Speech based emotion recognition using spectral feature extraction and an ensemble of kNN classifiers
Chinese Spoken Language Processing(2014)
摘要
Security (and cyber security) is an important issue in existing and developing technology. It is imperative that cyber security go beyond password based systems to avoid criminal activities. A human biometric and emotion based recognition framework implemented in parallel can enable applications to access personal or public information securely. The focus of this paper is on the study of speech based emotion recognition using a pattern recognition paradigm with spectral feature extraction and an ensemble of k nearest neighbor (kNN) classifiers. The five spectral features are the linear predictive cepstrum (CEP), mel frequency cepstrum (MFCC), line spectral frequencies (LSF), adaptive component weighted cepstrum (ACW) and the post-filter cepstrum (PFL). The bagging algorithm is used to train the ensemble of kNNs. Fusion is implicitly accomplished by ensemble classification. The LDC emotional prosody speech database is used in all the experiments. Results show that the maximum gain in performance is achieved by using two kNNs as opposed to using a single kNN.
更多查看译文
关键词
emotion recognition,feature extraction,learning (artificial intelligence),security of data,signal classification,speech recognition,ACW feature,CEP feature,LDC emotional prosody speech database,LSF feature,MFCC feature,Mel frequency cepstrum,PFL feature,adaptive component weighted cepstrum,bagging algorithm,biometric based recognition framework,cyber security,emotion based recognition framework,k-nearest neighbor,kNN classifier ensemble,line spectral frequencies,linear predictive cepstrum,password based system,pattern recognition paradigm,personal information,post-filter cepstrum,public information,spectral feature extraction,speech based emotion recognition,cyber security,emotion recognition,ensemble kNN classifier,fusion,machine learning
AI 理解论文
溯源树
样例
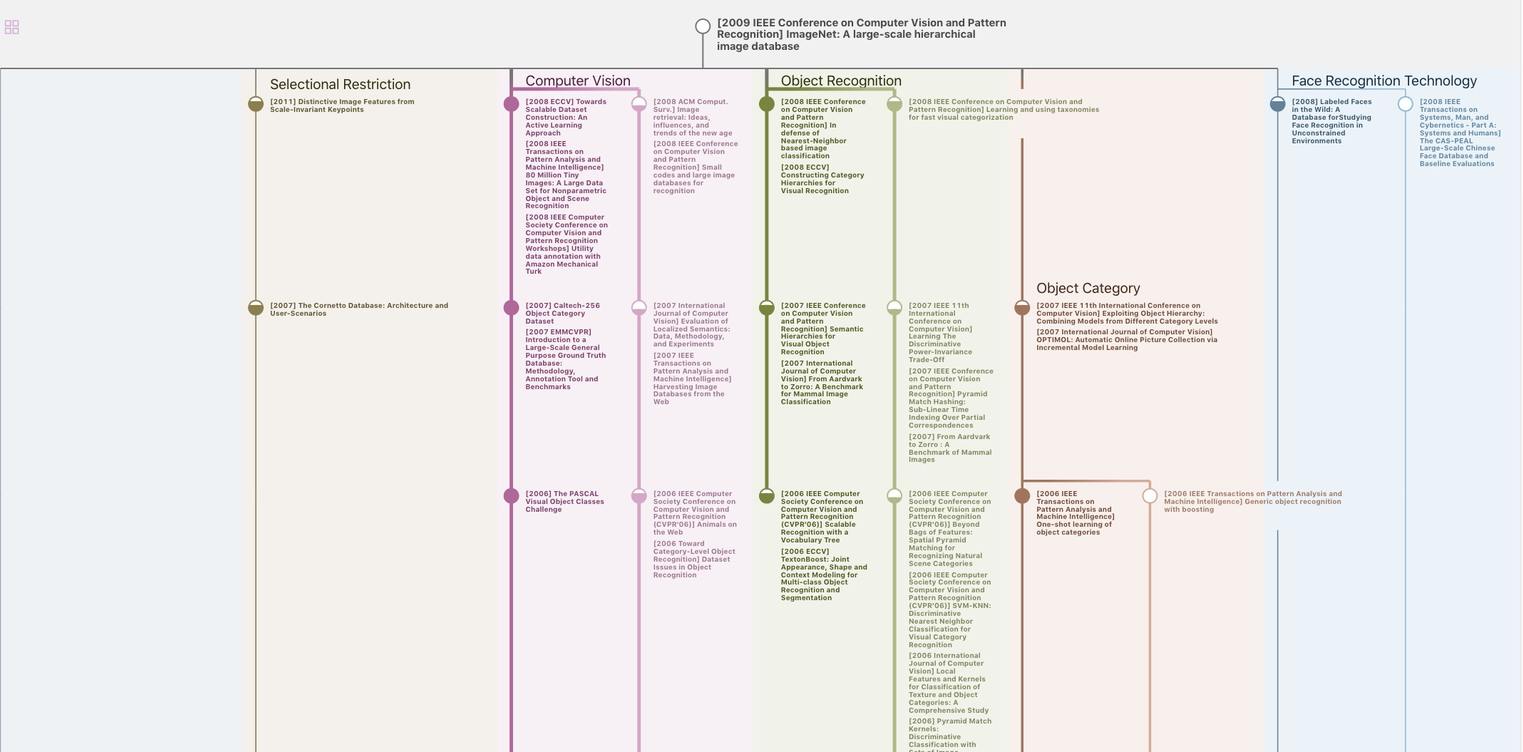
生成溯源树,研究论文发展脉络
Chat Paper
正在生成论文摘要