Learned Optimal Control of a Range Extender in a Series Hybrid Vehicle
ITSC(2015)
摘要
Each year, hybrid vehicles command a larger portion of total vehicles on the road. These vehicles combine multiple sources of energy, such as batteries and gasoline, which have different strengths and weaknesses. Active management of these energy sources can increase vehicle efficiency, longevity, or performance. Optimizing energy management is highly sensitive to upcoming power loads on the vehicle, but conventional control policies only react to the present state. Furthermore, these policies are computed at design-time and do not adapt to individual drivers. Advancements in cheap sensing and computation have enabled on-board learning and optimization that was previously impossible. In this work, we developed and implemented a real-time controller that exploits predictions computed from a dataset collected from other drivers. This data-driven controller manages a range-extender in a series gas-electric hybrid vehicle, optimizing fuel use, noise, and ignition frequency. The algorithm is scalable to large amounts of source data, and performance improves with prediction accuracy. We tested the algorithm in simulation and on a modified vehicle with direct programmatic control of the range extender. The experimental results on the vehicle reflected those observed in simulation, achieving fuel savings up to 12% and a noise-cost reduction of 73%.
更多查看译文
关键词
learned optimal control,range extender,batteries,gasoline,vehicle efficiency,vehicle longevity,vehicle performance,energy management optimization,cheap sensing,on-board learning,optimization,data-driven controller,series gas-electric hybrid vehicle,direct programmatic control,fuel savings,noise-cost reduction
AI 理解论文
溯源树
样例
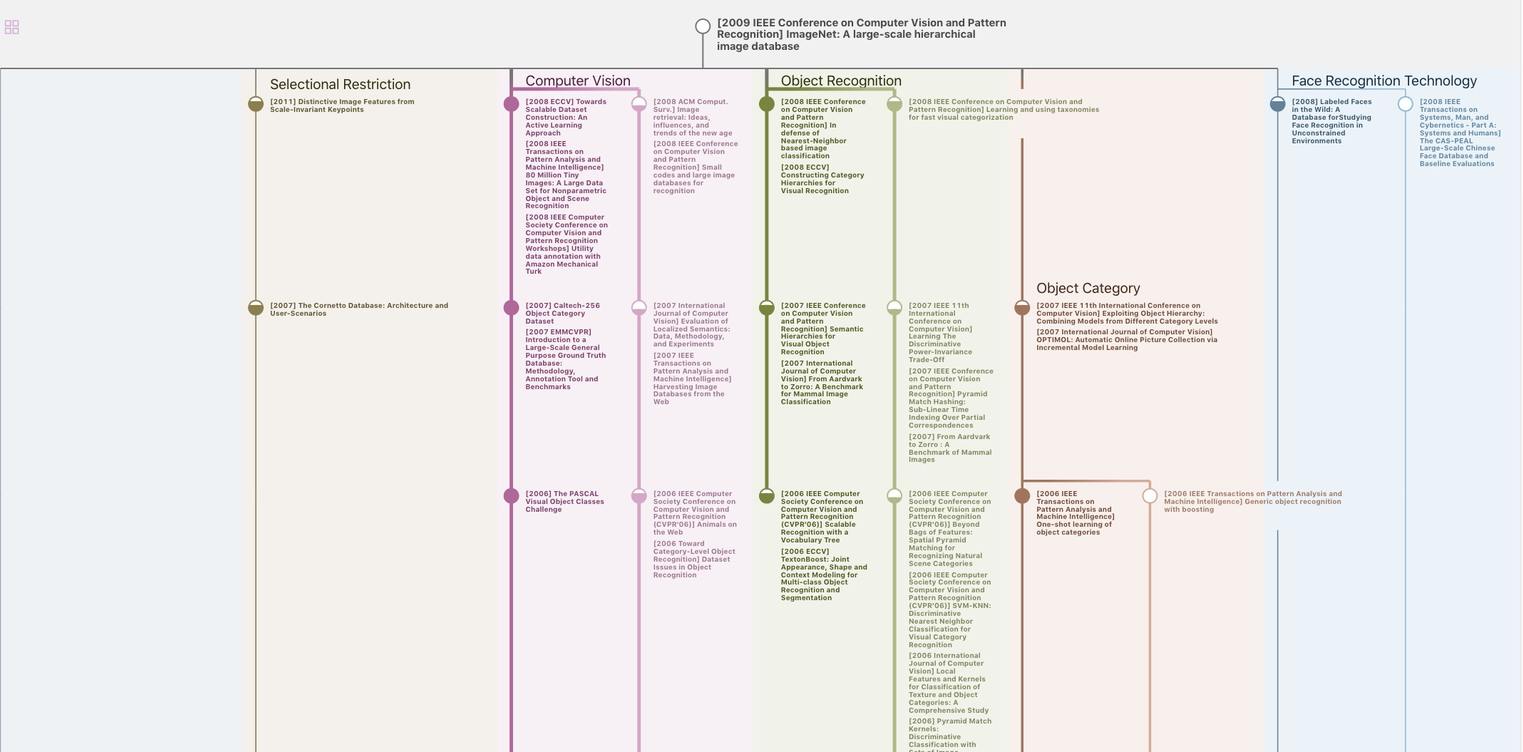
生成溯源树,研究论文发展脉络
Chat Paper
正在生成论文摘要