Tracking appliance usage information using harmonic signature sensing
2015 IEEE International Conference on Smart Grid Communications (SmartGridComm)(2015)
摘要
Real-time usage of individual electrical appliances is a key enabler of important advanced services for smart grids. With wide deployments of smart meters, there is a growing interest in using Non-Intrusive Load Monitoring (NILM) to acquire this information from the meter measurements. However, electrical signatures extracted from utility-side smart meters are often unreliable for NILM due to their large sampling intervals. This paper presents a new approach of using high-frequency current waveforms sampled periodically at a main branch to track reliably the on/off states of appliances in real-time. We develop an incremental training algorithm and a robust detection algorithm for the harmonic signatures, based on semi-supervised learning and a hidden Markov model, respectively. We evaluate the performance of the training and detection algorithms using simulations and a proof-of-concept testbed with five appliances. The simulation results show that our state detection algorithm is highly robust against noisy harmonic signatures - up to 16 times more robust than a baseline algorithm without the hidden Markov model. The experimental results show that the proposed algorithms can successfully learn most harmonic signatures using only 10% of label information. They can detect the on/off states with less than 4 % errors.
更多查看译文
关键词
tracking appliance usage information,harmonic signature sensing,individual electrical appliance real-time usage,smart grid reliability,nonintrusive load monitoring,NILM,meter measurement,utility-side smart meter deployment,large sampling interval,hidden Markov model,incremental training algorithm,robust detection algorithm,semisupervised learning,baseline algorithm,label information
AI 理解论文
溯源树
样例
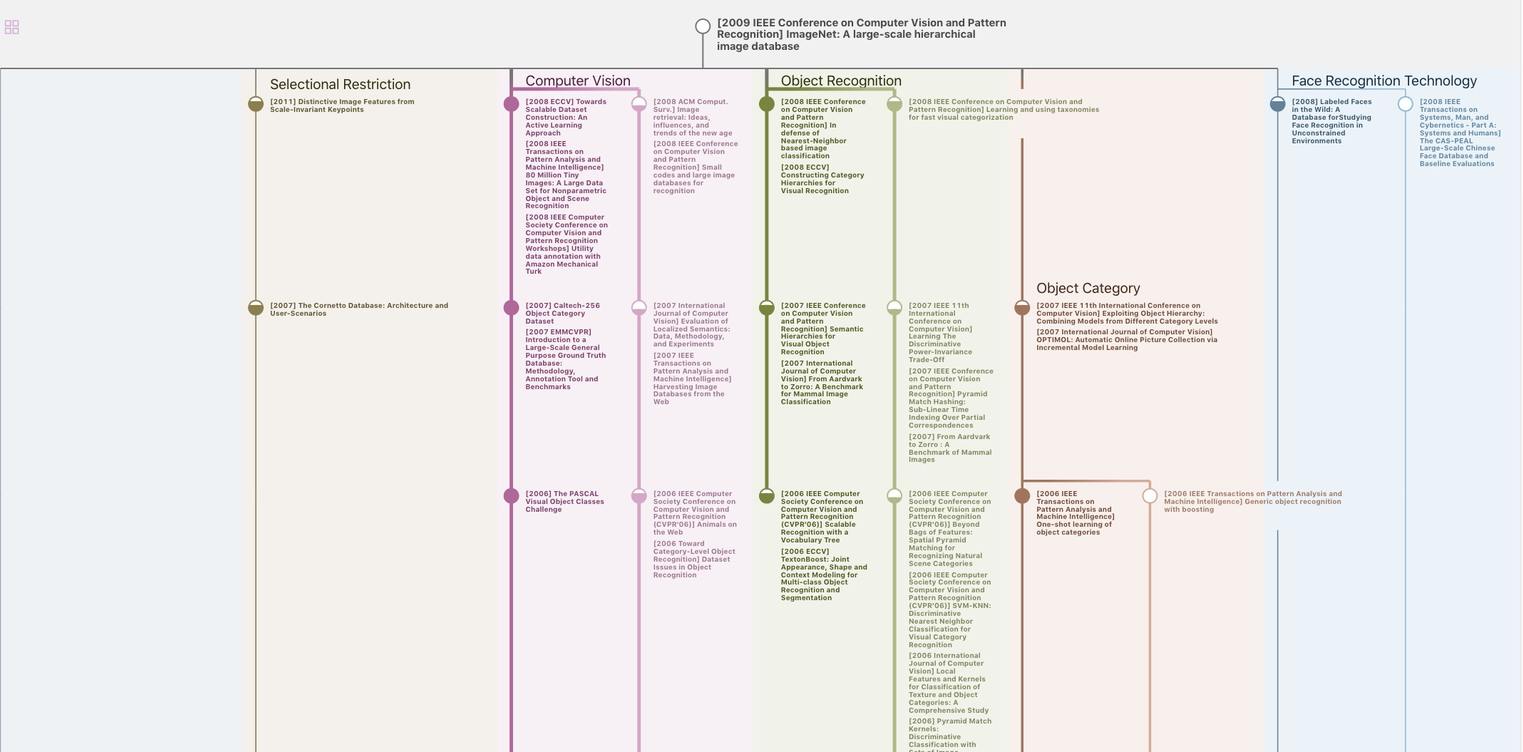
生成溯源树,研究论文发展脉络
Chat Paper
正在生成论文摘要