t-Closeness through Microaggregation: Strict Privacy with Enhanced Utility Preservation
ICDE(2016)
摘要
Microaggregation is a technique for disclosure limitation aimed at protecting the privacy of data subjects in microdata releases. It has been used as an alternative to generalization and suppression to generate k-anonymous data sets, where the identity of each subject is hidden within a group of k subjects. Unlike generalization, microaggregation perturbs the data and this additional masking freedom allows improving data utility in several ways, such as increasing data granularity, reducing the impact of outliers and avoiding discretization of numerical data. k-Anonymity, on the other side, does not protect against attribute disclosure, which occurs if the variability of the confidential values in a group of k subjects is too small. To address this issue, several refinements of k-anonymity have been proposed, among which t-closeness stands out as providing one of the strictest privacy guarantees. Existing algorithms to generate t-close data sets are based on generalization and suppression (they are extensions of k-anonymization algorithms based on the same principles). This paper proposes and shows how to use microaggregation to generate k-anonymous t-close data sets. The advantages of microaggregation are analyzed, and then several microaggregation algorithms for k-anonymous t-closeness are presented and empirically evaluated.
更多查看译文
关键词
data privacy,k-anonymity,microaggregation,t-closeness,clustering algorithms,merging,data models,algorithm design and analysis,privacy
AI 理解论文
溯源树
样例
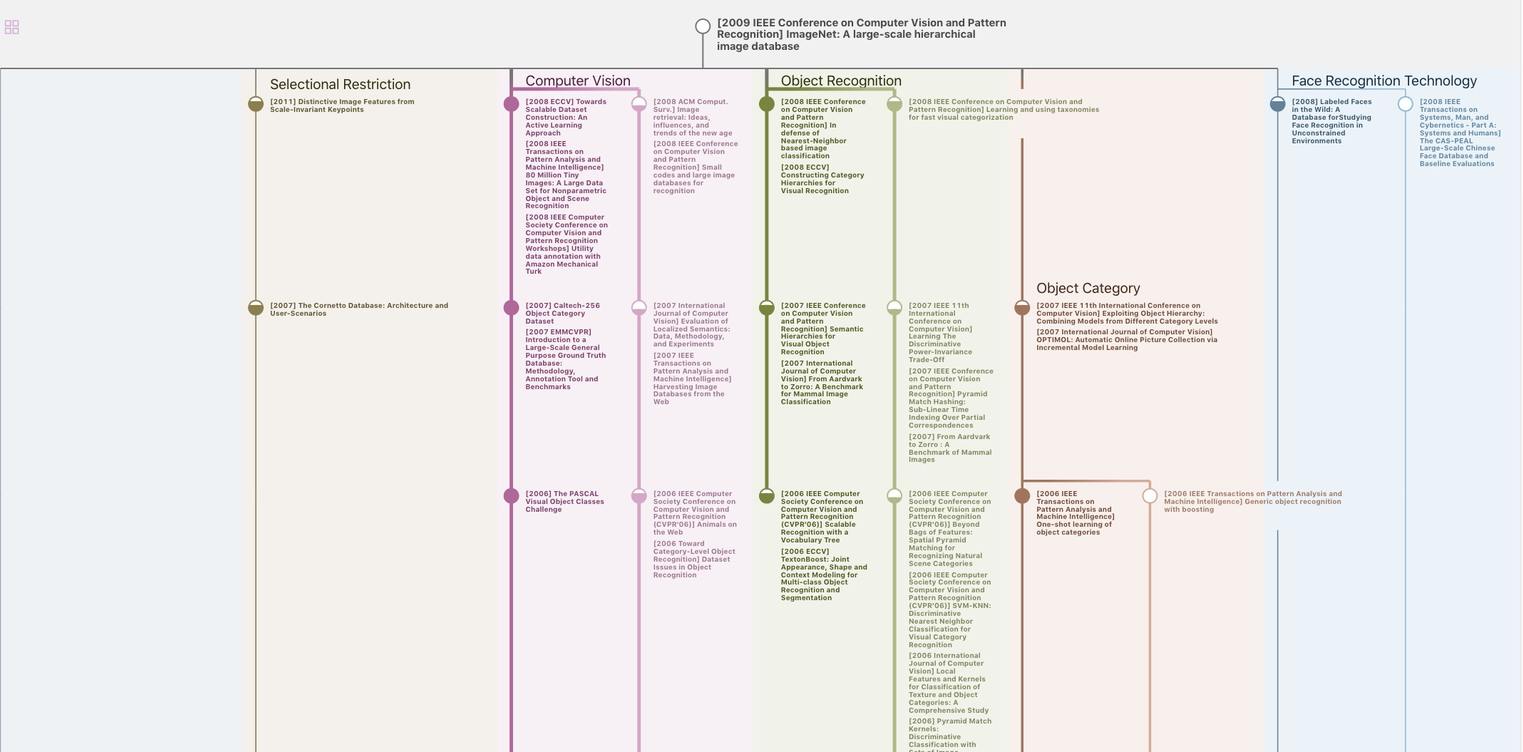
生成溯源树,研究论文发展脉络
Chat Paper
正在生成论文摘要