Incremental episodic segmentation and imitative learning of humanoid robot through self-exploration.
NEUROCOMPUTING(2016)
摘要
Imitation learning through self-exploration is an essential mechanism in developing sensorimotor skills for human infants as well for robots. We assume that a primitive sense of self is the prerequisite for successful social interaction rather than an outcome of it. During imitation learning, a crucial element of conception involves segmenting the continuous flow of motion into simpler units - motion primitives by identifying the boundaries of an action. Secondly, in realistic environment the robot must be able to learn the observed motion patterns incrementally in a stable adaptive manner without corrupting previously learned information. In this paper, we propose an on-line and unsupervised motion segmentation method allowing the robot to imitate and perform actions by observing the motion patterns performed by other partner through Incremental Kernel Slow Feature Analysis. The segmentation model directly operates on the images acquired from the robots vision sensor (camera) without requiring any kinematic model of the demonstrator. After segmentation, the spatio-temporal motion sequences are learned incrementally through Topological Gaussian Adaptive Resonance Hidden Markov Model. The learning model dynamically generates the topological structure in a self-organizing and self-stabilizing manner. Each node represents the encoded motion element (i.e. joint angles). The complete architecture was evaluated by simulation experiments performed on DARwIn-OP humanoid robot. (C) 2015 Elsevier B.V. All rights reserved.
更多查看译文
关键词
Robot behaviour learning,Adaptive Resonance Map,Gaussian distribution,Hidden Markov Model,Incremental learning,Topological map
AI 理解论文
溯源树
样例
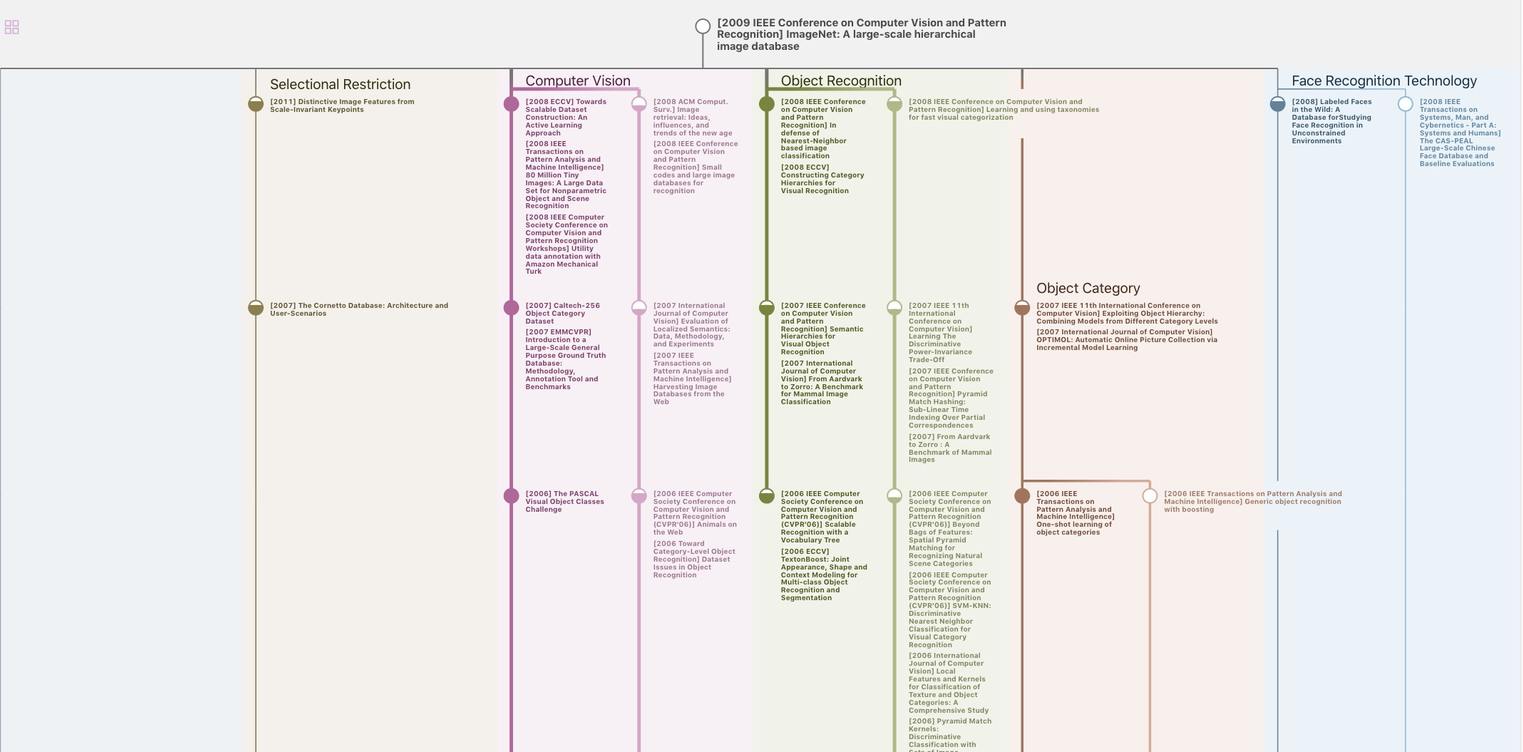
生成溯源树,研究论文发展脉络
Chat Paper
正在生成论文摘要