Recursive parameter and state estimation for an input nonlinear state space system using the hierarchical identification principle
Signal Processing(2015)
摘要
This paper focuses on the identification problem of an input nonlinear state space system with colored noise. Based on the observability canonical form, an identification model is derived and a state observer is designed. By using the hierarchical identification principle, a state observer based hierarchical stochastic gradient algorithm is presented for estimating the parameter vectors and states jointly. Furthermore, by using the multi-innovation identification theory, a state observer based hierarchical multi-innovation stochastic gradient algorithm is proposed for improving the convergence rate. The analysis indicates that the parameter estimates given by the proposed algorithms converge to the true values under persistent excitation conditions. Two numerical examples are offered to demonstrate the effectiveness of the proposed algorithms. HighlightsThe identification problem for nonlinear state space systems is considered.A state observer based hierarchical multi-innovation gradient algorithm is presented.The proposed algorithm can estimate the system states and parameters jointly.The convergence of the proposed algorithm is studied.
更多查看译文
关键词
Signal processing,State Filtering,Parameter estimation,Nonlinear system,State space model
AI 理解论文
溯源树
样例
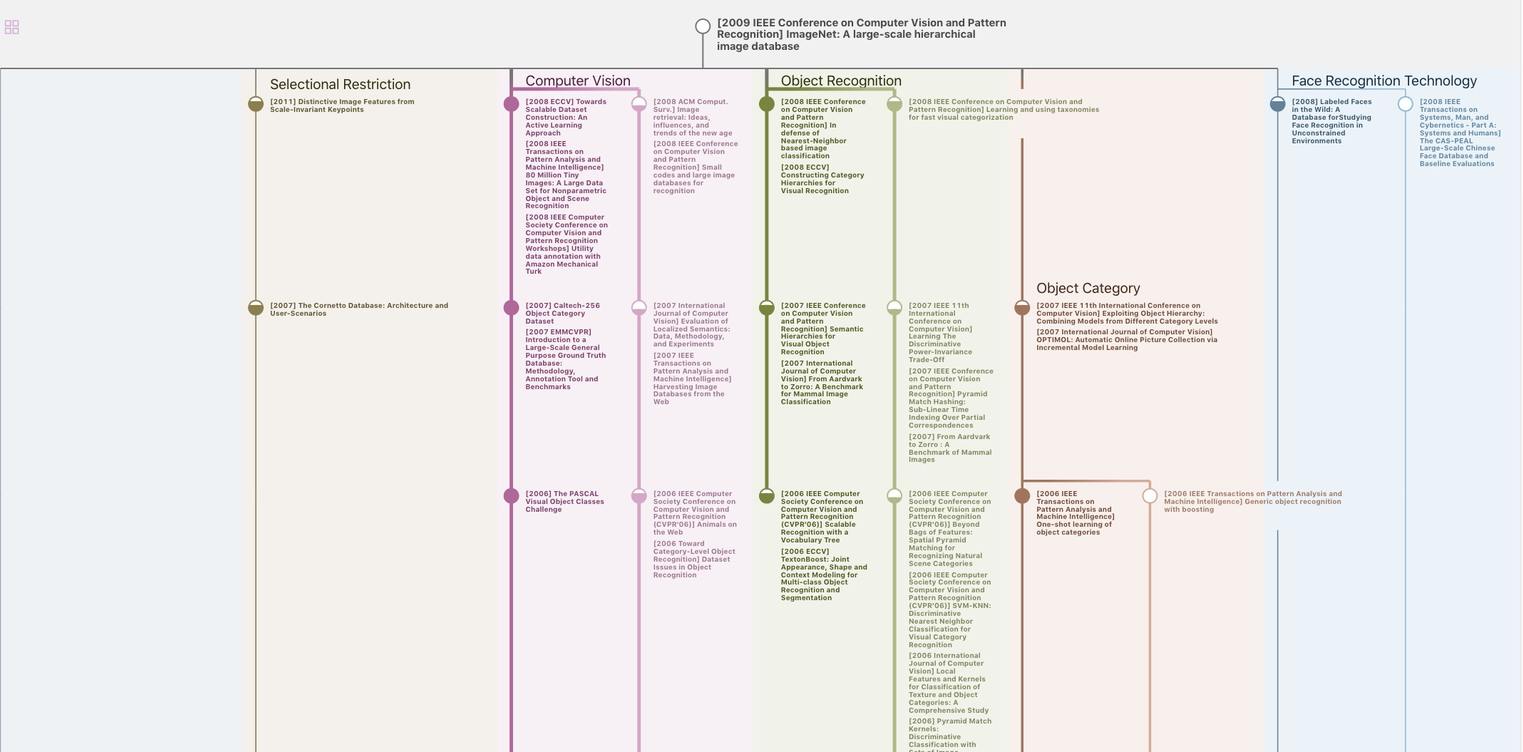
生成溯源树,研究论文发展脉络
Chat Paper
正在生成论文摘要