Topic Classification of Spoken Inquiries Using Transductive Support Vector Machine
Natural Interaction with Robots, Knowbots and Smartphones, Putting Spoken Dialog Systems into Practice(2014)
摘要
In this work, we address the topic classification of spoken inquiries in Japanese that are received by a guidance system operating in a real environment, with a semi-supervised learning approach based on a transductive support vector machine (TSVM). Manual data labeling, which is required for supervised learning, is a costly process, and unlabeled data are usually abundant and cheap to obtain. TSVM allows to treat partially labeled data for semi-supervised learning, including labeled and unlabeled samples in the training set. We are interested in evaluating the influence of including unlabeled samples in the training of the topic classification models, as well as the amount of them that could be necessary for improving performance. Experimental results show that this approach can be useful for taking advantage of unlabeled samples, especially when using larger unlabeled datasets. In particular, we found gains in classification performance for specific topics, such as city information, with a 6.30% F-measure improvement in the case of children’s inquiries and 7.63% for access information in the case of adults’ inquiries.
更多查看译文
关键词
Classification Performance, Automatic Speech Recognition, Unlabeled Data, Unlabeled Sample, Improve Classification Performance
AI 理解论文
溯源树
样例
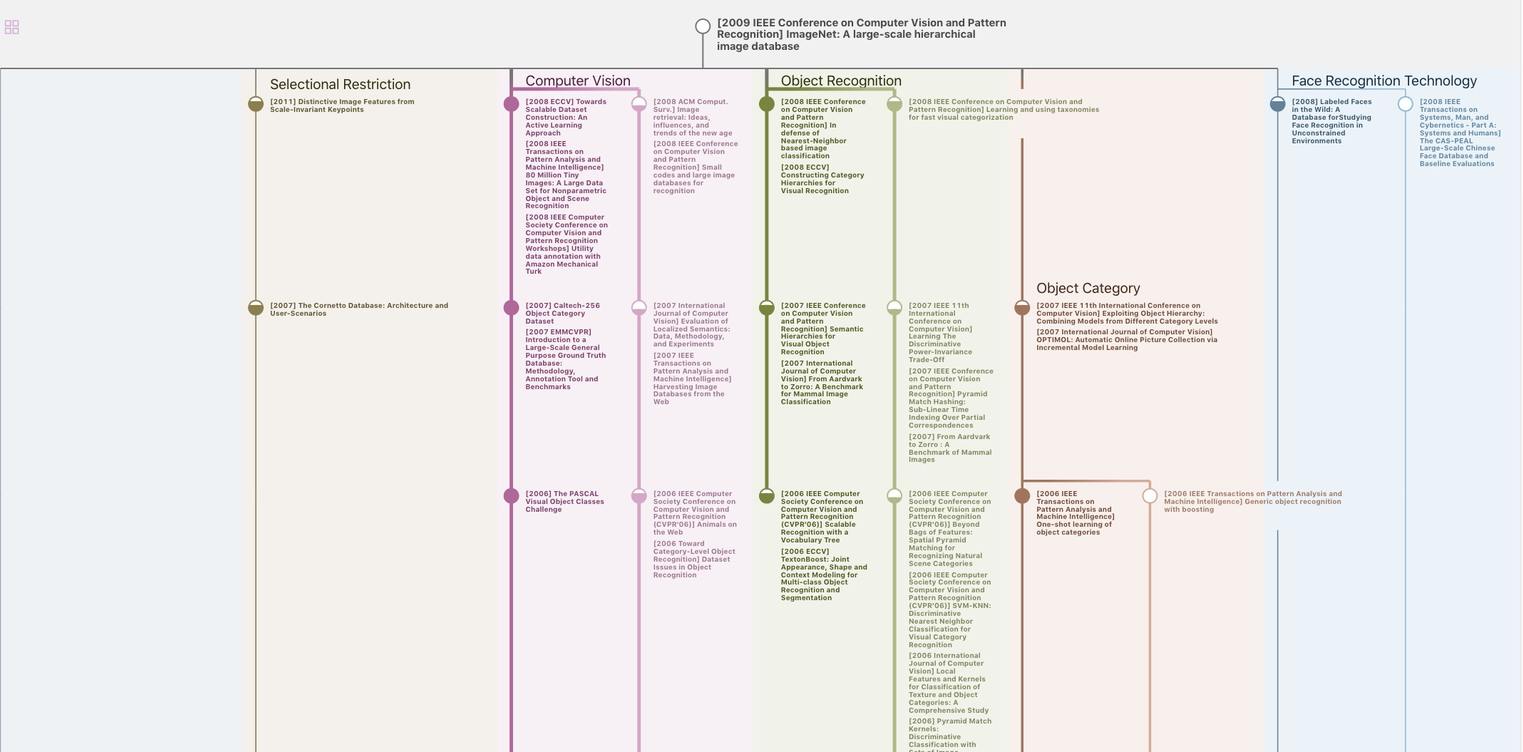
生成溯源树,研究论文发展脉络
Chat Paper
正在生成论文摘要