When in doubt ask the crowd: leveraging collective intelligence for improving event detection and machine learning.
dblp(2015)
摘要
The Internet and more specifically Web 2.0 is a promoter and enhancer of collective intelligence as it allows people to easily generate, store and retrieve information that can be shared without difficulty. Thus both the expression and exploitation of the wisdom of the crowds are facilitated by applications relying on collective and collaborative intelligence. The contribution of this thesis in leveraging the wisdom of the crowds effect is twofold. First, we propose methods for extracting event-related information from the collectively contributed Wikipedia, thus helping those who are interested in having a comprehensive overview of a happening to address their doubts about the reported facts. Second, we provide ways of exploiting on-demand requested wisdom of the crowds, by involving crowdsourcing in supervised machine learning. Thus, machines can call the crowd and ask for assistance whenever there are doubts about the tasks that need to be solved. We first focus on how collaborative intelligence in Wikipedia is manifested in the process of information actualization as a reaction to the new events. Real-world events directly influence the collaborative editing of Wikipedia articles about related entities. Consequently, as new events take place all over the world, Wikipedia users update the articles corresponding to the entities involved in these events, or influenced by them, causing an avalanche of edits on several articles, as more information regarding the event becomes available. The interactions of contributors with the articles give us clues on whether certain updates are event-related or not, or whether concurrent updates are a sign of participation in a common event. We identify and leverage these patterns in order to identify those updates that are a consequence of events and summarize them in a comprehensive way that presents all relevant information, even if intentionally forgotten. Moreover, as events can be defined as relationships between entities at a certain time point caused by a common happening, we investigate how concurrent edits can be used as indicators for entities being involved in common events. We then concentrate on how machine learning can benefit from crowdsourcing. We first propose methods to aggregate multiple crowd labels in order to produce reliable annotated content that can be used for supervised machine learning. The proposed methods take advantage of the workers’ history of already solved tasks in order to simultaneously assess worker expertise and find the underlying hidden labels. Then, we go a step further, and propose to couple active learning with crowdsourcing in an integrated framework. Thus, machines and humans can work together towards improving their performance at a specific task. An automatic algorithm can learn from the crowd how to do its task in an active learning manner. When the algorithm has a doubt about a label, or needs to reduce the doubts over the task at hand, it can directly ask the crowd, and get the reliable labels that it needs in order for it to become better. The proposed integrated framework accounts for different worker expertise, instance selection strategies, as well as various levels of resource allocation.
更多查看译文
AI 理解论文
溯源树
样例
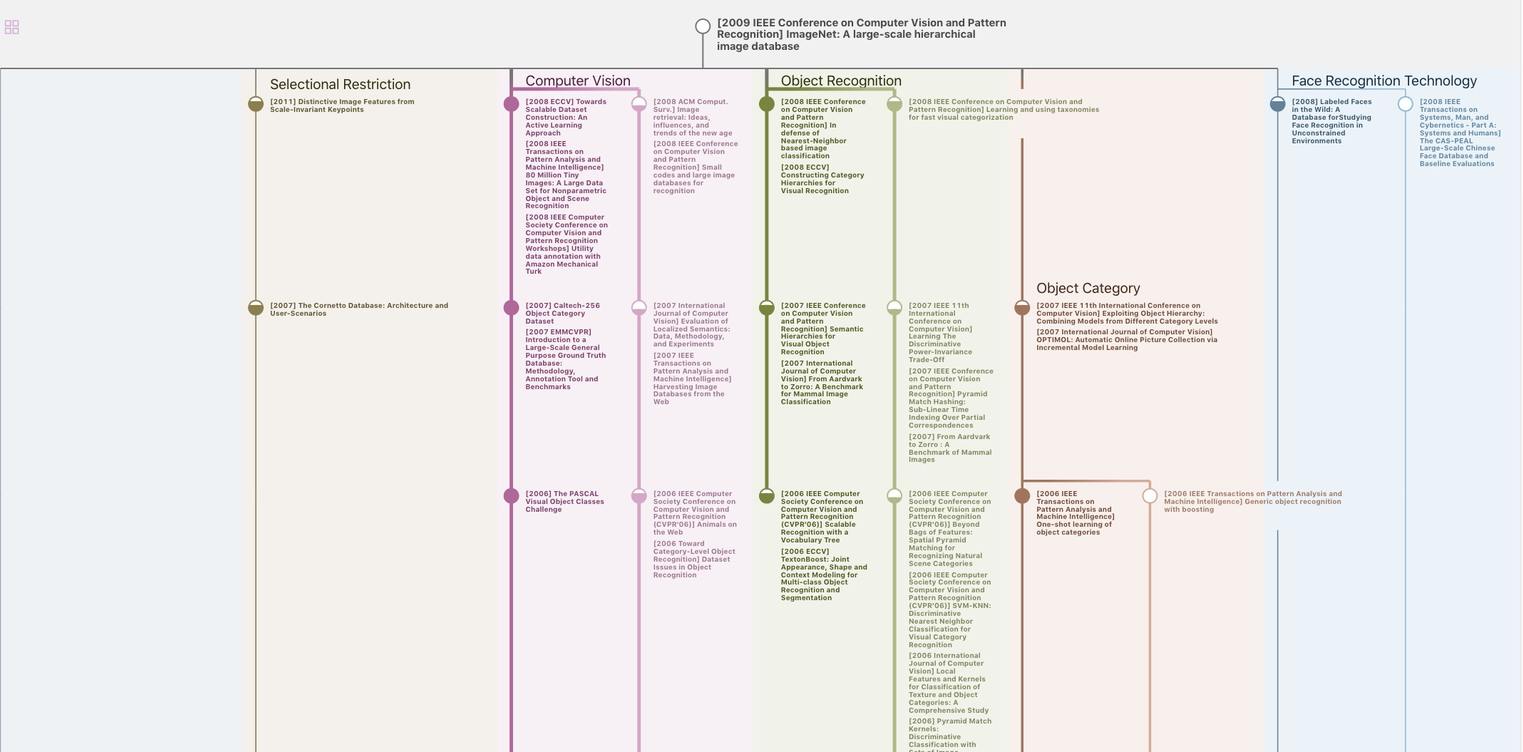
生成溯源树,研究论文发展脉络
Chat Paper
正在生成论文摘要