Identification Of Robust, Clinically Relevant Phenotypes Of Breast Cancer From Genome Scale Molecular Profiling
CANCER RESEARCH(2009)
摘要
CTRC-AACR San Antonio Breast Cancer Symposium: 2008 Abstracts
Abstract #2036
Genome scale analysis by microarrays or array CGH holds the promise to identify novel subclasses of breast cancer or characterize functional modules of clinical relevance in a robust fashion. However, the success of these endeavors is highly dependent on several factors such as the general noise structure and noise level of high throughput measurements or the strength of association between biomarkers/gene modules and clinical outcome. We will address two issues deeply rooted in the high throughput nature of genome scale profiling, which are relevant for the accurate analysis of clinical microarray data sets: systematic bias in clinical microarray data and establishing strategies that allow extracting robust, convergent information/classification from genome scale molecular profiling of breast cancer. As will be demonstrated, clinical microarray data are burdened with a high level of systematic bias. We identified sources of technical bias affecting many genes in concert, thus causing spurious correlations in clinical data sets and false associations between genes and clinical variables. A method will be presented to correct for technical bias in clinical microarray data, which increased concordance with known biological relationships in multiple data sets. Gene expression profiling based classification of breast cancer and prognostic or clinical response associated gene expression signatures are usually derived from a single data set. However, any result extracted from a single data set will reflect to a large extent the technical (which genes are measured reliably on the microarray) and biological (such as cohort selection) bias of the given cohort. An alternative approach uses multiple, in this case 5 different, analogous clinical data sets and determines the robust, convergent information emerging from the cross data-set analysis. We will present such a method, which will reduce the impact of data set specific bias and outline robust functional modules in breast cancer, ultimately leading to the reevaluation of gene expression profiling based subtyping and diagnostic gene expression signatures in breast cancer. Finally, we will present evidence for the existence of at least two fundamentally different types of genome instability in breast cancer with direct implications for response to chemotherapy.
Citation Information: Cancer Res 2009;69(2 Suppl):Abstract nr 2036.
更多查看译文
AI 理解论文
溯源树
样例
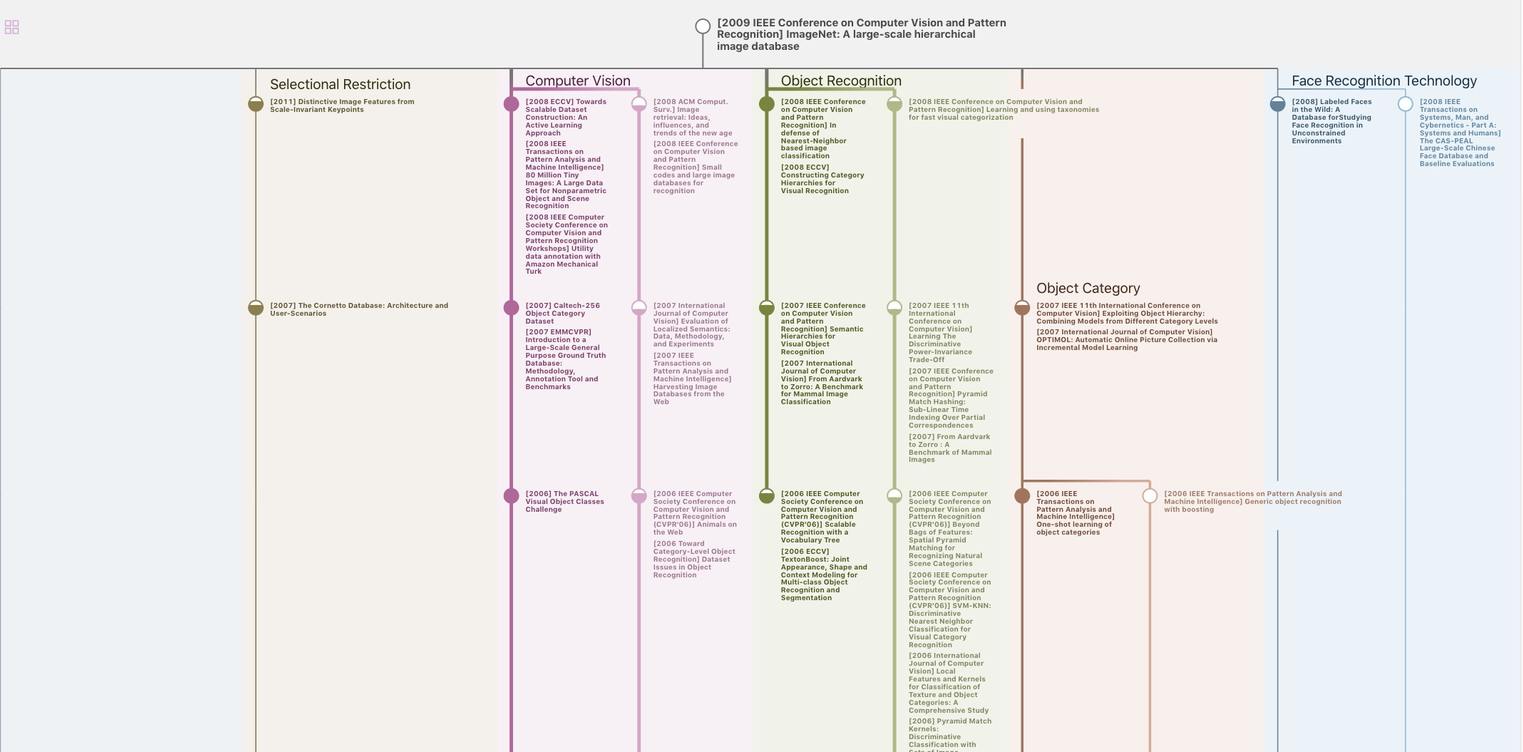
生成溯源树,研究论文发展脉络
Chat Paper
正在生成论文摘要