Exploiting User And Business Attributes For Personalized Business Recommendation
SIGIR '15: The 38th International ACM SIGIR conference on research and development in Information Retrieval Santiago Chile August, 2015(2015)
摘要
Data sparsity and cold-start are two major problems in personalized recommendation. They are especially severe in business recommendation, because business transactions are usually completed offline and customers generally do not provide ratings after a transaction. Due to these two problems, matrix factorization (MF) models, which are shown to be effective in many recommendation tasks, are likely to fail on business recommendation tasks, especially for new users and new items. In this paper, we propose an Integrated Bias and Factorization Model (IBFM), which exploits user and business attributes. The user attributes include demographic information, vote information, point-of-interests; the business attributes include check-in information, locations, business names, categories, etc. To handle the cold-start problem, we employ a sampling strategy to generate the latent factor vectors for new users and new businesses based on similar users/businesses. Our methods are evaluated on the data set used in the RecSys 2013 Yelp business rating prediction challenge. Experimental results show that our proposed methods significantly outperform several existing state-of-the-art methods. In particular, the single model IBFM performs the best in this challenge on both public and private leaderboards.
更多查看译文
关键词
Collaborative Filtering,Data Sparsity,Cold-start,Context Information,Matrix Factorization
AI 理解论文
溯源树
样例
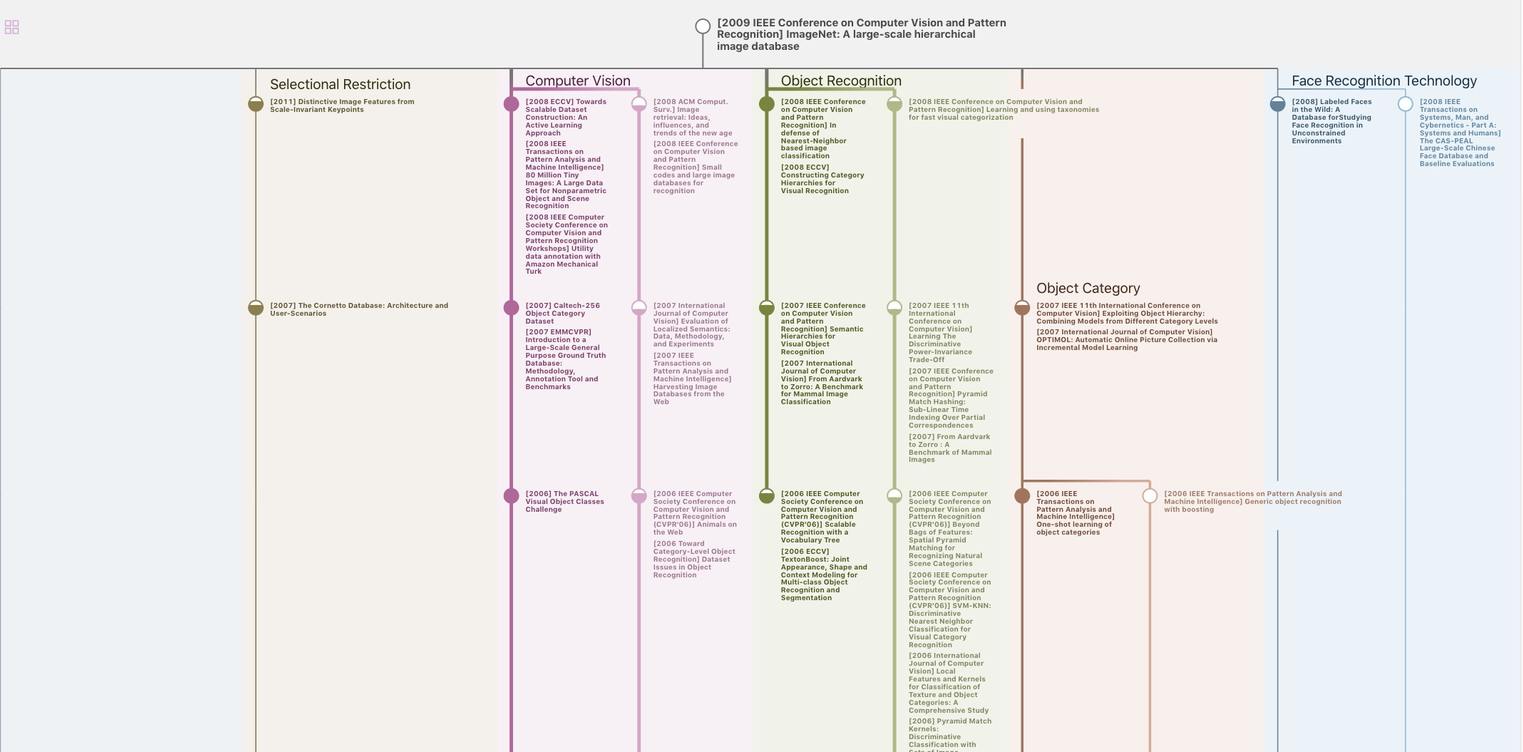
生成溯源树,研究论文发展脉络
Chat Paper
正在生成论文摘要