CEPR: A Collaborative Exploration and Periodically Returning Model for Location Prediction
ACM Transactions on Intelligent Systems and Technology (TIST)(2015)
摘要
With the growing popularity of location-based social networks, numerous location visiting records (e.g., check-ins) continue to accumulate over time. The more these records are collected, the better we can understand users’ mobility patterns and the more accurately we can predict their future locations. However, due to the personality trait of neophilia, people also show propensities of novelty seeking in human mobility, that is, exploring unvisited but tailored locations for them to visit. As such, the existing prediction algorithms, mainly relying on regular mobility patterns, face severe challenges because such behavior is beyond the reach of regularity. As a matter of fact, the prediction of this behavior not only relies on the forecast of novelty-seeking tendency but also depends on how to determine unvisited candidate locations. To this end, we put forward a Collaborative Exploration and Periodically Returning model (CEPR), based on a novel problem, Exploration Prediction (EP), which forecasts whether people will seek unvisited locations to visit, in the following. When people are predicted to do exploration, a state-of-the-art recommendation algorithm, armed with collaborative social knowledge and assisted by geographical influence, will be applied for seeking the suitable candidates; otherwise, a traditional prediction algorithm, incorporating both regularity and the Markov model, will be put into use for figuring out the most possible locations to visit. We then perform case studies on check-ins and evaluate them on two large-scale check-in datasets with 6M and 36M records, respectively. The evaluation results show that EP achieves a roughly 20% classification error rate on both datasets, greatly outperforming the baselines, and that CEPR improves performances by as much as 30% compared to the traditional location prediction algorithms.
更多查看译文
关键词
algorithms,clustering,data mining,design,experimentation,exploration prediction,information filtering,location prediction,location recommendation,location-based services,social network,spatial databases and gis,location based services
AI 理解论文
溯源树
样例
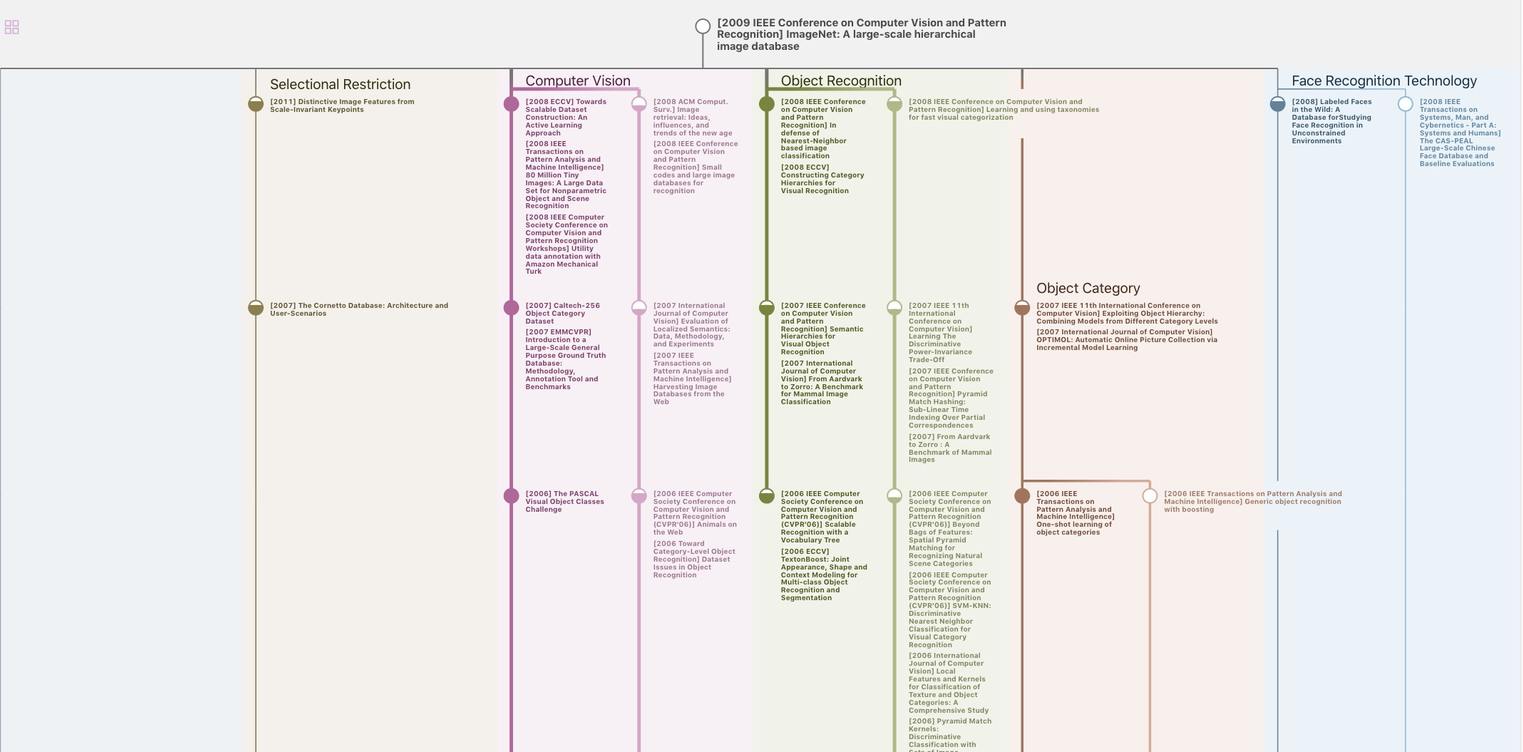
生成溯源树,研究论文发展脉络
Chat Paper
正在生成论文摘要