Slowing the Firehose: Multi-Dimensional Diversity on Social Post Streams.
EDBT(2016)
摘要
Web 2.0 users conveniently consume content through subscribing to content generators such as Twitter users or news agencies. However, given the number of subscriptions and the rate of the subscription streams, users suffer from the information overload problem. To address this issue, we propose a novel and flexible diversification paradigm to prune redundant posts from a collection of streams. A key novelty of our diversification model is that it holistically incorporates three important dimensions of social posts, namely content, time and author. We show how different applications, such as microblogging, news or bibliographic services, require different settings for these three dimensions. Further, each dimension poses unique performance challenges towards scaling the diversification model for many users and many high-throughput streams. We show that hash-based content distance measures and graph-based author distance measures are both effective and efficient for social posts. We propose scalable real-time stream processing algorithms leveraging efficient indexes that input a social post stream and output a diversified version of the stream, diversified across all three dimensions. Next, we show how these techniques can be extended to serve multiple users by appropriately reusing indexing and computation where possible. Through extensive experiments on real Twitter data, we show that our diversification model is effective and our solutions are scalable. We show that different algorithms perform best for different application settings.
更多查看译文
AI 理解论文
溯源树
样例
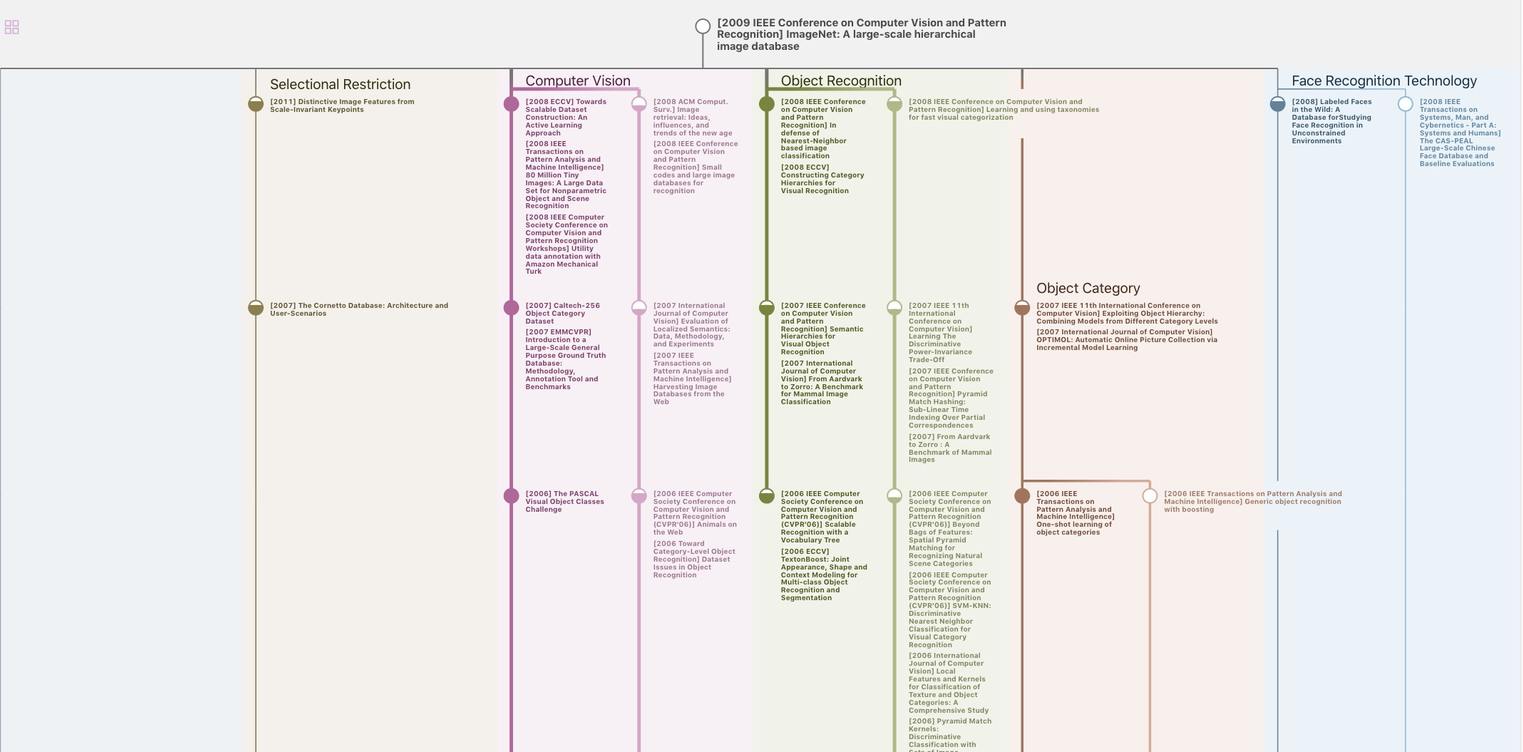
生成溯源树,研究论文发展脉络
Chat Paper
正在生成论文摘要