Generating Pit-free Canopy Height Models from Airborne Lidar
Photogrammetric Engineering and Remote Sensing(2014)
摘要
Canopy height models (aims) derived from lidar data have been applied to extract forest inventory parameters. However, variations in modeled height cause data pits, which form a challenging problem as they disrupt CHM smoothness, negatively affecting tree detection and subsequent biophysical measurements. These pits appear where laser beams penetrate deeply into a tree crown, hitting a lower branch or the ground before producing the first return. In this study, we develop a new algorithm that generates a pit-free CHM raster, by using subsets of the lidar points to close pits. The algorithm operates robustly on high-density lidar data as well as on a thinned lidar dataset. The evaluation involves detecting individual trees using the pit-free CHM and comparing the findings to those achieved by using a Gaussian smoothed CHM. The results show that our pit-free aims derived from high- and low-density lidar data significantly improve the accuracy of tree detection.
更多查看译文
AI 理解论文
溯源树
样例
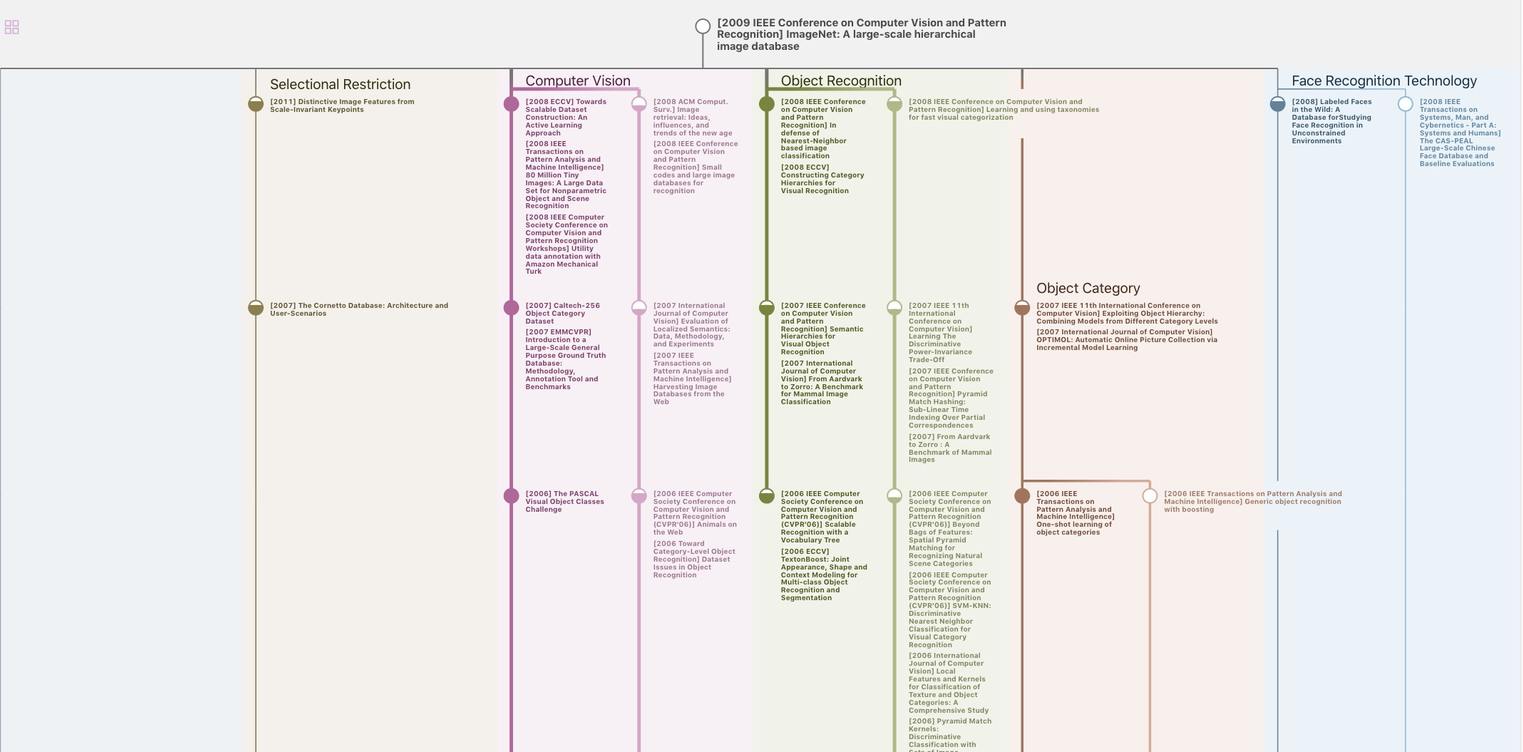
生成溯源树,研究论文发展脉络
Chat Paper
正在生成论文摘要