Estimating load carriage from a body-worn accelerometer
2015 IEEE 12th International Conference on Wearable and Implantable Body Sensor Networks (BSN)(2015)
摘要
Heavy loads increase the risk of musculoskeletal injury for foot soldiers and first responders. Continuous monitoring of load carriage in the field has proven difficult. We propose an algorithm for estimating load from a single body-worn accelerometer. The algorithm utilizes three different methods for characterizing torso movement dynamics, and maps the extracted dynamics features to load estimates using two machine learning multivariate regression techniques. The algorithm is applied, using leave-one-subject-out cross-validation, to two field collections of soldiers and civilians walking with varying loads. Rapid, accurate estimates of load are obtained, demonstrating robustness to changes in equipment configuration, walking conditions, and walking speeds. On soldier data with loads ranging from 45 to 89 lbs, load estimates result in mean absolute error (MAE) of 6.64 lbs and correlation of r = 0.81. On combined soldier and civilian data, with loads ranging from 0 to 89 lbs, results are MAE = 9.57 lbs and r = 0.91.
更多查看译文
关键词
musculoskeletal injury,foot soldiers,first responders,continuous load carriage monitoring,single body-worn accelerometer,torso movement dynamics,feature extraction,machine learning multivariate regression techniques,leave-one-subject-out cross-validation,equipment configuration,walking conditions,walking speeds,mean absolute error,MAE,soldier data,civilian data,mass 45 lb to 89 lb
AI 理解论文
溯源树
样例
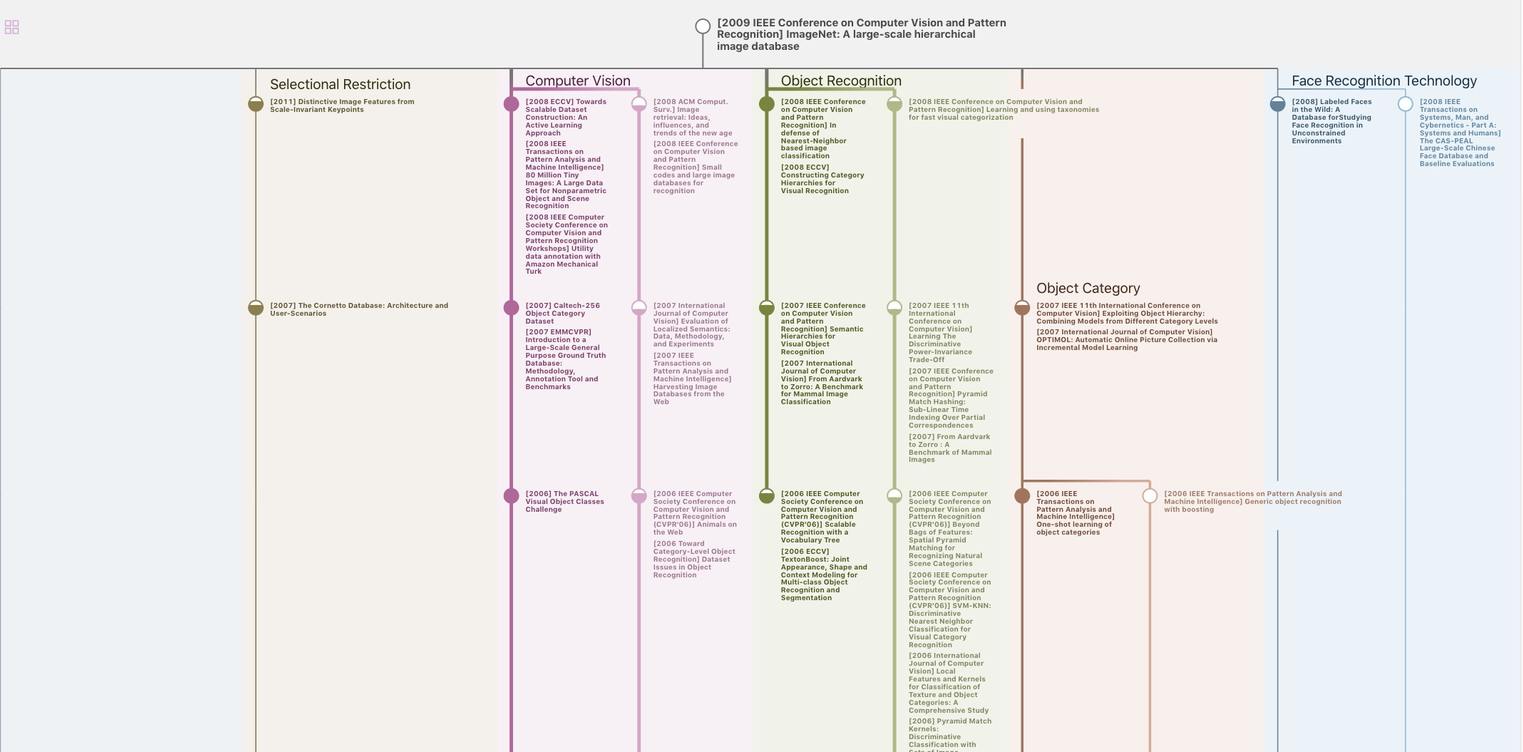
生成溯源树,研究论文发展脉络
Chat Paper
正在生成论文摘要