Patterns of Weber magnitude and orientation for uncontrolled face representation and recognition
Neurocomputing(2015)
摘要
Robust, discriminative and computationally efficient feature extraction is vital for a successful real-world face recognition system. To this end, we present a novel local feature descriptor, named patterns of Weber magnitude and orientation (PWMO), for face representation and recognition. Instead of merely taking advantage of the pixel intensity which is sensitive to variant impact factors such as illumination variations and noises, we describe a pixel with two robust statistic attributes of the local patch centered around it: the histogram of Weber magnitude and the dominant Weber orientation. By encoding them in a self-similarity manner with patch-based local binary patterns (p-LBP) and patch-based local XOR patterns (p-LXP) respectively, we obtain a robust representation for face images. To further enhance the discriminative power, we extend PWMO to its multi-scale version, and apply the block-based Fisher's linear discriminant (BFLD) to reduce the dimensionality and select the most discriminative features. The Fisher separation criterion (FSC) based block weighting scheme is incorporated for discriminative classification. We evaluate the proposed face representation method on two publicly available face databases: FERET and FRGC version 2.0 experiment 4 (FRGC-204). The recognition results demonstrate that the proposed method performs much better than most of the state-of-the-arts, and achieves comparable recognition performance with the recently proposed state-of-the-art algorithm based on the fusion of Gabor magnitude and phase, with only 1/7 storage requirement and 1/10 computational cost. (C) 2015 Elsevier B.V. All rights reserved.
更多查看译文
关键词
Face recognition,Feature extraction,Fisher's linear discriminant (FLD),Local binary patterns (LBP),Local XOR patterns (LXP),Weber's law
AI 理解论文
溯源树
样例
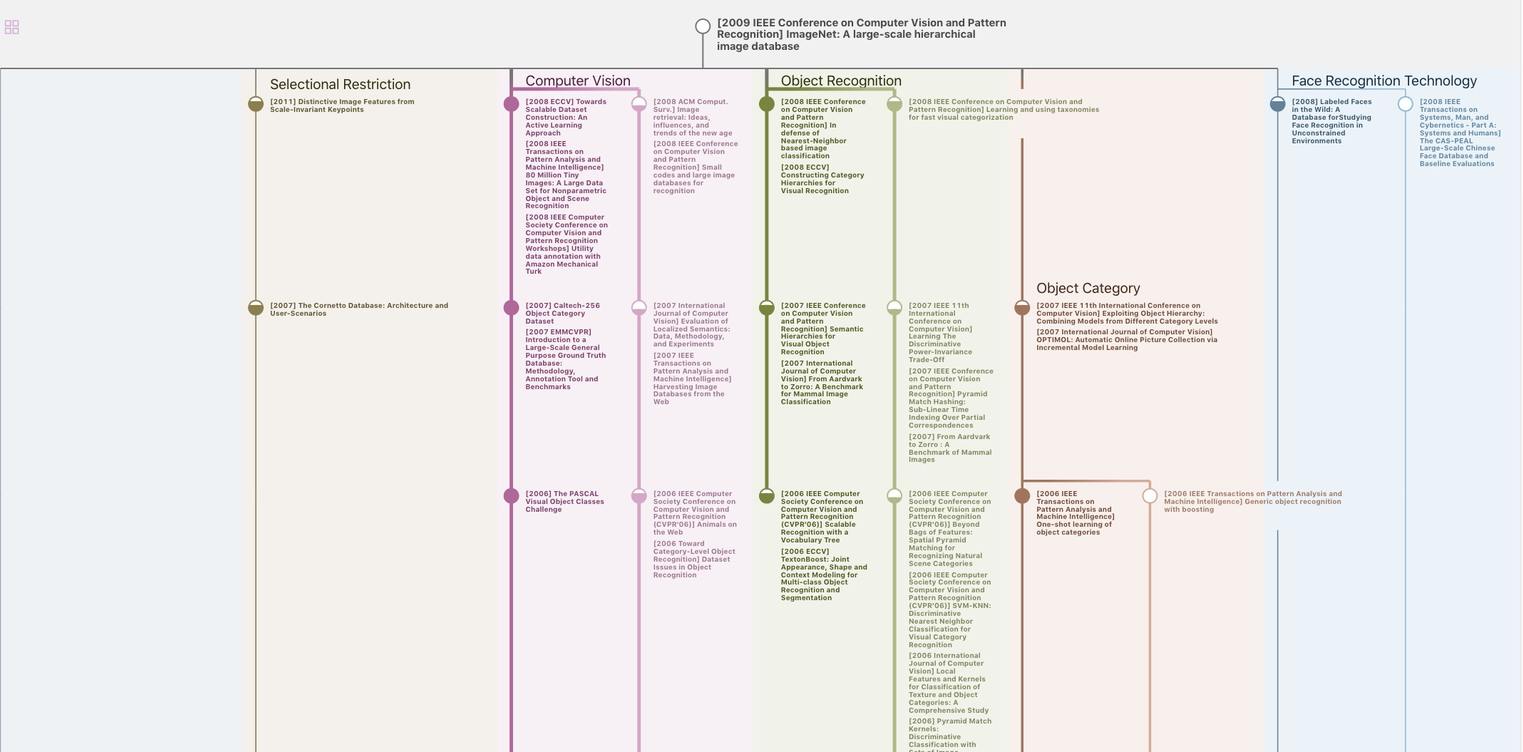
生成溯源树,研究论文发展脉络
Chat Paper
正在生成论文摘要