Multi-view based multi-label propagation for image annotation
Neurocomputing(2015)
摘要
Multi-view learning and multi-label propagation are two common approaches to address the problem of image annotation. Traditional multi-view methods disregard the consistencies among different views while existing algorithms toward multi-label propagation ignore the underlying mutual correlations among different labels. In this paper, we present a novel image annotation algorithm by exploring the heterogeneities from both the view level and the label level. For a single label, its propagation from one view should agree with the propagation from another view. Similarly, for a single view, the propagations of related labels should be similar. We call the proposed approach as Multi-view based Multi-label Propagation for image annotation (MMP). MMP handles the consistencies among different views by requiring them to generate the same annotation result, and captures the correlations among different labels by imposing the similarity constraints. By taking full advantage of the dual-heterogeneity from views and labels, MMP is able to propagate the labels better than state of the art. Furthermore, we introduce an iterative algorithm to solve the optimization problem. Extensive experiments on real image data have shown that the proposed framework has effective image annotation performance.
更多查看译文
关键词
Image annotation,Multi-view,Multi-label
AI 理解论文
溯源树
样例
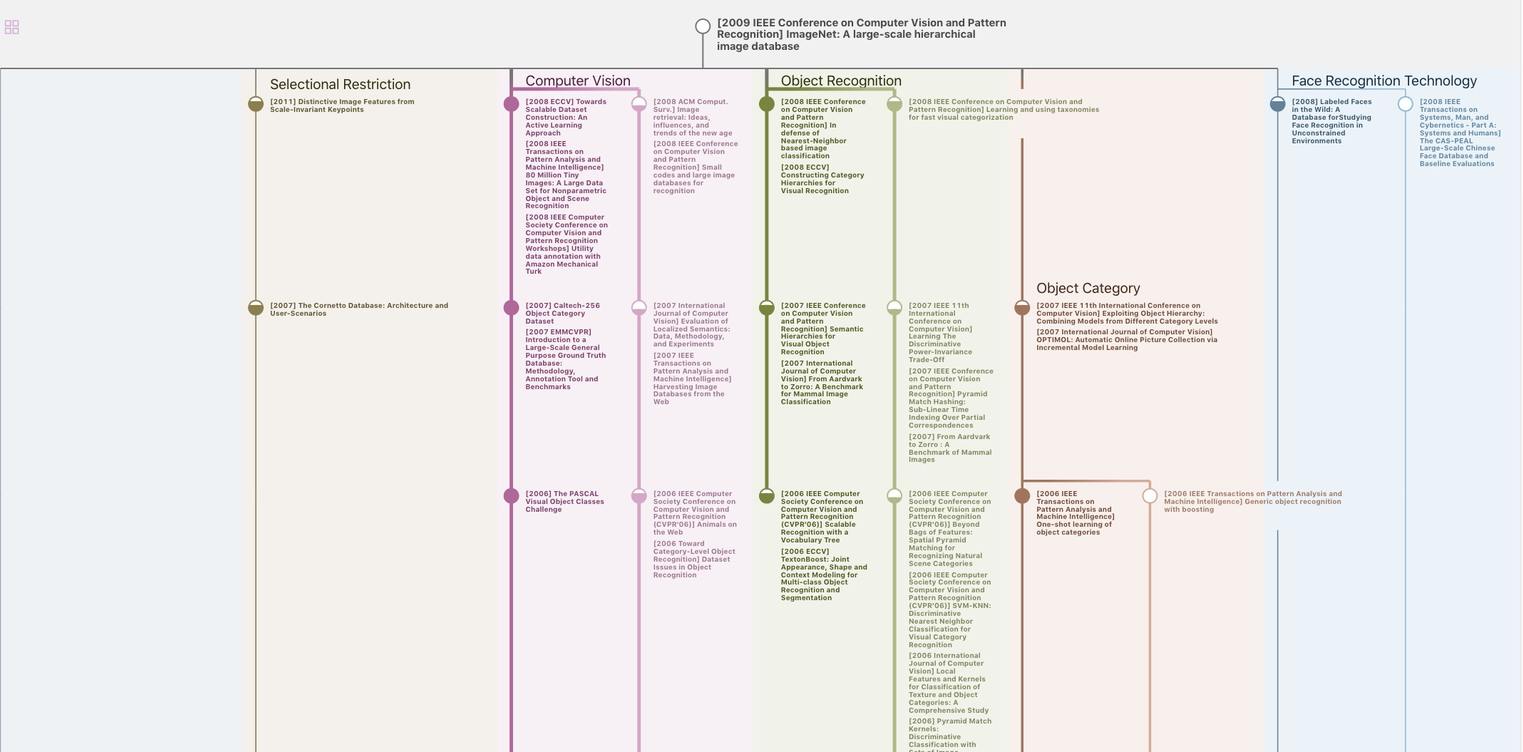
生成溯源树,研究论文发展脉络
Chat Paper
正在生成论文摘要