Decentralized compressive sensing.
Communication, Control, and Computing(2010)
摘要
Motivated by sensor network applications, in this paper we study the problem of a decentralized network of J sensors, in which each sensor observes either all or some components of an underlying sparse signal ensemble. Sensors operate with no collaboration with each other or the fusion center. Each sensor transmits a subset of its linear measurements to the fusion center. The fusion center gathers the data sent by all sensors and reconstructs the signal. The goal is to compress data at each node efficiently for accurate reconstruction at the fusion center. Accurate reconstruction is possible only if sufficient, well-chosen measurements are provided at the fusion center. In a decentralized network, each sensor measures part of a sparse signal. We refer to the sparsity of the observed signal at each node as local sparsity. Although the original signal is sparse, there is no guarantee on local sparsity at each node. To manage decentralized reconstruction, we propose a new Bernoulli Sampling scheme. This scheme associates an independent Bernoulli trial, with parameter p, to each measurement that a sensor makes. The sensor makes a measurement, if the outcome of the associated Bernoulli trial is 1. The measurement is ignored otherwise. We apply this sampling scheme to different sparsity models, including a common signal model, a common signal with innovation model, and a partitioned signal model for the observed signal. We show that it is possible to accurately reconstruct the signal through Bernoulli sampled measurements, with no assumption on the local sparsity, if the success probability of the Bernoulli sampling exceeds a lower bound. We also show the recovery through Bernoulli sampling is robust to noisy measurements and packet loss. If the signal is of length N, with sparsity k, the lower bound we derived for the parameter p of the Bernoulli Sampling, for robust and accurate reconstruction, is O(k/N log (N/k)). This result implies that the expected number of measure- - ments needed for stable and accurate reconstruction is O(k log (N/k)). This is the same as the result obtained for a collaborating sensor network or a distributed network with local sparsity assumption.
更多查看译文
关键词
data compression,signal reconstruction,signal sampling,bernoulli sampling,collaborating sensor network,decentralized compressive sensing,distributed network,fusion center,linear measurements,sparse signal,random variables,minimization,lower bound,sensor network,length measurement,sparse matrices,packet loss,correlation,compressed sensing
AI 理解论文
溯源树
样例
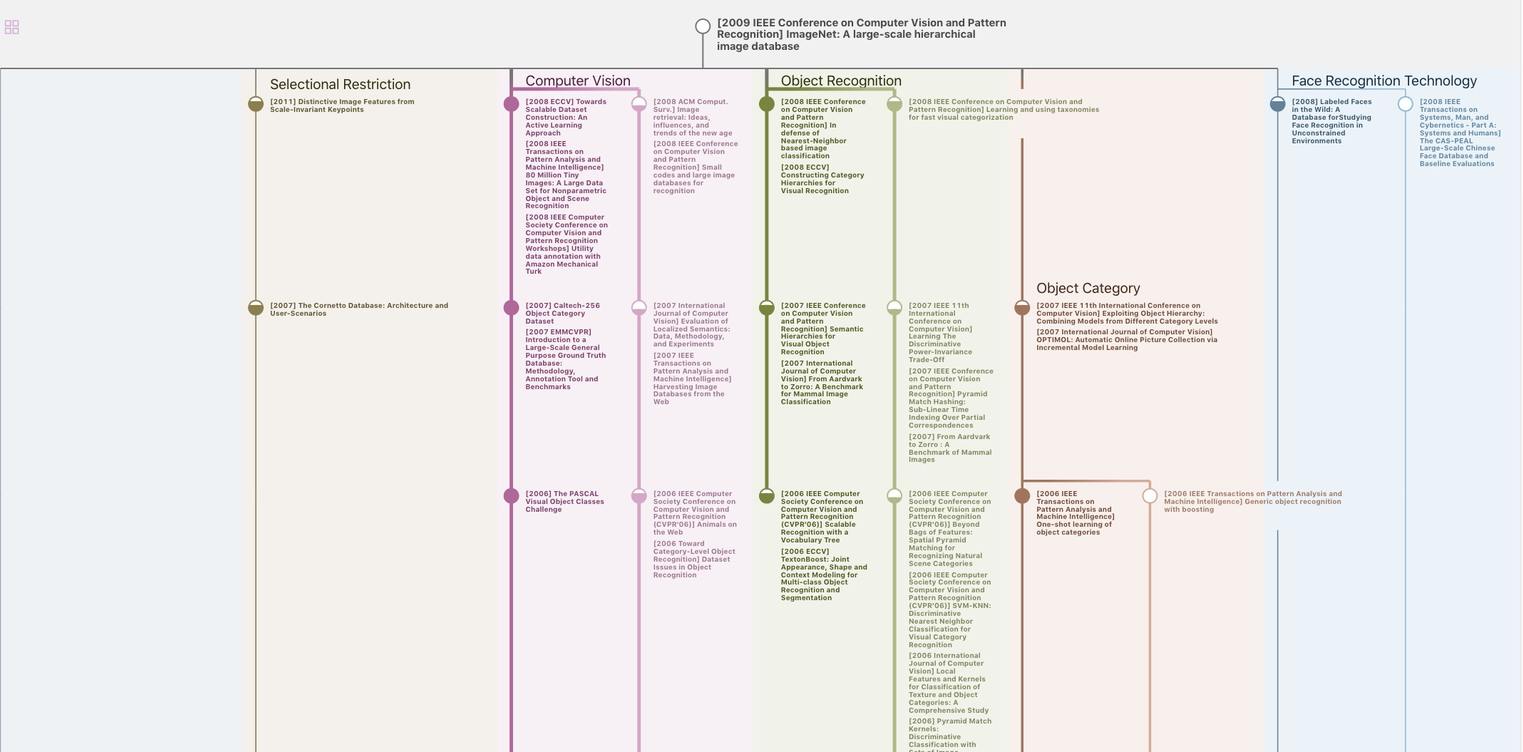
生成溯源树,研究论文发展脉络
Chat Paper
正在生成论文摘要