Multi trajectory pose adjustment for life-long mapping
Intelligent Vehicles Symposium(2015)
摘要
State of the art highly automated and self-driving vehicles heavily depend on detailed maps since they free the system from many otherwise complex onboard processing tasks. However, depending on the environment and the fineness of the map, the validity span of maps is often short and a periodic remapping of large areas with sensor-packed mapping vehicles is beyond any feasibility. Crowd based mapping approaches using low cost sensors appear more practicable. Herein we propose a general method to align several trajectories of the same area which is fundamental for any life-long mapping. Our algorithm requires previously acquired pose differences as input. These differences induce a pose graph which is aligned yielding a minimum least-squares residual. Therefore, our method is independent from the underlying sensor technology. For evaluation purposes, we align pose graphs from simulated pose differences and compare it against the ground truth. Furthermore, stereo cameras are used to obtain pose difference estimates by common visual odometry methods. We present quantitative results of the robustness and accuracy of our method based on these pose differences. The results are compared against a high precision GPS receiver. Our approach clearly outperforms this costly reference sensor.
更多查看译文
关键词
Global Positioning System,graph theory,intelligent transportation systems,least squares approximations,pose estimation,road vehicles,stereo image processing,GPS receiver,automated vehicles,crowd based mapping,detailed maps,life-long mapping,minimum least-squares residual,multitrajectory pose adjustment,pose difference estimates,pose graph,self-driving vehicles,sensor-packed mapping vehicles,stereo cameras,visual odometry methods
AI 理解论文
溯源树
样例
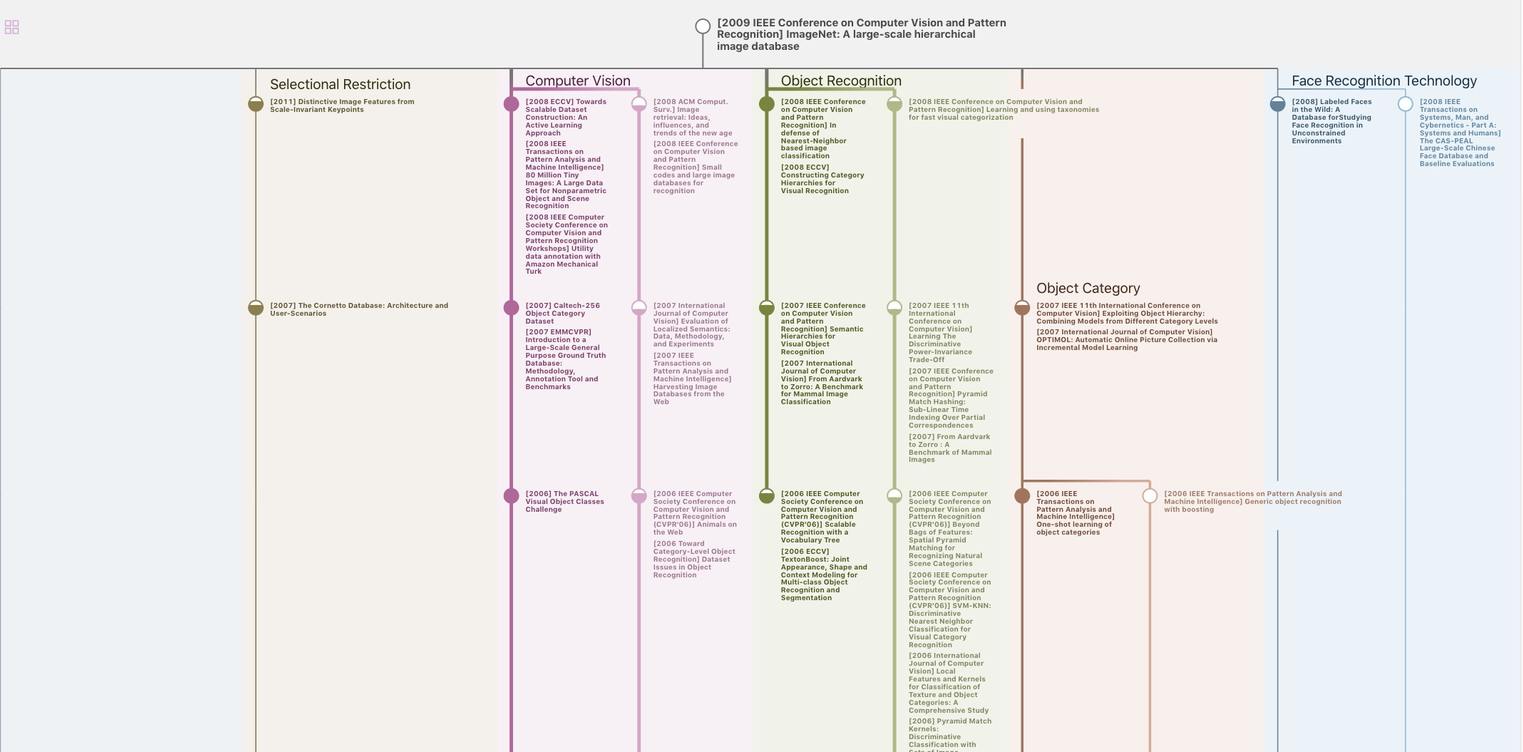
生成溯源树,研究论文发展脉络
Chat Paper
正在生成论文摘要