Novelty Based Learning of Primitive Manipulation Strategies
international conference on robotics and automation(2012)
摘要
A novelty based learning system which self generates p rimitive actions that can be used to form more complex manipulations is presented. In the field of intelligent robotics research, manipulation capability is important as it enables complex, yet delicate and precise interactions with the environment. Thus far, manipulation research has made only minor progress in developing general manipulation techniques. In order to provide the flexibility to adapt to unstructured environments, robotic manipulation systems will require an autonomous learning capability. Conventional machine learning algorithms, such as various forms of neural networks, reinforcement learning and decision tree’s, have provided good results in previous research for relatively simple tasks. However for increasingly more diverse and complex tasks, these algorithms lack adaptability and expandability and as such often yield poor performance. A relatively new field of machine learning for intelligent robotics modelled on human behaviour is evolving which has promising potential for manipulation applications. Motivated or novelty based learning uses interesting occurrences or outcomes to direct and focus learning towards such observations of interest. In this project this concept is applied to a simplified robotic manipulation system so that primitive actions can be learned. It is intended that these primitive actions will then be combined to form more complex actions to complete a given task.
更多查看译文
AI 理解论文
溯源树
样例
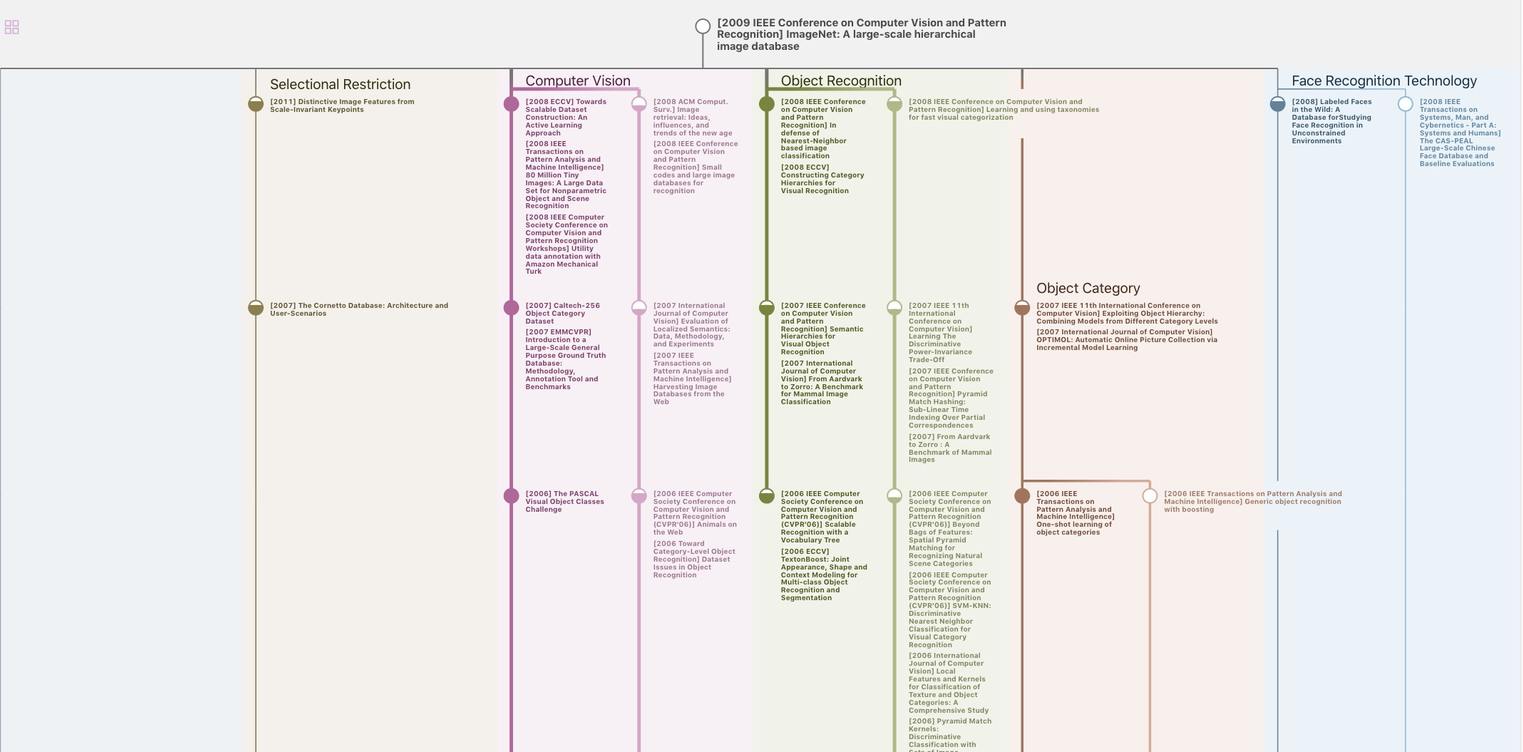
生成溯源树,研究论文发展脉络
Chat Paper
正在生成论文摘要