Learning to discount transformations as the computational goal of visual cortex
Nature Precedings(2011)
摘要
It has been long recognized that a key obstacle to achieving human-level object recognition performance is the problem of invariance. The human visual system excels at factoring out the image transformations that distort object appearance under natural conditions. Models with a cortex-inspired architecture such as HMAX as well as nonbiological convolutional neural networks are invariant to translation (and in some cases scaling) by virtue of their wiring. The transformations to which this approach has been applied so far are generic ; a single example image of any object contains all the information needed to synthesize a new image of the transformed object. In contrast, viewpoint and illumination transformations depend on the object's 3D structure and material properties. These are normally consistent within, but not between, classes.Class-specific modifications of the HMAX model achieve good viewpoint and illumination tolerant performance in a one-shot identification task. Performance suffers when a model that is specialized for transformations of one class is tested on identification within a different class. In fact, viewpoint-pooling models employing templates from the wrong class perform worse on viewpoint invariant identification tasks than models that have no particular mechanisms for dealing with viewpoint at all. The same situation arises for illumination invariance. This is in stark contrast to the generic case where the model is invariant to all classes undergoing the transformation no matter what templates are used.
更多查看译文
关键词
neuroscience
AI 理解论文
溯源树
样例
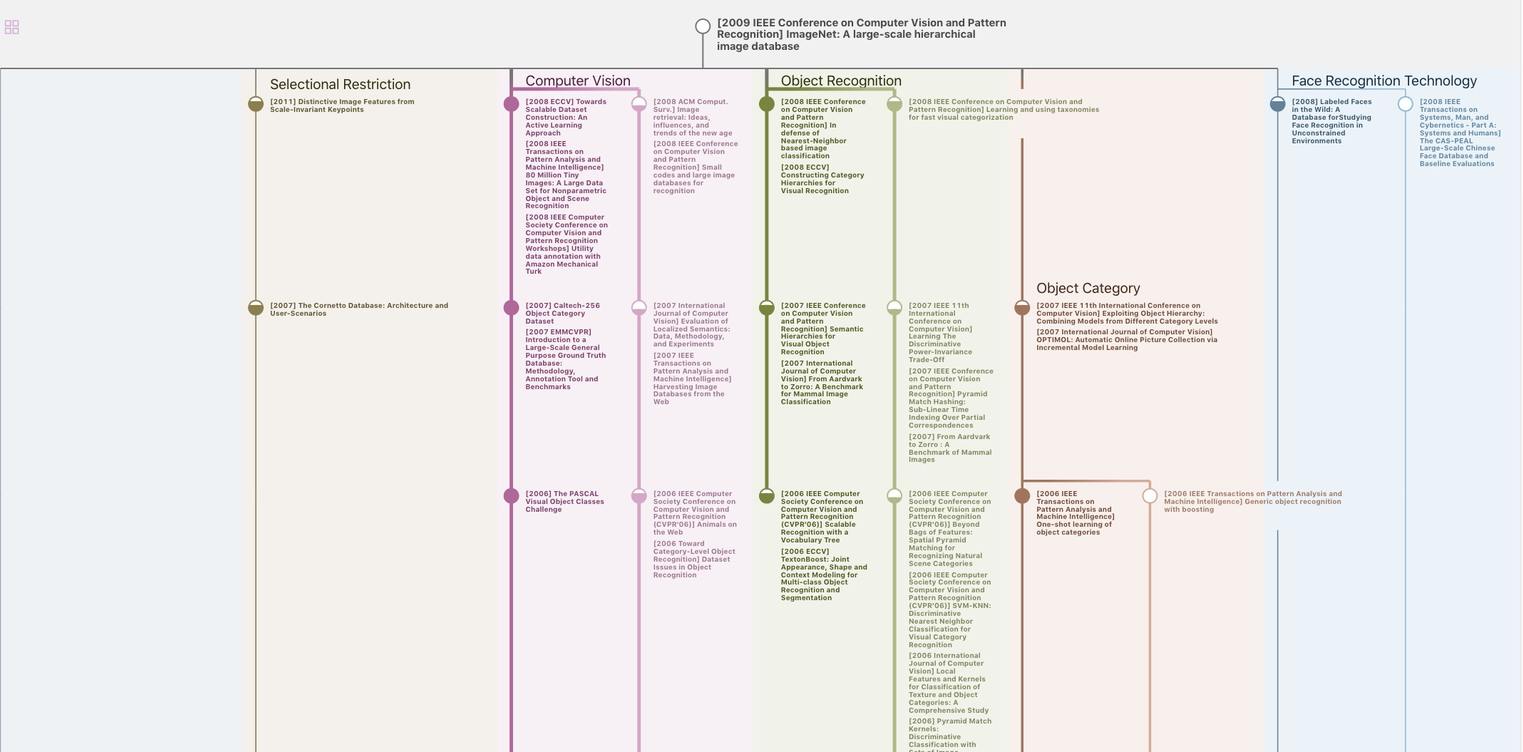
生成溯源树,研究论文发展脉络
Chat Paper
正在生成论文摘要