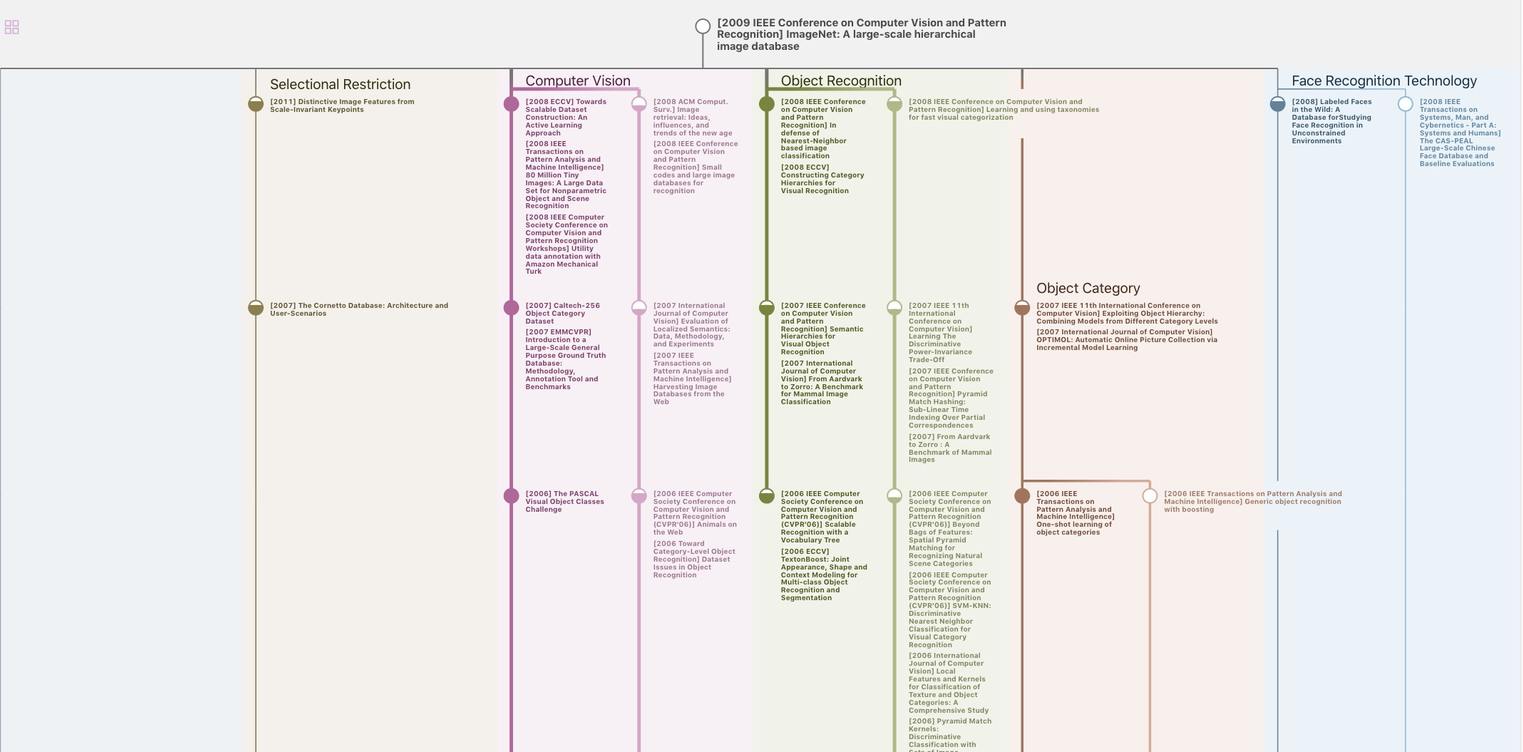
生成溯源树,研究论文发展脉络
Chat Paper
正在生成论文摘要