Max-Margin Discriminant Projection via Data Augmentation
IEEE Transactions on Knowledge and Data Engineering(2015)
摘要
In this paper, we introduce a new max-margin discriminant projection method, which takes advantage of the latent variable representation for support vector machine (SVM) as the classification criterion. Specifically, the proposed model jointly learns the discriminative subspace and classifier in a Bayesian framework by conditioning on augmented variables. Moreover, an extended nonlinear model is developed based on the kernel trick, where the similar model can be used in this setting with few modifications. To explore the sparsity in the kernel expansion, we use the spike-and-slab prior to seek basis vectors (BVs) from the corresponding candidates. Unlike existing methods, which employ BVs to approximate the original feature space, in our method BVs are sought to associate the final classification task. Thanks to the conditionally conjugate property, the parameters in our models can be inferred via the simple and efficient Gibbs sampler. Finally, we test our methods on synthesized and real-world data, including large-scale data sets to demonstrate their efficiency and effectiveness..
更多查看译文
关键词
Max-margin, Gibbs sampling, feature extraction, kernel methods, radar automatic target recognition (RATR)
AI 理解论文
溯源树
样例
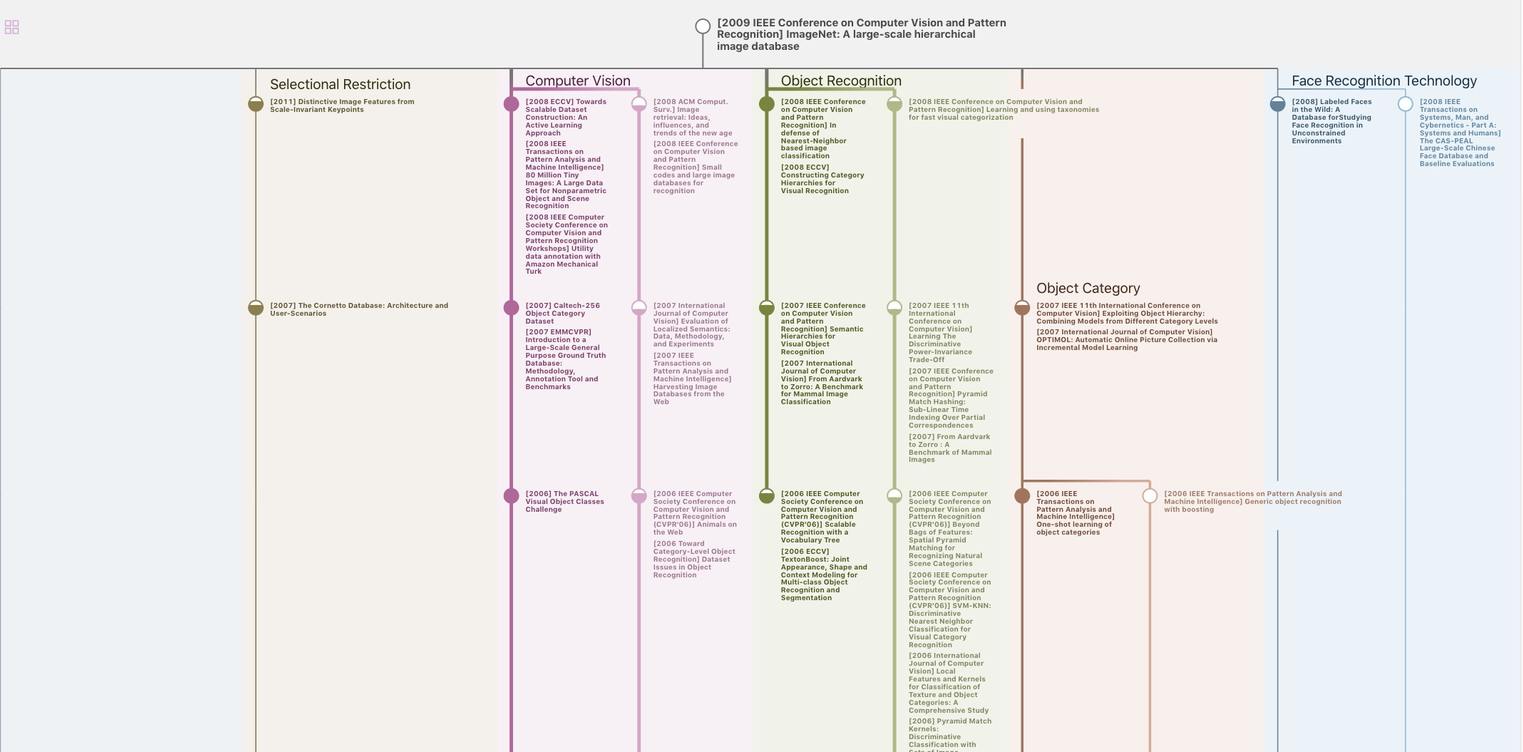
生成溯源树,研究论文发展脉络
Chat Paper
正在生成论文摘要