Modulating Communication to Improve Multi-agent Learning Convergence
mag(2013)
摘要
The problem of getting interacting agents to learn simultaneously to improve their joint performance has received significant attention in the literature. One of the key challenges is to manage the system-wide effects that occur due to learning in a non-stationary environment. In this paper, we look at the impact on the system-wide dynamics and the learning convergence due to communication between the agents. Specifically, we look at the problem of learning routes between locations in a graph in the case where agents using the same edge at the same time slow each other down. We implemented and empirically examined a model where the agents simply try to model each edge in the graph as being either slow, medium, or fast due to the other agents using that edge. Communication on a fixed social network occurs only when an agent changes the speed category it has for a particular link, e.g., when it changes from believing a link is slow to believing it is medium. We find that the system dynamics are very sensitive to the ratio between the influence of direct observations on local beliefs to the influence of communicated beliefs. For some values of this ratio, convergence to good behavior can occur very quickly, but for others a brief period of good performance is followed by wild oscillations.
更多查看译文
关键词
Multi-agent learning, Information sharing, Congestion games, Social networks
AI 理解论文
溯源树
样例
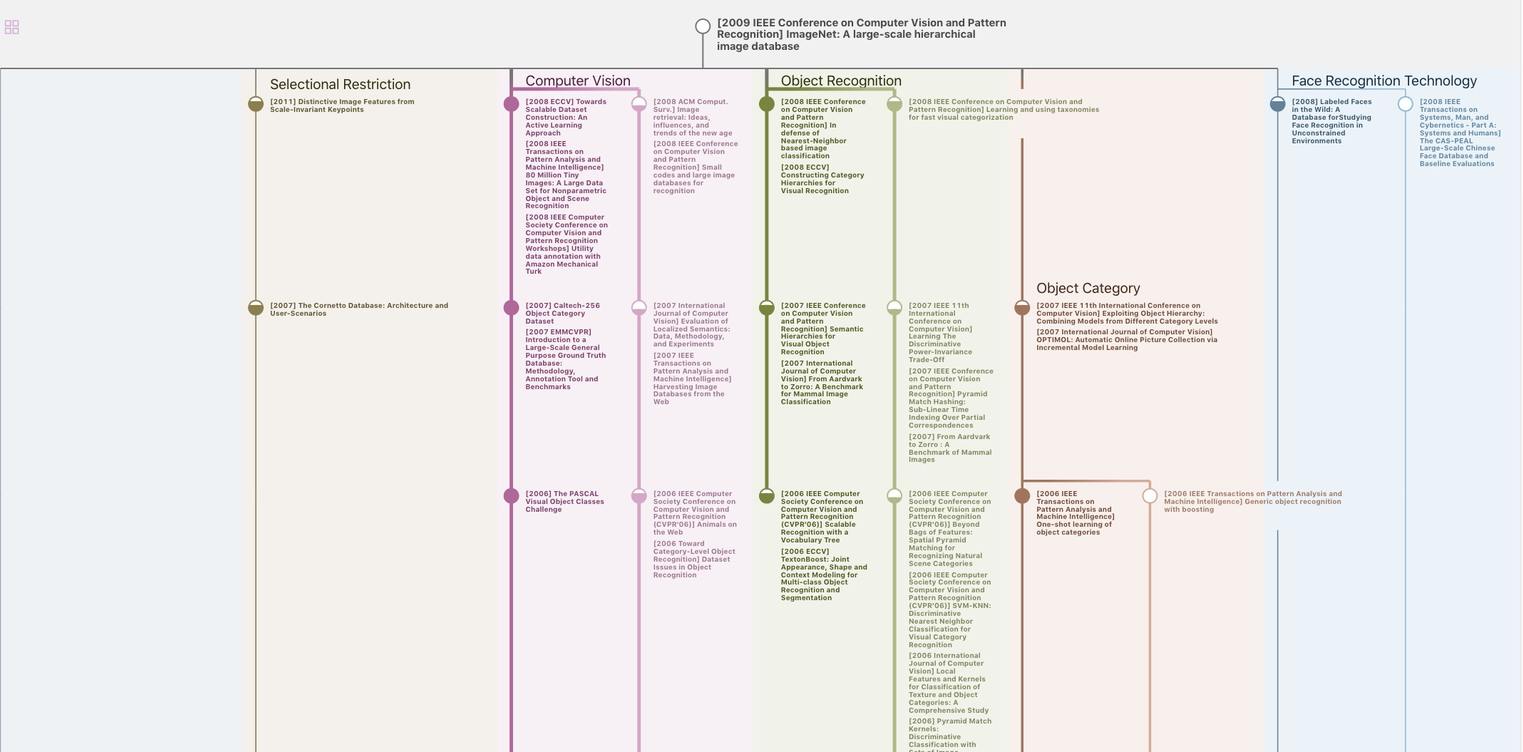
生成溯源树,研究论文发展脉络
Chat Paper
正在生成论文摘要