Robust Lane Detection Using Two-stage Feature Extraction with Curve Fitting
Pattern Recognition(2016)
摘要
With the increase in the number of vehicles, many intelligent systems have been developed to help drivers to drive safely. Lane detection is a crucial element of any driver assistance system. At present, researchers working on lane detection are confronted with several major challenges, such as attaining robustness to inconsistencies in lighting and background clutter. To address these issues in this work, we propose a method named Lane Detection with Two-stage Feature Extraction (LDTFE) to detect lanes, whereby each lane has two boundaries. To enhance robustness, we take lane boundary as collection of small line segments. In our approach, we apply a modified HT (Hough Transform) to extract small line segments of the lane contour, which are then divided into clusters by using the DBSCAN (Density Based Spatial Clustering of Applications with Noise) clustering algorithm. Then, we can identify the lanes by curve fitting. The experimental results demonstrate that our modified HT works better for LDTFE than LSD (Line Segment Detector). Through extensive experiments, we demonstrate the outstanding performance of our method on the challenging dataset of road images compared with state-of-the-art lane-detection methods. HighlightsWe proposed a novel lane detection method.Our method regards lane boundary as collection of small line segments.We proposed a modified Hough Transform to detect small line segments.Small line segments are clustered based on our proposed similarity measurement.Removing interferential clusters depends on the balance of small line segments.
更多查看译文
关键词
hough transform,curve fitting,cluster
AI 理解论文
溯源树
样例
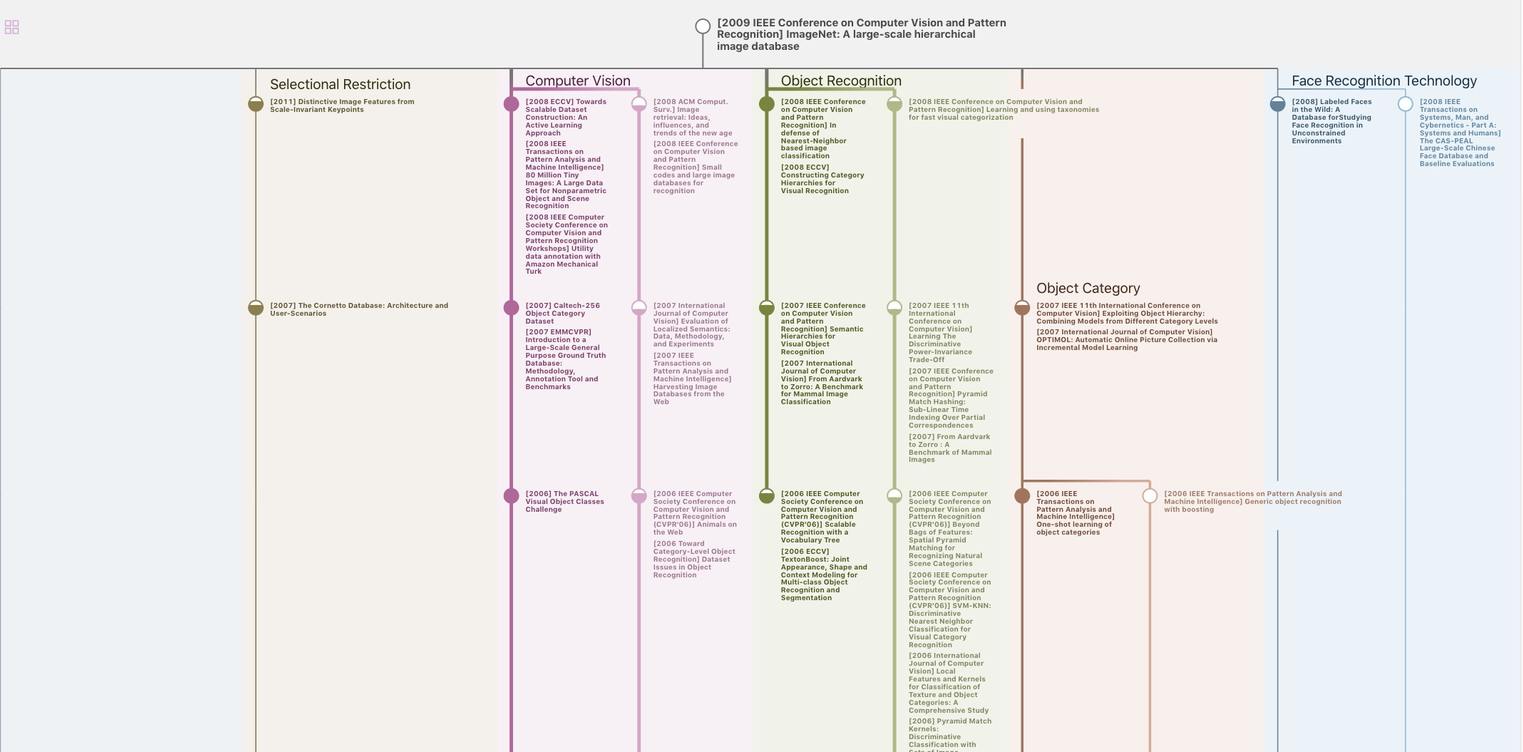
生成溯源树,研究论文发展脉络
Chat Paper
正在生成论文摘要