Exemplar Based Deep Discriminative and Shareable Feature Learning for Scene Image Classification
Pattern Recognition(2015)
摘要
In order to encode the class correlation and class specific information in image representation, we propose a new local feature learning approach named Deep Discriminative and Shareable Feature Learning (DDSFL). DDSFL aims to hierarchically learn feature transformation filter banks to transform raw pixel image patches to features. The learned filter banks are expected to (1) encode common visual patterns of a flexible number of categories; (2) encode discriminative information; and (3) hierarchically extract patterns at different visual levels. Particularly, in each single layer of DDSFL, shareable filters are jointly learned for classes which share the similar patterns. Discriminative power of the filters is achieved by enforcing the features from the same category to be close, while features from different categories to be far away from each other. Furthermore, we also propose two exemplar selection methods to iteratively select training data for more efficient and effective learning. Based on the experimental results, DDSFL can achieve very promising performance, and it also shows great complementary effect to the state-of-the-art Caffe features. HighlightsWe propose to encode shareable and discriminative information in feature learning.Two exemplar selection methods are proposed to select effective training data.We build a hierarchical learning scheme to capture multiple visual level information.Our DDSFL outperforms most of the existing features.DDSFL features show great complementary effect to Caffe features.
更多查看译文
关键词
Deep feature learning,Information sharing,Discriminative training,Scene image classification
AI 理解论文
溯源树
样例
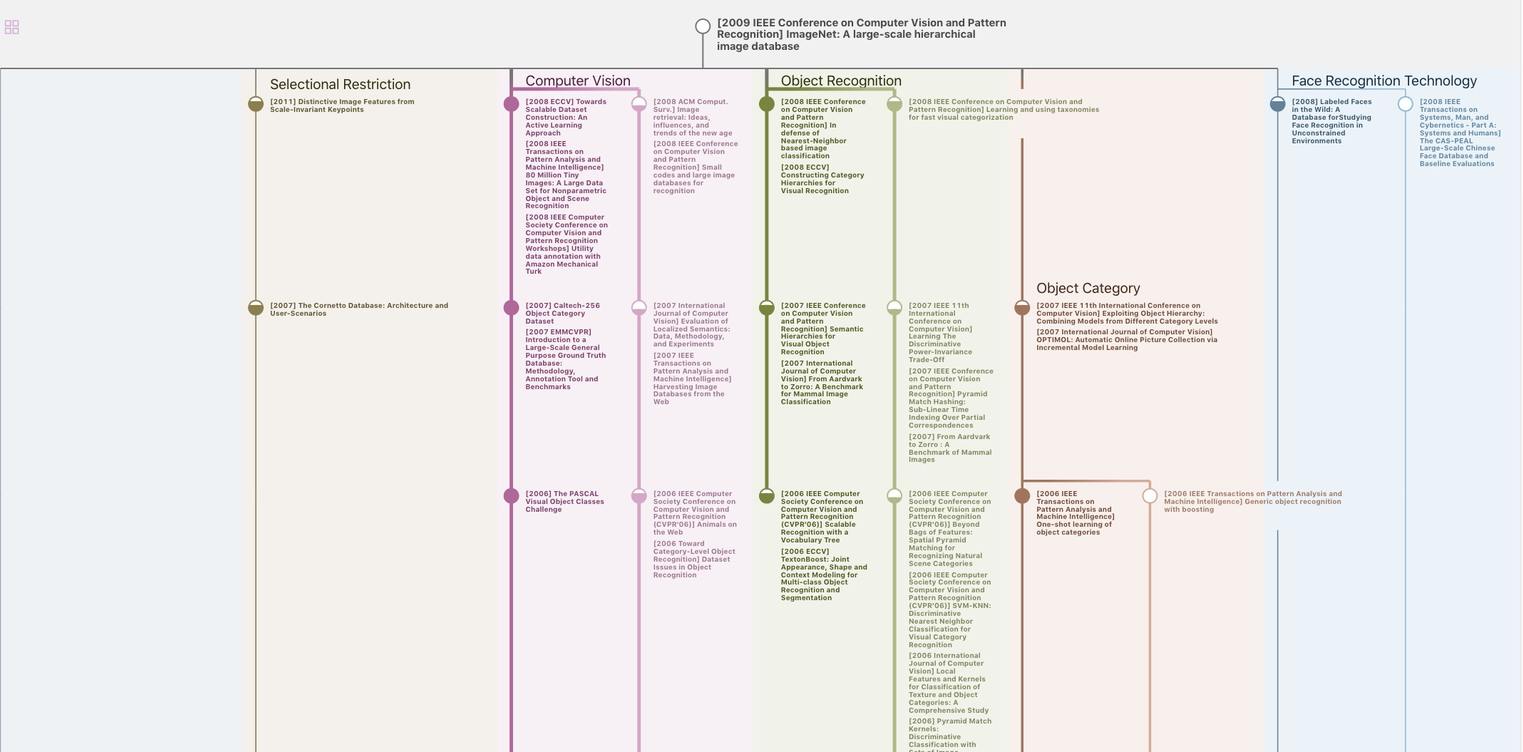
生成溯源树,研究论文发展脉络
Chat Paper
正在生成论文摘要