On Lagrangian Relaxation and Reoptimization Problems
arXiv: Data Structures and Algorithms(2015)
摘要
We prove a general result demonstrating the power of Lagrangian relaxation solving constrained maximization problems with arbitrary objective functions. This yields a unified approach for solving a wide class of {em subset selection} problems with linear constraints. Given a problem this class and some small $eps in (0,1)$, we show that if there exists an $r$-approximation algorithm for the Lagrangian relaxation of the problem, for some $r in (0,1)$, then our technique achieves a ratio of $frac{r}{r+1} -! eps$ to the optimal, and this ratio is tight. The number of calls to the $r$-approximation algorithm, used by our algorithms, is {em linear} the input size and $log (1 / eps)$ for inputs with cardinality constraint, and polynomial the input size and $log (1 / eps)$ for inputs with arbitrary linear constraint. Using the technique we obtain (re)approximation algorithms for natural (reoptimization) variants of classic subset selection problems, including real-time scheduling, the {em maximum generalized assignment problem (GAP)} and maximum weight independent set.
更多查看译文
AI 理解论文
溯源树
样例
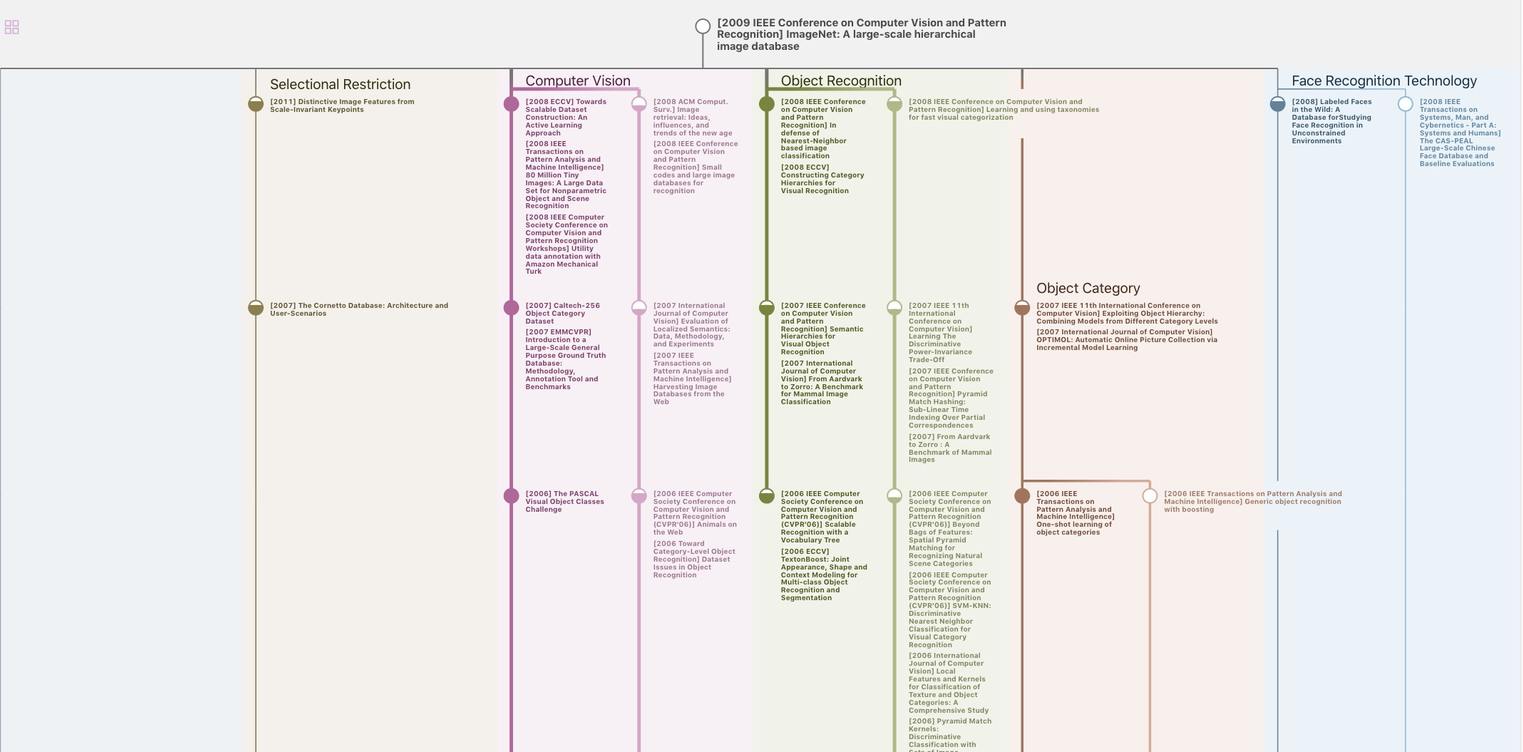
生成溯源树,研究论文发展脉络
Chat Paper
正在生成论文摘要