Application of neural networks with back-propagation to genome-enabled prediction of complex traits in Holstein-Friesian and German Fleckvieh cattle
Genetics, selection, evolution : GSE(2015)
摘要
Background Recently, artificial neural networks (ANN) have been proposed as promising machines for marker-based genomic predictions of complex traits in animal and plant breeding. ANN are universal approximators of complex functions, that can capture cryptic relationships between SNPs (single nucleotide polymorphisms) and phenotypic values without the need of explicitly defining a genetic model. This concept is attractive for high-dimensional and noisy data, especially when the genetic architecture of the trait is unknown. However, the properties of ANN for the prediction of future outcomes of genomic selection using real data are not well characterized and, due to high computational costs, using whole-genome marker sets is difficult. We examined different non-linear network architectures, as well as several genomic covariate structures as network inputs in order to assess their ability to predict milk traits in three dairy cattle data sets using large-scale SNP data. For training, a regularized back propagation algorithm was used. The average correlation between the observed and predicted phenotypes in a 20 times 5-fold cross-validation was used to assess predictive ability. A linear network model served as benchmark. Results Predictive abilities of different ANN models varied markedly, whereas differences between data sets were small. Dimension reduction methods enhanced prediction performance in all data sets, while at the same time computational cost decreased. For the Holstein-Friesian bull data set, an ANN with 10 neurons in the hidden layer achieved a predictive correlation of r =0.47 for milk yield when the entire marker matrix was used. Predictive ability increased when the genomic relationship matrix ( r =0.64) was used as input and was best ( r =0.67) when principal component scores of the marker genotypes were used. Similar results were found for the other traits in all data sets. Conclusion Artificial neural networks are powerful machines for non-linear genome-enabled predictions in animal breeding. However, to produce stable and high-quality outputs, variable selection methods are highly recommended, when the number of markers vastly exceeds sample size.
更多查看译文
关键词
Artificial Neural Network,Hide Layer,Predictive Ability,Artificial Neural Network Model,Artificial Neural Network Architecture
AI 理解论文
溯源树
样例
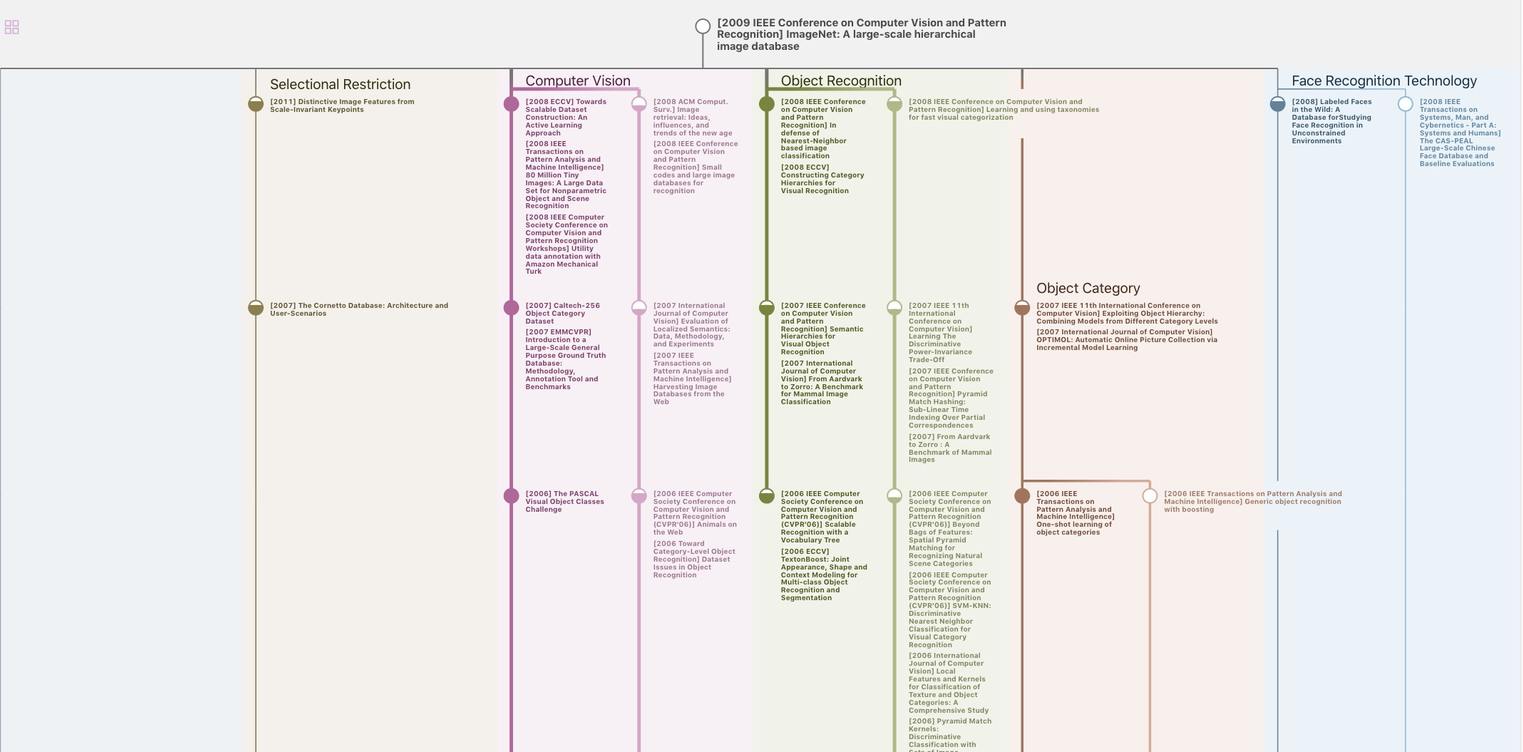
生成溯源树,研究论文发展脉络
Chat Paper
正在生成论文摘要