Denoising X-ray CT images based on product Gaussian mixture distribution models for original and noise images
TENCON IEEE Region 10 Conference Proceedings(2010)
摘要
An adaptive Wiener filter for denoising X-ray CT image has been proposed based on the universal Gaussian mixture distribution model (UNI-GMM). In this method, the UNI-GMM is estimated by the statistical learning method using two sets of pari images, one of which is an observed (low dose) X-ray CT image set and the other is an original (high dose) X-ray CT image set. Owing to the physical limitations of CT scanners, the original (high dose) X-ray CT image also includes considerable noise that prevented precise learning of the UNI-GMM. On the other hand, the noise included in the X-ray CT images is the specific artifact which is called streak artifact and is known to be statistically non-stationary. In the previously proposed method, the artifact is treated to be stationary for simplicity. Thus the restored images include residual noise due to the non-stationary noise. In this paper, the UNI-GMM method is improved by a two stages product modeling. First, the UNI-GMM for the original images is estimated using a low noise natural image set that include scenes, portraits and still pictures, to prevent the effect of noise on the original (high dose) CT images. Second, the UNI-GMM for the noise images is estimated using a noise image set casted by subtracting the original X-ray CT images from the observed X-ray CT images. Simulation results show that the proposed product UNI-GMMs performs better than the conventional stationary noise model simply learned using X-ray CT images.
更多查看译文
关键词
wiener filter,learning artificial intelligence,gaussian distribution,statistical analysis
AI 理解论文
溯源树
样例
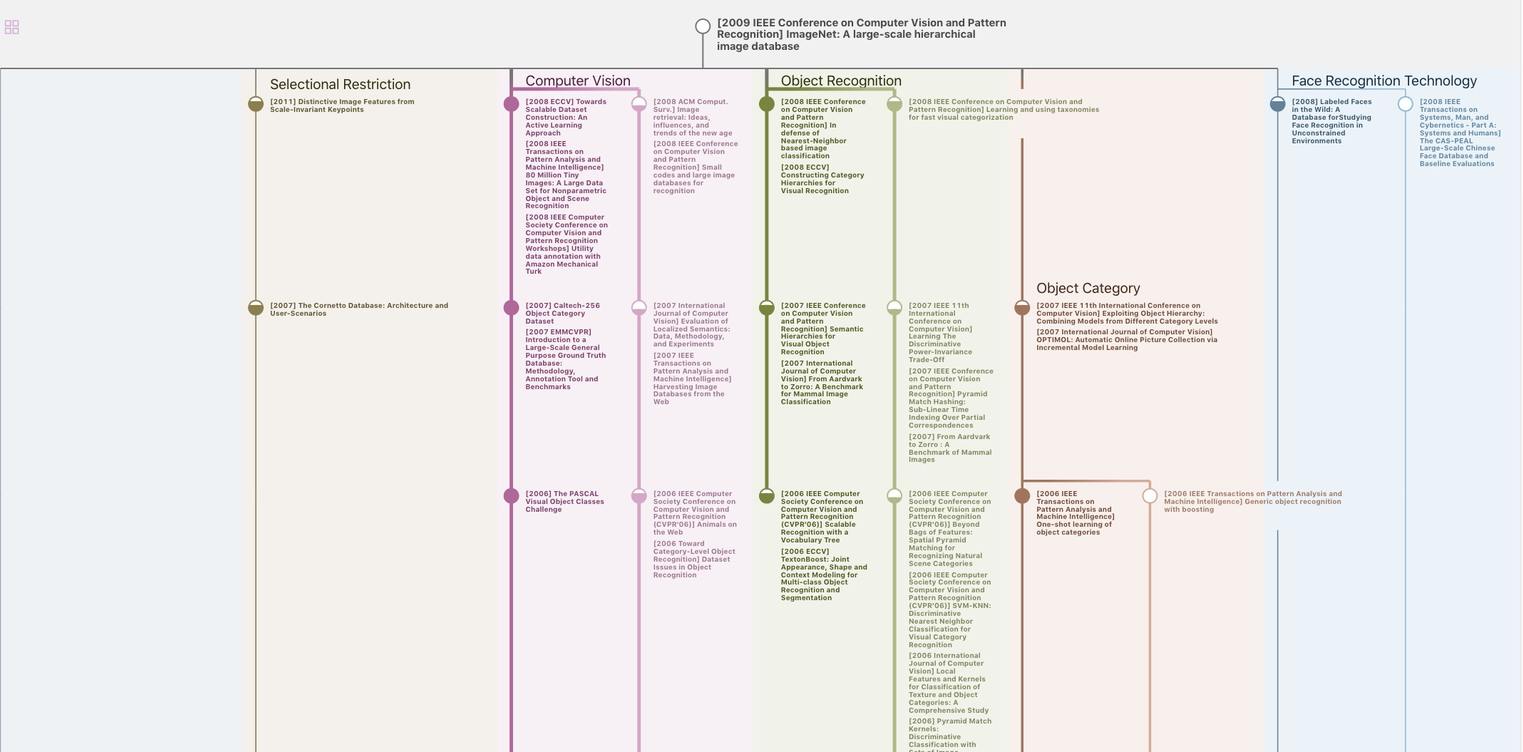
生成溯源树,研究论文发展脉络
Chat Paper
正在生成论文摘要