Using idea of three-step sparse residuals measurement to perform discriminant analysis
Soft Computing - A Fusion of Foundations, Methodologies and Applications(2014)
摘要
Classification of high-dimensional data is usually not amenable to standard pattern recognition techniques owing to lack of necessary, underlying structured information of data. In this paper, we propose a new discriminant analysis based on three-step sparse residuals measurement called DA-TSSR to address this problem. Specifically, in the first stage of the proposed method, the contribution in presenting the test sample of any chosen class is respectively calculated by adding up the total contributions of all the training samples of this class, and then a certain class with the smallest contribution score is eliminated from the set of the training samples. This procedure is iteratively carried out for the set of the training samples of the remaining classes till the predefined termination condition is satisfied. The second stage of DA-TSSR seeks to represent the test sample as a linear combination of all the remaining training samples and exploits the representation ability of each training sample to determine M “nearest neighbors” for the test sample. By this means, it generates unequal number of training samples on each candidate class. The third stage of DA-TSSR again determines a new weighted sum of all unequal numbers of training samples from candidate classes, which is approximately equal to the test sample. We use the new weighted sum to perform the designing of sparse residuals grades, which can be incorporated into the typical discriminant analysis criterion. The proposed method not only has a high accuracy but also can be clearly interpreted. Experimental results conducted on the ORL, XM2VTS, FERET and AR face databases demonstrate the effectiveness of the proposed method.
更多查看译文
关键词
Sparse residuals measurement, Discriminant analysis, Transform method, Image recognition
AI 理解论文
溯源树
样例
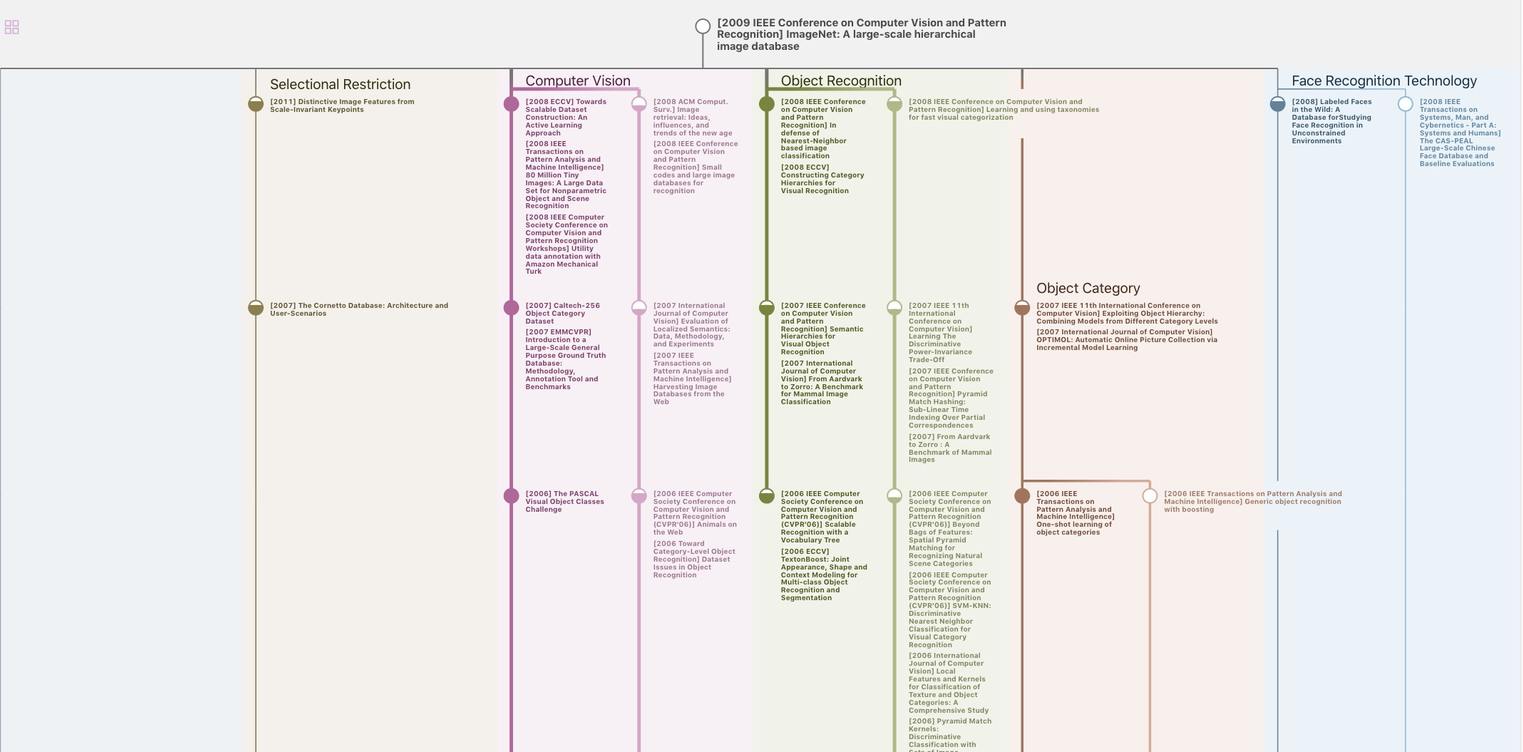
生成溯源树,研究论文发展脉络
Chat Paper
正在生成论文摘要