Action Recognition Based on Efficient Deep Feature Learning in the Spatio-Temporal Domain
IEEE Robotics and Automation Letters(2016)
摘要
Hand-crafted feature functions are usually designed based on the domain knowledge of a presumably controlled environment and often fail to generalize, as the statistics of real-world data cannot always be modeled correctly. Data-driven feature learning methods, on the other hand, have emerged as an alternative that often generalize better in uncontrolled environments. We present a simple, yet robust, 2-D convolutional neural network extended to a concatenated 3-D network that learns to extract features from the spatio-temporal domain of raw video data. The resulting network model is used for content-based recognition of videos. Relying on a 2-D convolutional neural network allows us to exploit a pretrained network as a descriptor that yielded the best results on the largest and challenging ILSVRC-2014 dataset. Experimental results on commonly used benchmarking video datasets demonstrate that our results are state-of-the-art in terms of accuracy and computational time without requiring any preprocessing (e.g., optic flow) or a priori knowledge on data capture (e.g., camera motion estimation), which makes it more general and flexible than other approaches. Our implementation is made available.
更多查看译文
关键词
Three-dimensional displays,Computational modeling,Feature extraction,Convolution,Robots,Data models,Optical imaging
AI 理解论文
溯源树
样例
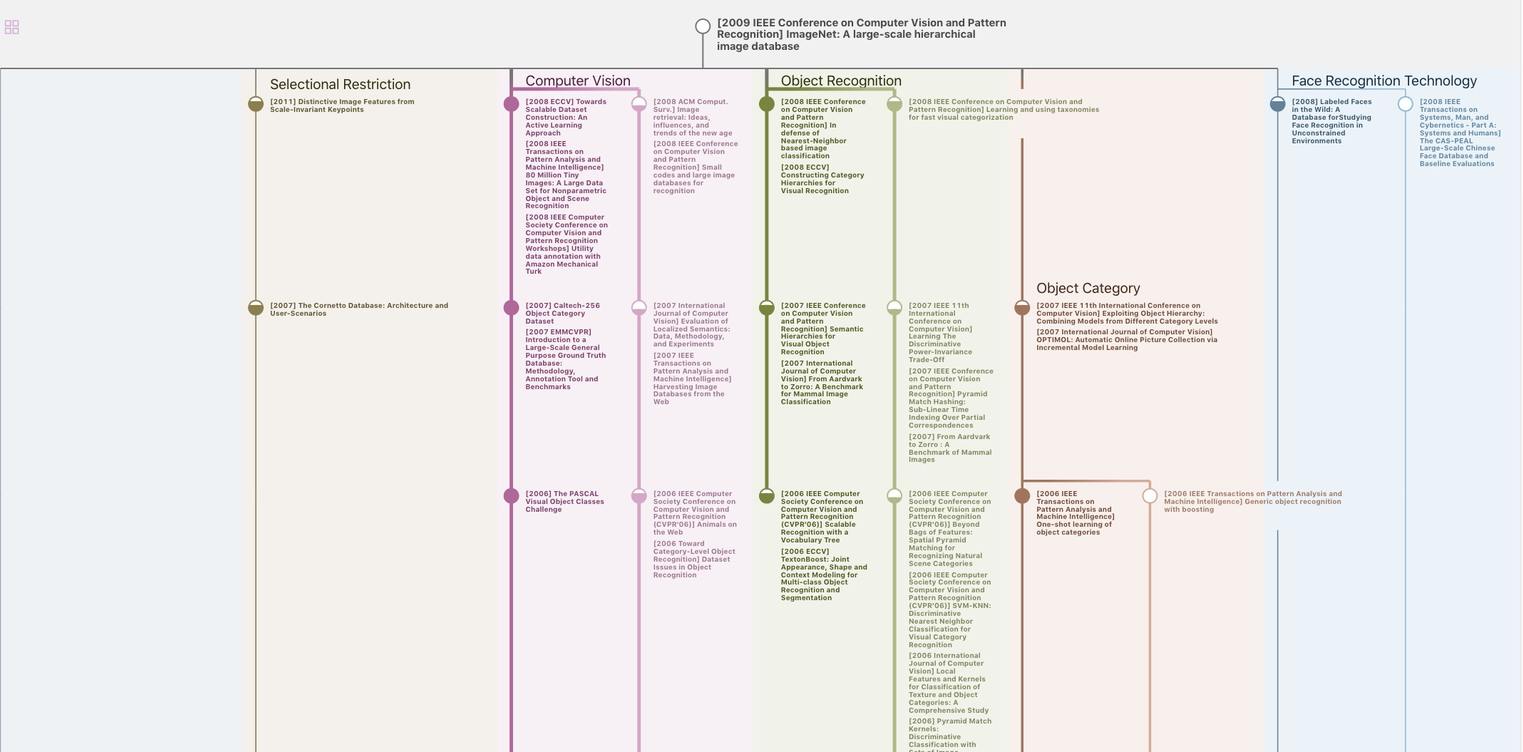
生成溯源树,研究论文发展脉络
Chat Paper
正在生成论文摘要