Rate Analysis for Detection of Sparse Mixtures
2016 IEEE International Conference on Acoustics, Speech and Signal Processing (ICASSP)(2015)
摘要
In this paper, we study the rate of decay of the probability of error for distinguishing between a sparse signal with noise, modeled as a sparse mixture, from pure noise. This problem has many applications in signal processing, evolutionary biology, bioinformatics, astrophysics and feature selection for machine learning. We let the mixture probability tend to zero as the number of observations tends to infinity and derive oracle rates at which the error probability can be driven to zero for a general class of signal and noise distributions. In contrast to the problem of detection of non-sparse signals, we see the log-probability of error decays sublinearly rather than linearly and is characterized through the $\chi^2$-divergence rather than the Kullback-Leibler divergence. Our contributions are: (i) the first characterization of the rate of decay of the error probability for this problem; and (ii) the construction of a test based on the $L^1$-Wasserstein metric that achieves the oracle rate in a Gaussian setting without prior knowledge of the sparsity level or the signal-to-noise ratio.
更多查看译文
关键词
Detection theory,large deviations,error exponents,sparse detection,likelihood ratio test
AI 理解论文
溯源树
样例
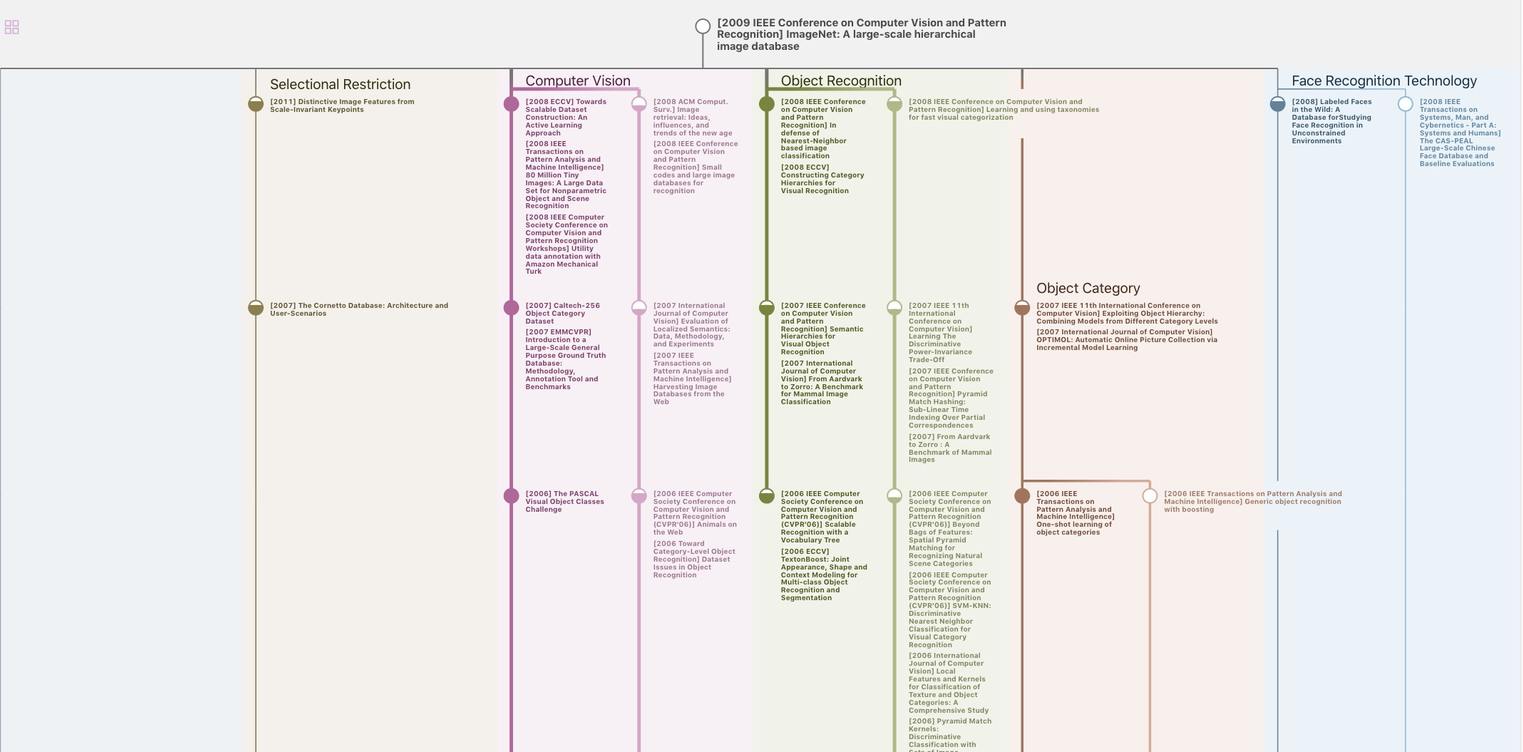
生成溯源树,研究论文发展脉络
Chat Paper
正在生成论文摘要